Tim Gasper [00:00:06] Hello everyone. Welcome. It's time once again for Catalog and Cocktails. It's your honest no BS, non- salesy conversation about enterprise data management with tasty beverages in hand. I'm Tim Gasper, longtime data nerd, product guy, customer guy at Data. world. Joined by co- host Juan Sequeda. Juan-
Juan Sequeda [00:00:23] Hey Tim.
Tim Gasper [00:00:23] ...where are you?
Juan Sequeda [00:00:24] How are you doing, Tim? I'm Juan Sequeda, principal scientist at Data. world, and as always, it's a pleasure. And today I am lucky to be in person with our guest. I'm actually on the other side of the pond. I'm in Amsterdam today and I'm super excited to be able to chat today with Peter Kromhout and Miriam Halfiede from Exibia that we have been just working. It's just really cool to get so many people, like- minded talk about AI and becoming AI ready, which we're going to chat about. But Peter, Miriam, how are you guys doing?
Peter Kromhout [00:00:56] Doing great, thanks. Pleasure having you here.
Juan Sequeda [00:00:58] Well, to kick it off, we always do our tell and toast. So what are we drinking and what are we toasting for today? Tim, how about you? You- inaudible
Tim Gasper [00:01:04] You want to start with me because mine's going to be the most boring. It's 8: 00 AM over here in Austin, Texas, and I've got my delicious home coffee that I make. So what about you guys? You probably have some more interesting things you're drinking?
Peter Kromhout [00:01:19] inaudible ice coffee for you.
Miriam Halfiede [00:01:20] I'm afraid I'm beating you to it with the most boring drink, which mine is just water. Okay, carbohydrated water, but still.
Peter Kromhout [00:01:27] All right, well then-
Juan Sequeda [00:01:29] Tim, Peter, we have actually a very nice one.
Peter Kromhout [00:01:32] It's a non- alcoholic Belgian beer called Leffe.
Juan Sequeda [00:01:37] I'm happily surprised. I didn't know that you did an non- alcoholic one. This is actually super tasty.
Tim Gasper [00:01:42] I like Leffe. I didn't know that they had a non- alcoholic version.
Peter Kromhout [00:01:46] We have lots of non- alcoholic beers nowadays here in the Netherlands that are really good. And I still need to drive, so it's a good thing to have non- alcoholic beers, I would say. Yeah.
Juan Sequeda [00:01:56] Yeah. Well, and I think let's toast for being here in person always. It's just great to go chat and have conversations about AI, so cheers.
Peter Kromhout [00:02:04] Cheers.
Juan Sequeda [00:02:05] You've been missed. We miss you, Tim. All right, well let's just kick it off. All right, honest, no, BS. What do we mean by being AI- ready? I think it's a phrase, a term people are throwing out there, but what does that mean for you all?
Miriam Halfiede [00:02:19] To me it depends a bit, what do you want to do with AI because it's not necessarily the goal in itself, at least I would say, I would argue it shouldn't be. It's a means to an end. And then means to an end, well, what's your goal as an organization in general?
Peter Kromhout [00:02:38] So it's about answering business questions and using AI-
Miriam Halfiede [00:02:42] To support that.
Peter Kromhout [00:02:43] Yeah. In the traditional sense of AI, so machine learning, optimization models, classification models, but also the new-
Miriam Halfiede [00:02:51] Yeah, I think with Gen AI it could be even more like what would be the purpose? Is it to increase your productivity or is it to support your mission or whatever that might be, but it's more about that purpose and AI supporting that purpose as means to an end rather than just by itself.
Juan Sequeda [00:03:08] So I think this is one of the stuff that we need to start becoming a little bit more philosophical, but it's valuable to be philosophical around the stuff because what is the purpose? And one of the things that we're seeing all the time is that with AI, the big values that we're seeing with Gen AI language models is productivity. So that's a productivity gain. So what does it mean to be AI ready to be productive versus, oh, I want to be able to go say, answer business questions. Well, you can see that through a lens of, well, I'm being more productive because I can answer more questions, but also it can be I'm answering questions I haven't even been able to do before. So maybe in that case it's not even just, it's a type of productivity, but I'm opening new opportunities that have never been exposed all the way to new revenue opportunities we're doing. So given these different types of ways of viewing what AI can be used, how would we then actually get more tactical, more specific, what does it mean to be AI ready then?
Peter Kromhout [00:04:07] So what I think is that, and we're seeing that as a movement here, is that we recognize that the garbage in garbage out still holds true today. So if we in the traditional sense of AI, have machine learning models or that kind of models and we just feed it data that is of low quality, it's going to give you output that is of low quality and that means you cannot properly answer these business questions, whatever driver inaudible. So we are seeing that as a movement here, that there's more attention to make sure that whatever goes in is of high quality and is usable so that the output of your AI is good. And I do think that would also be applicable to the Gen AI phase that we're now in, of course. You'll probably have the same there, which is a little bit different with hallucinations and all, but still you need to have your foundations in order to have your AI working properly.
Tim Gasper [00:05:06] So this starts to get into what are the activities that you need to do in order to become AI ready? So it sounds like having that good foundation around data quality, data management ends up being one really key thing.
Miriam Halfiede [00:05:22] Yeah. And that's maybe more of even on the, let's say, actual indeed execution side of things that you need to have a good foundation. But it starts of course, with strategy. Sometimes we struggle even there, but essentially, and I would say also having a set of use cases that are clearly scoped, it's a good place to start.
Juan Sequeda [00:05:44] So it's interesting how we now see these two paths around it. One, we can talk about frankly the technical side. It's like, okay, you need foundations, you need to have the data quality to avoid garbage in garbage out. And the second one is going to be around on the strategy and the use cases. I think the purpose will be associated to that. So let's dive a bit more into that. I mean, you want to take it first with on the strategy side, what do we need to be ready from a strategy perspective?
Miriam Halfiede [00:06:14] I think first of all, it's good to know what do you want as an organization? Where do you want to be? I mean, essentially it boils down to where do you want to be? Where are you at now and how do you come from point A to point B? But these get quite philosophical as well. It's a bit more high level. What do you want to be? What are your desire as an organization? Can have so many ways and to make it more concrete. And then how can analytics, data, AI, all of the above, help you with that?
Peter Kromhout [00:06:49] So from your opinion, do you see that a lot of the companies that we help, do they have a clear strategic vision on these things or is there a lot to gain there still?
Miriam Halfiede [00:07:02] I develop data strategies. So usually when company approach me, they don't have a clear strategic vision in terms of data. But they might have a business strategy and then it's our job to link one into another so that the business strategy is actually supported by the data strategy.
Juan Sequeda [00:07:17] So we're seeing data strategy and now AI strategies, and then you have your business strategy. How are all these things related? I'd love to go... Can you give some hypotheticals, some example?
Miriam Halfiede [00:07:33] I think the data strategy or AI strategy a bit dependent on the maturity of the organization, indeed. Does it have the foundation to actually start doing things with AI? Then it's most probably AI strategy. If they still need to work on that foundation including data management, then it's still a data strategy.
Juan Sequeda [00:07:59] That's on the strategy side, we'll get back to it more. Now from the foundational technical side, how is this going to be... Let's unpack that more and then I want to connect these two things with a strategy.
Peter Kromhout [00:08:12] So where do we start? Where do you want to start?
Juan Sequeda [00:08:14] I don't know.
Peter Kromhout [00:08:14] This is a big topic. So getting your foundations right starts with, okay, so we want to do something with data management. So we are trying to answer a business question and instead of just flying in and trying to get the data and build a report or build a model, we take a step back and think about, what do we need to have in place to do that correctly? And then a lot of these kind of data management concepts come in like, what source am I using for it? Is this the correct source? Am I getting the data there correctly? What does the data mean that we're fetching? Data's being created in a real world situation, how does that translate to data that's being stored in the database somewhere? And how do we capture that relationship? What do we do with data quality and how to deal with bad quality? All these things that you should incorporate in the way that you're answering that business question, is going to help you build that foundation. Or how do you approach that? Is that a bottom- up thing? Is that a top- down thing? I've seen both approaches in different organizations and well, some aspects of them are successful and some aspects of them are clearly not. But I think that's what you want to do, To get the foundation.
Tim Gasper [00:09:36] Do you find that companies often have that foundation that they need in order to start tackling these AI initiatives and questions? Or would you say by and large that it's missing?
Peter Kromhout [00:09:50] Yeah, I would say by and large that it is missing. I think a big part of that might be, well, I think a big part of it is that we have moved into a new paradigm like 5, 7, 8 years ago where we said we're going to stop doing traditional data warehousing. We're going to do data lakes, ELT. Not doing ETL, we do ELT. And I think with that paradigm shift, we've lost a lot of structure and, well, foundational knowledge that we used to capture in this old paradigm. And we don't have that anymore. And I think a lot of the companies are now running into the fact that they have a beautiful data lake. They've sunken a lot of millions of euros or dollars into that, and it's still not answering the business questions that they want to have answered. And I'm not advocating that we go back to on-premise, ETL like in the old days, but I am advocating that we bring some of that structure and way of working back into the modern time that we are in.
Tim Gasper [00:10:54] Yeah, I know some people talk about the lake house and maybe we have a beautiful lake, but we haven't done enough to build the house yet.
Juan Sequeda [00:11:04] So I'm speechless of these things. You said it yourself, we've been doing this stuff over and over again and changing the technologies. So it seems like it's just, we lack completely at the strategy. I mean, we love to go through technology, the problem, but why is it that we are so much struggling then on this strategy? Because I mean, if you did have a strong strategy around all this stuff, you wouldn't be doing it over and over again and then repeating and reinventing the wheel. Why is this?
Miriam Halfiede [00:11:38] Why the strategy is absolutely needed, you mean?
Juan Sequeda [00:11:41] No, why aren't we actually using... We don't have a strategy defined.
Tim Gasper [00:11:45] Why are we so bad at this?
Juan Sequeda [00:11:47] Why do we suck at this, basically? I'll be very, very blunt and honest here, we suck. We are doing this. We keep solving this problem, quote- unquote, " solving this problem" over and over again and it's still not solvable. And why is it? And it seems to me like where we have no strategy then. I mean, the strategy is what? Throw more technology? That's not...
Peter Kromhout [00:12:09] That's not going to-
Juan Sequeda [00:12:10] Well, that seems to be the strategy that has been working. I don't know.
Miriam Halfiede [00:12:14] It feels like a bit, it boils down to asking the tough questions that requires to be honest with yourself because you feel you get all of these questions and the same goes with ethics and you feel like, " Maybe not now I just want to use the tech. I don't want to think about it too much right now." And then you forget that it's going to come and bite you back.
Tim Gasper [00:12:35] You can't take shortcuts.
Miriam Halfiede [00:12:36] Yeah.
Peter Kromhout [00:12:38] So shortcuts is a thing here, and I think it's a people problem. Sorry, people that I'm not here to offend you, but it's not a technology problem, it's a people problem. It's data analysts, data engineers, data scientists needing to solve a business question, but not stepping over the boundaries and not getting the support from business people, from SMEs to really understand what is the business problem, why am I using this data element? Is this really answering your question? It's about people that need to collaborate, that need to work together, but that are in different domains in an organization that are in different silos in organizations and those bridges, it's difficult for people to cross those bridges. It's much more easy to be in your daily meeting and say, " Well, I'm going to code this, I'm going to code that. I'm going to deploy it hopefully today or end of the week and then just hope that it answers the question." So I think that is a large part of the problem.
Juan Sequeda [00:13:36] So how do we build these bridges?
Miriam Halfiede [00:13:39] Well, it helps when you have people that can communicate on both sides of the equation. Maybe inaudible IT data and business, of course. Those are quite scarce, but that definitely helps. And sometimes a business person that is more savvy and interested in the tech or the other way around, those people really drive the collaboration in that sense. But at the end of the day, you're still dealing with people and it's still also part of the culture. And if it's not carried by upward management as well as by example, that it'll also not land at the end of the day. So it's not only top- down and it's not only bottom up, of course dependent organization, but you need both to meet in the middle.
Juan Sequeda [00:14:31] You say this, we need people who are very scarce, who are able to cross the bridges communicate. So are we then just doomed because there's not enough of these people? I mean, shouldn't part of the strategy here then be that we need to start investing and actually educating our workforce, our organization? We need to start investing and people can be these bridges. I mean, either within your organization. How about we need more boot camps on, not just coding boot camps, but people boot camps or... I mean honestly, because what are we going to do then? I think in five years, 10 years from now we have another technology, paradigm shift, which we all are already in one. So then another five, 10 years, we're going to have the exact same conversation.
Peter Kromhout [00:15:24] It would be nice to have you here in Amsterdam again, maybe I'll join you in Texas. So the team of today is crawling, walking, running, and it's like in real life when you have a kids, you need to teach them to start walking. You do that by holding their hand in the beginning and by doing it together. So I've seen different approaches, bottom- up, top- down. What I've seen work is really a use case driven approach. So not trying to cover the entire scope of your organization and be compliant everywhere and do data governance or data management everywhere, but start with a couple of use cases and do those use case in a collaborative manner. So make sure that you bring the different teams, the different participants from business data and IT together, and collaborate on that use case. And do it in such a way that you capture all that information and capture all that knowledge, such as the meanings, the relationships, the semantics, the quality expectations, etc. And that will help you in well, the output of your use case. And it will build that, " Well, I'm now learning how to walk, I'm now learning how to do data management," Together in a collaborative way. And from there you can skate it further. I think that's important.
Miriam Halfiede [00:16:46] On top of the use case driven approach, actually what we see as part of the use case driven approach is also the fact that this use case is aligned with the actual strategy and can generate measurable value so that you actually have the buy- in from the top, in that sense, so that it's not just a bottom- up use case that this needs to be connected and align. And you can also measure things like governance, in a way. You know how it was before, you implement the use case, you implement the governance around it and then you can also measure it, what it generates after.
Tim Gasper [00:17:24] It needs to be something that matters to the organization, but then connecting it to what you said, Peter, it still needs to be focused. You can't boil the ocean all at once. So can either of you give an example of where you think, whether it's one of your clients or just something you've seen in the industry where you think that this has gone well? Where they were able to do this crawl, walk, run, and what did that look like?
Juan Sequeda [00:17:52] I always like how Tim brings up more on the positive side. I'm always like, " Oh, we're doomed. We're screwed, five years." No, this can't be the case. You have to have successful. Thank you, Tim.
Tim Gasper [00:18:02] No worries, yeah.
Peter Kromhout [00:18:05] Yeah, so from a personal experience, I've just finished a project with an airliner and well, you've been involved in that sense there also, of course, and I think we've done that very successfully there. We were missing a little bit of the bottom- up support, but we took this use case driven approach. We partnered with a very important strategic program for this organization. So they said, " Well, we have a strategic program. There are a bunch of data use cases in that program. These are important for our future, and we do believe that we want to do data management properly for those use cases." " So we will commit to, well, every use case that we do that's data related, we are going to follow this data management framework that we have and we're going to do it properly." That is important because you need that support because it's going to take a little bit longer, let's be honest. You can cut less corners, you need to do a bit more work to do it properly, and there is always business pressure to get those models out there and to get those reports out there. But if you have this support, you have the wiggle room to take a little bit more time and do it of higher quality.
Juan Sequeda [00:19:23] So how do folks like that realize, oh, we need to follow? You said these data management frameworks, how did they come to the realization that it was valuable and necessary to invest more into this proper data management foundations? As you said, gives you wiggle room to be, " Okay, I'm going to take a little bit longer than you would expect because yeah, foundations are not as immediate."
Miriam Halfiede [00:19:52] Oftentimes in my cases, they already approach us but because they've been trying it without, and they've seen that it doesn't work and then they, okay, now maybe we should try something else, but curious to hear-
Tim Gasper [00:20:05] Sometimes you need to fail first.
Peter Kromhout [00:20:07] Yeah, so maybe fun anecdote. One of the things we did is, so you probably know DAMA or the big book, that's the standard for data management.
Tim Gasper [00:20:17] The data bible? inaudible
Peter Kromhout [00:20:20] Yeah, yeah. So to create this kind of awareness that people were not that mature, I summarized this book in 10 questions and I said, " If you as an organization are able to answer these 10 questions, then you're doing data management well." I can share those 10 questions, no problem.
Juan Sequeda [00:20:40] Can you do it now?
Peter Kromhout [00:20:40] It starts with what data do you have? What does it mean? Who owns it? Can I use it? Who is using it? Is there PII data in there? I think that's six out of 10. And the others...
Juan Sequeda [00:20:54] You're taking the notes here Tim? This is good.
Tim Gasper [00:20:56] I'll just get a few in here. But yeah, these are good.
Peter Kromhout [00:20:59] But this is not even the fun part of the anecdote. So I summarized the book in these 10 questions and then I had this all- staff meeting and I asked everybody to stand up, the entire audience, a 100 and plus people, and I said, " I'm going to ask you these 10 questions, and if your answer is yes, you're allowed to keep standing. If your answer is no, you need to sit down." It took me four questions and then only one person was still standing, the fourth of the 10 questions. And then everybody felt like, " Oh, we need to do something because we're not there yet." So this was one of the things that we did to create awareness.
Juan Sequeda [00:21:36] This is a fantastic example. I have never heard this before, something like this.
Tim Gasper [00:21:41] I like that you're actually simplifying it too, because the demo book, it's pretty intense reading, and if we can boil it down to 10 tenets of well- managed data, then that's a lot better.
Juan Sequeda [00:21:58] I'm already imagining, okay, I'm going to use this approach. And it's the whole thing, I like how everybody stand up and sit down and then people realize like, " Oh shit."
Peter Kromhout [00:22:09] You feel it here.
Juan Sequeda [00:22:10] Yeah. And then you're like, " This is not good." Curious, in this particular example, the folks in the audience, what were the backgrounds? Were they technical or the business-
Peter Kromhout [00:22:21] Yeah, this was more on the IT side of the organization. So this wasn't business.
Juan Sequeda [00:22:27] So what if the business, if you would've asked those questions to the business side, what are the 10 questions that you'd ask with respect to- inaudible
Tim Gasper [00:22:33] You ask them, what data do you have? And then they all sit down, right?
Miriam Halfiede [00:22:36] Yeah, it's also a bit of problem in the sense that data management is such a broad topic, especially for DAMA. There's so many aspects of it that everybody refers to maybe slightly a different thing when they name data management. So it's first also having this common understanding. Do we mean the same thing when we say data management or data governance? Because yeah...
Peter Kromhout [00:22:59] It's very broad.
Miriam Halfiede [00:22:59] Sometimes it means something else for everyone else to say whether it's business or IT for sure.
Peter Kromhout [00:23:06] And I would say towards the business, I've used storytelling a lot. It's about perception. So in my first phase there, I came across a couple of situations where I could clearly see that lacking data management led to problems. So dashboards breaking, models breaking because of certain, well upstream choices that were made in the past. And if you can simplify, well, this is what happened to your dashboard, which is critical for you, but the causes are this and this and this, and that means you lacked on data management. That's making it more tangible. That helped a lot also to get by.
Juan Sequeda [00:23:44] It reminds me, Tim, of the episode we had on data storytelling with Cat, I forget her last name, going through the and or the and, but therefore. So it's and this has happened... But, and then at the end, we have to do something, therefore we need to go do this. That's the-
Tim Gasper [00:24:04] The structure of the story.
Juan Sequeda [00:24:06] Approach inaudible story should be telling. And I think-
Tim Gasper [00:24:08] I also think of our episode with RuPaul that we had a while ago where we talked a lot about the importance of examples and anecdotes.
Juan Sequeda [00:24:17] I think this example anecdotes is really critical. And I think people, they live through these issues and then they realize, " Okay, I don't want to go through it again," And then it just remind me, you have all this PTSD of all this stuff inaudible not going well I want to go fix it. Okay, so then tie the foundations back to the strategy. I'm just looking, I'm going back to I'm already imagining all these people sitting down or standing up, sitting down. Do they understand what is the strategy or how are they connecting then that the reason why they're sitting down is a problem and how that's going to affect the larger strategy? How do we connect these two dots? Because I feel that we have over time, it's like, again, the bridges are not built between the foundations of data and then the strategy.
Peter Kromhout [00:25:04] So I think the connection is, we understand now that we are in a need to be better at this, and then we chose strategic program with important use cases that will help the organization achieve their strategic goals. Then let's come in with a approach that we execute in those use cases and we help them to actually go from crawling to walking. We show them, " Okay, so if you want to do this properly, this is our framework. We're going to help you do the data modeling. We're going to help you do the data classification, tie the conceptual to the technical data, get the metadata up and running." So it's really holding their hands, executing these strategic use cases, and in that sense, maturing also the people that are part of it. And whilst you're doing that, you don't only do that with the analyst or the scientist, but you do that with the business SME, with the IT person, with the data engineer and data scientist together in a room workshop wise. And that's the way that you create these bridges because people are forced to sit together and talk to each other and express expectations and get assumptions out of the way. That's what happens when you put people in a room together and workshop on these topics.
Miriam Halfiede [00:26:24] And so I think one of the things that we also do is a use case ideation and refinement and validation. And in that process we gather a lot of use cases. Really let your creative method with the imagination go, but then how do you select the right ones? That process of actually selecting the use cases that directly visibly support your strategy. That is the main link, I would say. Yeah.
Juan Sequeda [00:26:51] At the end of the day, this is a people problem too.
Miriam Halfiede [00:26:54] For sure, yeah.
Juan Sequeda [00:26:55] We've been saying this is a people problem, and then we need to get people together. We can get them in the same room and let's stand up, sit down all this stuff and then send ideas. And then you'll realize if you start prioritizing, " Oh, I didn't know that was a priority for you, tell me more. Explain more of that stuff." It goes back to the storytelling too. This is all very important.
Miriam Halfiede [00:27:14] That's indeed, the core of my work, if you ask me what I do, then I would say, usually I gather people in the room that find different things important and then make sure that they have at least some type of shared vision together. That would be the core of my work. Yeah.
Juan Sequeda [00:27:30] So then, okay, looks like we started this whole conversation about what does it mean to be AI ready? And it's boiled down to be bold, you really need to be data ready.
Peter Kromhout [00:27:40] Yeah.
Juan Sequeda [00:27:41] Okay, so now let's jump ahead. Let's just say folks are data ready. Let's say people do have a strong data foundation. They have in their foundation, they have a strategy, their data foundations. There's connected to the data strategy, the business strategy. So now we have that. What's missing to get from there to be AI ready then?
Miriam Halfiede [00:28:07] I think it also, this... How do you call it, this? It's a bit of a shift in paradigm, because AI, it's a whole different thing if you consider generative AI- inaudible
Juan Sequeda [00:28:25] Well, I think that's the other thing to me, we've got to be, people are out throwing the word AI all over the place, but it means so many different things everywhere.
Miriam Halfiede [00:28:30] It's, you mentioned productivity, but it's not only productivity, it's also like this step- by- step tutoring in your pocket that everybody has access to. I believe someone called it proliferation, so that will have completely different effect.
Peter Kromhout [00:28:46] Maybe we should split the two. So first let's talk about traditional AI ready, and then we can go into the Gen AI ready.
Juan Sequeda [00:28:54] And I think this is important. I think we're all hesitating ourselves here too, because I'm thinking about this question too, and I'm like, " Okay, well, we're live brainstorming here too." And part of it's just to figure out, okay, you have the traditional AI, or we're not even just the machine learning AI approach. Now we have the generative AI.
Tim Gasper [00:29:14] Can we actually unpack that a little bit? When you guys think about traditional AI versus Gen AI, what are the differences? What do you think about those two being different?
Peter Kromhout [00:29:23] I think they're very different. So traditional AI building machine learning models has been around for quite a long time, and that is making sure that patterns are visible in the data and that you leverage those patterns to come to some kind of optimization or prediction. That is something that is different than the Gen AI where we really-
Miriam Halfiede [00:29:51] Generate new content.
Peter Kromhout [00:29:52] Yeah. Where it says it, right. It's generative, it's much more black box. We don't know exactly what's going on. We all know about the hallucinations. So that's a very different area of AI, I would say, or at least it requires something different from your data.
Juan Sequeda [00:30:07] And then-
Miriam Halfiede [00:30:09] And also from your people.
Juan Sequeda [00:30:10] Okay. Yeah. So let's break this down even further. From the people process technology side, you need different technology for these different things, but then also on the people and process side also. Yeah, let's break that down.
Peter Kromhout [00:30:25] So let's start with machine learning and what does it mean then to be ready? I think if we cross this step that we talked about earlier, having your foundations in place, making sure that you do your data management properly, then your machine learning project will go off much easier and quicker. You will be able to leverage, we know which data we have, we know what the semantics are behind it, we know how to interpret all these different elements. We are trusting that it comes from the correct sources. If we don't know exactly what things mean or we need to interpret something and it's not there, we know who to talk to because we have captured all that information. So it will help you speed up this machine learning use case tremendously. It will help you be able to... Well, you often need to develop features in your machine learning model, make it much easier to develop your features, especially if you have captured KPIs and how to calculate those. So I think that is what it means, being AI ready from a data perspective for-
Juan Sequeda [00:31:33] For the machine learning case.
Juan Sequeda [00:31:36] And
Juan Sequeda [00:31:36] then for the generative AI?
Peter Kromhout [00:31:38] For the generative AI-
Juan Sequeda [00:31:40] I mean, the same thing should apply too, right?
Miriam Halfiede [00:31:41] Yeah. On top of that, additional things apply, I would say. Right?
Peter Kromhout [00:31:46] Well, I think one of the very interesting promises here is that we can stop doing self- service analytics maybe in the future and really provide end users on the business, which is a different audience from what you just mentioned. It's a different audience. We can provide them maybe with an interface that they can ask questions and they will get proper answers instead of having to go to a data team to build a report or a dashboard. I think we can work differently in our industry. That is the promise of Gen AI on top of data.
Juan Sequeda [00:32:21] But then I still think that I acknowledge that there are these two different things we need to separate them, but I do wonder if each one will have different types of foundations, and I'm like, well, I think once you at the end, all these foundations are-
Miriam Halfiede [00:32:39] Building on top of each other.
Juan Sequeda [00:32:40] Yeah, they're building on top of each other. I don't think there's like, well, you need this specific foundation for the machine learning one, I think they're all... I mean, the other one, the topic is explainability and it comes into the part of ethics. And you need that for all of this type of AI. It's not just for one or the other.
Miriam Halfiede [00:32:55] It's true, but it's much more apparent with Gen AI because it shows when it goes rogue, so to say. It shows a bit the implications of it clearly shown, but it holds for other traditional ways as well-
Juan Sequeda [00:33:08] All the biases and stuff.
Miriam Halfiede [00:33:09] Yeah. But why I'm saying this, because for years I've been advocating for ethics and responsible use, but nobody would ever listen. Nobody cared until Gen AI came along and all of a sudden the momentum is there and everybody... Still some shifts that all of a sudden-
Juan Sequeda [00:33:26] These technology paradigm shifts actually helped to, I've been saying this for so long, finally, people are paying attention.
Peter Kromhout [00:33:32] Oh, that's so true actually, yeah.
Juan Sequeda [00:33:34] The same thing for me. I've been talking about knowledge now for so long and it's like, yes, this Gen AI, LLMs are finally good. I think the same thing- inaudible
Miriam Halfiede [00:33:42] Shows the potential of also negative implications and misuse as well as a potential for the positive implications. I mean, it's a two- sided coin or double- edged sword, however you want to call it inaudible
Tim Gasper [00:33:52] Just unpack one thing. I think it was you, Peter, that mentioned you're creating these experiences and applications that people can use with generative AI, and that makes me think that we're pushing data people to be a little uncomfortable and pushing them into a new arena. Because when you're just making machine learning models to optimize a particular pricing scheme or a business process, even though you need business understanding, the product often is just the model. But in the case of generative AI now, you're actually having to worry about how the business user is going to interact and what the user experience is, and it's almost forcing data people to become more like product people. And I'm curious if that's a problem that Miriam and you and Peter are saying, or if that changes part of what it means to become AI ready.
Miriam Halfiede [00:34:59] It could be in a sense. I've heard, someone mentioned this example, when you're in a shop and you have, I don't know, your child with you, and if you break something in a shop, then you break it, you buy it. And in a similar sense, maybe if Gen AI is used by someone to break something, then maybe, I don't know, the accountability should be at the vendor side who's making that? Is that the sense of the product, you mean? You break it, you buy it, or...
Tim Gasper [00:35:30] I think more from the perspective of data people having to take new things into account because now you can't just create a model and work with the data. You actually have to think about how end users are going to use it because there's an actual user experience involved.
Juan Sequeda [00:35:55] Well, and I think part of that user experience is this issue. You just mentioned accountability. Maybe that's something that, if that's a foundation and the data foundation, now you've got more pressure because now all the stuff that you're doing is being used for these other things. So where does that accountability go down? And it's like what inaudible You can make an argument. Like the building, something happened to the building. Well, it wasn't the wall, it wasn't the inaudible foundation.
Miriam Halfiede [00:36:24] Because you can use that same product with a very different intention. So you don't know what type of intention the user has. You mean that?
Juan Sequeda [00:36:33] Yeah, I mean that opens up an interesting question too, because you're like, well, you have so much responsibility saying, " I want this data to have well strong foundations because it's going to be used for this ML, this AI and this AI, this AI." And then you're like, I don't even know what you're going to use it for, and I don't even know what the use cases are. So this was built for some intention and later on you're going to go do it for something else and maybe it does work, but we didn't think about this other ethics part that we didn't know. So at the end of the day, you could argue that there's more and more pressure going to go on top of these foundations.
Peter Kromhout [00:37:09] Probably because the output is much more visual and if it goes wrong, the impact is bigger... Or well, maybe not the impact is bigger, I think machine learning models go in growth and have a very big impact also. But often coming back to what you were saying, Tim, it's more internal product. So there's a small user group internally. In a best case scenario, they use it automatically in some business process, but in many scenarios it goes to a person that does a sanity check before using the output of a model for decision making. In other cases, it goes to your website and it automatically does it. But that's not always the case. Many organizations are still in the stage where they say, " Well, give me the output of a machine learning model. I'll do a sanity check and then I'll use it in my decision making." And then the potential impact is much lower. You have a sanity check whilst if you go into this new Gen AI thing and it directly hits an end user, you have a bigger responsibility to bear there.
Miriam Halfiede [00:38:08] And especially because the end user usually is not as literate as the end user for ML model in general. And then there's also, for example, transparency. There's an additional layer added. With traditional models you just have transparency that can be on a different layers and levels, the transparency on outcome on the data itself or on the model. But now you also have a part, am I also transparent about the generated content that I use, for example, or not? There's another layer because yeah, you can actually copy paste something and reuse it.
Juan Sequeda [00:38:43] Oh, my brains started real-
Miriam Halfiede [00:38:47] Yeah, it's a big topic.
Juan Sequeda [00:38:48] Well, now realizing that when we talking about... We all started about getting into foundations and I'm like, wow, this foundations are getting more complicated. And then you want to have these strong pillars, but then you're like, " Well, I need to pack this thing to this thing so then we need more pillars or is that pillar need to get bigger?" And I'm like, well, I think the principles are getting more complicated here.
Tim Gasper [00:39:12] It's becoming harder to control all the variables. I think as data people, we've had more ability to compartmentalize. Even in this data lake world, we've been able to say, " Oh, well, all the bad stuff is in raw and silver and all the good stuff we're going to put in platinum. Okay, well, at least we're going to choose our zone and we're going to try to control it." But I think with generative AI now those boundaries are starting to become more fluid and it's becoming a lot harder to predict. To your point, Miriam, around the accountability, how it's going to be used and entering a brave new world.
Peter Kromhout [00:39:52] We should avoid that. This accountability comes back to the data engineers or the data team again. We've seen that with the data lake approach that they become the man in the middle and suddenly they are the ones to blame. Whilst maybe things go very wrong in this upstream situation, we should avoid that this happens again. And that again, the data people are the ones to blame when things go wrong with LLMs, et cetera.
Juan Sequeda [00:40:17] So what do we do then?
Miriam Halfiede [00:40:19] I believe honestly, that there is just like I mentioned right before, there is this race ongoing of deploying the latest capabilities as fast as possible in order to keep up with the race. The race of, if I don't do it that somebody else will. And I will say, I have a say in the future. I understand where that race is coming from, but there are incentives that are governing the dynamic of how companies deploy AI, that might not necessarily match public interest in that sense. And it's a difficult topic on its own.
Juan Sequeda [00:40:51] Yeah, this is, oh man. We should have another podcast episode just on incentives on managing-
Miriam Halfiede [00:40:57] Yeah, such a world on that. But yeah, to me it oftentimes boils down... There's not always right or wrong in this case. Sometimes it's a lot of gray areas and things are not defined yet. And for me, it's good enough if you just say that you actually made a conscious choice, that you actually thought about it. Just that by itself at least creating some awareness where to start. Because yeah, everybody has a different set of norms and values at the end anyway.
Juan Sequeda [00:41:31] Well, I knew this was going to go by so quickly. 30, 40 minutes, I told you we just got into the next topic or to the next hour, so we'll do that. All right, let's kick it off with our lightning round questions. I'll kick it off. Number one, do most companies realize they need to become AI ready or they don't even realize it yet?
Peter Kromhout [00:41:55] I think a lot of people still think this Gen AI thing is a magical solution and we don't need to invest or we don't need to do the legwork to reap the benefits. And I think it's up to us to educate these companies. You need to do the legwork.
Miriam Halfiede [00:42:11] Yeah, I think most of them do not realize what's actually behind it and what's needed for it to actually- inaudible
Juan Sequeda [00:42:19] So you can now send this podcast to those folks.
Peter Kromhout [00:42:22] Yeah, exactly. If there's one key takeaway, do the legwork, otherwise it's going to fail. Yeah.
Juan Sequeda [00:42:28] All right, you go Tim.
Tim Gasper [00:42:30] Second question. Should data strategy and AI strategy be combined?
Miriam Halfiede [00:42:38] If that's relevant, but usually, it can be both. I wouldn't say it should be combined. It depends a bit on what your goal is. No, not necessarily.
Tim Gasper [00:42:56] Okay. It depends on your goal, but not necessarily.
Miriam Halfiede [00:42:59] They don't necessarily need to be combined, but they do need to be aligned. Yeah.
Juan Sequeda [00:43:02] Yeah. A takeaway for me is whenever we're discussing these things, what is a purpose? Let's make sure we go back to, I don't know what the purpose is. If we don't know why the heck we're here, what's the purpose? Then we should sit down and figure that one out. All right, next question. Is there AI low- hanging fruit that doesn't require you to have a data foundation yet?
Peter Kromhout [00:43:25] That is a good question that you come up with.
Miriam Halfiede [00:43:30] I'm going to bring the ethics topic in general because ethics by itself does not maybe necessarily require the data from the foundation right away. But creating, identifying your organizational ethical compass is already helping to know what your value is. Also, organization and whether or not certain, let's say, choice that may have ethical implications desirable for you as an organization or not. That's more like a prep work, in a sense.
Peter Kromhout [00:44:02] Additional benefit to this is that you start that collaboration. You start bridging those silos.
Miriam Halfiede [00:44:07] And it's on a less technical level, so everybody can get philosophical on that.
Juan Sequeda [00:44:14] All right, Tim, take us away. Final question.
Tim Gasper [00:44:16] All right, final question. Are ethics and responsibility, a mandatory part of data and AI strategy?
Miriam Halfiede [00:44:25] Well, you're asking someone who's advocating for it. I wouldn't say it's mandatory part, but I would really advocate for it in the sense that, like I said, making the conscious choice. I would say it is mandatory to actually sit down and think about that. Whether or not you decide to go a route without ethics, that may be your own moral compass in a way, but at least you're making an aware choice.
Juan Sequeda [00:44:54] An honest, no BS position right there.
Peter Kromhout [00:44:56] But maybe it's mandatory because we're getting the EU AI Act. So we have the European AI Act in a draft state, and that enforces organizations to think about how to use and deploy AI. So you need to think about the ethics also there.
Tim Gasper [00:45:12] That's true.
Miriam Halfiede [00:45:14] From a personal point of view I would even say, yeah, don't do it because you have to do it because of the Act, but do it because it's the right thing to do. I really feel like we can wait for the government. It's going to take a long. AI, the first proposal came out in 2021 and it's still not accepted yet. So God knows when it will. And in the meanwhile we got Gen AI and all that. So I really feel that as professionals in the industry, we carry that responsibility to help... Interaction between AI and ethics is a two- way street, in the sense that yeah, how is this going to evolve? We have a say in it actually.
Juan Sequeda [00:45:52] Yeah, so I think, time to go to takeaways, but no, no, no. This is for me, my high level takeaway is one, yes to the legwork, foundation. Second, remember, what is your purpose? And third is, what's your moral compass? We should figure that one.
Tim Gasper [00:46:08] I think that's fair. I think that's fair. And the regulatory comment I think is an important one because there's what's ethical and there's what's legal and sometimes what's legal can help people to do what's ethical.
Miriam Halfiede [00:46:20] Those are not necessarily the same. It's true.
Juan Sequeda [00:46:23] Yeah.
Miriam Halfiede [00:46:23] And there's also, ethics is a product of society which can be seen as product of society and technology changes to society. And right now we're trying to fit everything in the current frameworks that we have, but ethics will be changed through technology as well. We can shape this, we have that influence as professionals.
Tim Gasper [00:46:42] We can shape it. And there's a feedback loop.
Miriam Halfiede [00:46:45] Yes, and I believe we should, but...
Juan Sequeda [00:46:47] All right, this is another podcast we need to go do. All right, kick us off. Take us away with takeaways.
Tim Gasper [00:46:54] All right, so we started off with what does it mean? Honest, no BS, to be AI ready? And you both really mentioned and especially you Miriam around it depends on what you want to accomplish, that it is a means to an end. And it all goes back to what's the goal of your organization? What are the business questions and use cases that you have, what are the metrics that you're trying to drive? Is it to increase productivity? Is it to increase efficiency? Is it to support your mission as an organization? So it really all does start with, so if you want to become AI ready, you got to have the strategy. You have to an underlying strategy. And if you haven't defined your business strategy, it's going to be really hard to then define your data strategy because you'll be doing it in a vacuum. You need to define your use cases around your strategy. What do you want to be as an organization? Where are you going? How do you want to get there? You need to connect your data strategy to that business strategy. You have to ask tough questions and you can't take shortcuts. You have to collaborate. This is a people problem, not a technology problem. You have to build those bridges and you have to cross them. You can't just code the thing and hope it will solve the problem. Garbage in and garbage out also is very much the issue. And generative AI and AI in general as it continues to become more predominant is going to exacerbate that and make that problem even more exposed, even worse. You need the foundation, data quality, data management. What source am I using? Is it the correct source? What does the data mean? How does the real life situation map to the data that we have? What is good quality? What is bad quality? So these are all really important foundations that we need to have the right people, process, technology and strategy to address. And we talked about how do you build those bridges? Well, scarce people and resources that can cross the bridge. How do we develop more of them? How do we cultivate and empower more of them? There's a crawl, walk, run. Peter, you mentioned you got to think about kids. You start by holding their hand. And so how do we handhold and go across this bridge here and get people used to it, doing it more often. Use case driven approach, very important. Bring it data analytics and business altogether. Learn how to do data management in a collaborative way around use cases tied to the strategy so you get that buy- in. Juan, what about you? What were your big takeaways?
Juan Sequeda [00:49:14] What I like is the takeaways I have here is, how do I actually take all this in practice and implement it to go with an example of folks you were working with, an airline. Is an organization where they've already partnered with a strategic program, they already have these data use cases. They've already realized, they know what's important for their future and they've come to the realization themselves that data management is important to have these strong foundations. Why? Because they've tried to go do this without foundations and they failed. I think that's an important thing. Sometimes when you fail, you learn that you need to do it differently and you need to have these strong foundations. And when you acknowledge that you need to have these foundations, you actually have the wiggle room to actually spend more time doing that. Because if you didn't, people are expecting things now, now, now. One of the things Peter was talking about, he has the bible, the DAMA, big book of data, summarize it in 10 questions. Wait, what data do you have? What does it mean? Who owns it? Does it have PII? So forth. And I love that whole anecdote of ask people these questions. They all stand up and if they can't say no, then they start sitting down and suddenly people realize, we don't have this foundation. We got to have to use storytelling, give examples and anecdotes and connect it to situations where you've actually had issues before that people can feel that pain. And just, again, we talked, this is a people problem. We get people in the room together. I mean, do use case ideations and then do the select priorities. And I mean having that conversation right there is how people start realizing it. Now, let's assume we already have this strong data foundation. What does it mean to get from data ready to AI ready? We had this discussion about separating two types of AI. You're building your traditional AI and building ML models and then now the generative AI. And I think there are foundations for both of these things. One of the stuff that comes up is that you have accountability, impacts, positive and negatives, and all these exacerbate and data teams are always at the center. This is something that we need to figure out, make sure it's not an issue over and over again. But there needs to be some shared accountability. And we're in the middle of this race and sometimes we just move so fast and we really need to sit down and understand what are the best interests for the broader stakeholders and at the end, for everybody around it. That was a lot. How did we do? What did we miss?
Peter Kromhout [00:51:25] Oh, there's lots to talk about, but this was a great conversation.
Juan Sequeda [00:51:30] Well, let's throw it back to you all to crack us up. So three questions. What's your advice? Who should invite next? And what resources do you follow?
Peter Kromhout [00:51:40] My advice would be, step over your boundaries and collaborate with other people, because I think that's where really the understanding and the knowledge is. So don't stay in your silo.
Miriam Halfiede [00:51:53] My advice would be don't be afraid to ask the tough questions and just be honest with yourself.
Juan Sequeda [00:52:00] Ask tough questions and honest, no BS.
Miriam Halfiede [00:52:04] Yeah.
Juan Sequeda [00:52:05] Who should we invite next?
Miriam Halfiede [00:52:07] Who should we invite next?
Juan Sequeda [00:52:09] Yes. To our podcast. Who should we...
Miriam Halfiede [00:52:11] Oh, I need to think about that.
Peter Kromhout [00:52:14] Well, there's this very interesting idea going on about doing less distributed. So this idea of we all need big distributed systems because we have this petabytes of data. A lot of companies actually don't. So we are seeing here a bit of a momentum around less distributed going like the DuckDB route. Talk about that next. That's interesting. Yeah, we should probably have somebody from DuckDB. There are challenges to tie it into data management, but that's up to you.
Juan Sequeda [00:52:44] Okay. Things like DuckDB and strong data foundations. I was like, oh, that's a good one. All right. Finally, what resources do you follow?
Peter Kromhout [00:52:55] Well, LinkedIn has become like Instagram. I'm sometimes scrolling through it, which is ridiculous of course, but it is. And sometimes you find very interesting articles and that link you into more and more information. So nowadays it's often a starting point for me to find more interesting stuff.
Juan Sequeda [00:53:13] LinkedIn is your new Instagram?
Peter Kromhout [00:53:13] Yeah.
Juan Sequeda [00:53:13] How about you?
Miriam Halfiede [00:53:15] On top of that, I closely follow Center for Humane Technology.
Juan Sequeda [00:53:20] Center for Humane Technology.
Miriam Halfiede [00:53:22] I think it's, yeah, really good content there.
Juan Sequeda [00:53:27] All right, with that, thank you all very much. We appreciate it and have a great rest of your day inaudible
Tim Gasper [00:53:33] Thank you so much and glad to have you all.
Peter Kromhout [00:53:34] Bye.
Miriam Halfiede [00:53:34] Bye bye.
Peter Kromhout [00:53:34] Bye-Bye.
Special guests
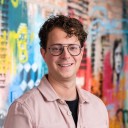
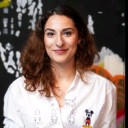