Tim Gasper [00:00:00] Welcome once again, it's time for Catalog& Cocktails presented by Data. world. I'm sure many of you are longtime listeners, we're excited here to be both in Austin as well as somebody somewhere else, you'll find out in a second. This is the honest, no BS chat about data. I'm Tim Gasper, longtime data nerd, product guy, customer guy, joined by co- host, Juan Sequeda. Hey, Juan.
Juan Sequeda [00:00:20] Hey, Tim. I'm Juan Sequeda, principal scientist at Data. world and as always, it is a pleasure to spend the middle of the week, Wednesday, end of the day, towards end of the day or really end of the day where we are and just spend the time and chat data. And if you see the background, we're in this weird place. We're actually in a castle in the middle of nowhere Germany and I'm super excited to be with Paco Nathan, which Paco Nathan, evil mad scientist.
Tim Gasper [00:00:46] Yeah, perfect.
Juan Sequeda [00:00:47] Evil mad scientist, Paco Nathan. And if you don't know who Paco Nathan is, just look him up, because you're little busy now. And we owe a lot to the stuff that Paco's been doing. Anyways, Paco, how are you doing? It's great to finally have you here in the podcast.
Paco Nathan [00:00:58] It's great. Juan, Tim, thank you very much. I'm really looking forward to this. Also just, we're having a fantastic time here at Dagstuhl. This is great. This is my first time here.
Juan Sequeda [00:01:07] So let's kick it off with what are we drinking and what are we toasting for?
Paco Nathan [00:01:10] Okay, so this is Serrat, this actually comes from a part of Italy one of our colleagues is from. And it's a little fruity, but it's got some legs to it. And if you let it open up, it'll do it.
Juan Sequeda [00:01:21] Yeah, this is susumaniello is a grape that we're having right now. And we are in a place called Dagstuhl, which is a castle, probably like three hours south from Frankfurt. And we're here just to get ourselves immersed, a group of 40 people to talk about the future of knowledge graphs. And that's what we're doing here and we have a lot to talk about today. Not just about knowledge graph, but also about just research and innovation and open- source and so forth. Tim, how about you?
Tim Gasper [00:01:55] Hey, I am drinking something a little less classy. It is a Dale's Pale Ale, very tasty and light pale ale. Very good. And I'm actually hanging out in Austin, Texas. I have strong FOMO for the event. I know, Juan, you go there quite often. Very cool event. But I'm here at the Capital Factory in Austin, Texas. We're doing our sales kickoff. So it's been a great day, a very energizing kickoff to the year. And cheers to being able to hang out with great people in person. It's truly a wonderful experience.
Juan Sequeda [00:02:26] Cheers to that, cheers to that.
Tim Gasper [00:02:28] Cheers.
Paco Nathan [00:02:28] Is Dale's local from Austin? Is that a microbrew there?
Tim Gasper [00:02:33] I forget if it's based in Austin or not. But I know that they have a pretty strong presence here and a lot of people like their beer around here.
Juan Sequeda [00:02:39] Oh cool. I lived in Austin for almost 20 years. My spouse is from there, but I've lost track of the microbreweries, so inaudible. So warmup question, we should also toast that it's your birthday.
Tim Gasper [00:02:55] Yes.
Juan Sequeda [00:02:57] Happy birthday.
Paco Nathan [00:02:58] Thank you, thank you very much.
Tim Gasper [00:02:58] Happy birthday.
Juan Sequeda [00:03:00] So inaudible question, what's your favorite birthday celebration you ever had?
Paco Nathan [00:03:03] Cool. Well I wanted to do something special when I turned 50. This was actually a while ago. But I wanted to do something special. So I invited friends and family from around the world and we set up. I was born down on the Central Coast of California. So right around where my parents lived at the time there's this great state park, it's called Montaña de Oro and it's got these amazing sand dunes and tide pools and everything, and some pretty good surfing too. So I invited friends over and we just had this party way out in the middle of nowhere, out in the sand dunes. And I figured that was the best way I could come up with it of celebrating my 50th.
Juan Sequeda [00:03:38] How about you, Tim? What's your favorite birthday celebration?
Tim Gasper [00:03:41] That sounds awesome. That's a great question. Probably about six or seven years ago there was a surprise party that my wife threw for me and I totally got caught off guard. That was a lot of fun. You know when you have no idea? That's kind of fun.
Juan Sequeda [00:03:59] No. So mine is for my 30th, which happened right at the end of one of the main conferences that I go to, the Semantic Web Knowledge Graph Conference every year. And this has been my community, which a lot of the folks are here right now this week and they're my friends. And so the conference was near New York, so we just rented a house in New York and I think like 20 people came over after the conference and we just hung out and had so much fun in New York City, that was a great 30th birthday. I'll never forget that. So anyways, well let's kick it off. All right, honest, no BS. What's the state of just bringing innovation from research and bringing it, translate it into the real world and how does open- source play the role in all of this stuff? Because I know that's a lot, what's on your mind?
Paco Nathan [00:04:46] Yeah, that's definitely that's what's on my mind. That's what I try to do, what our team is doing, I have a lot of background working in open- source, so some projects way back in ancient history like Hadoop, but also like Spark. And I've done a few other things on some other projects, maybe not quite as big of changes, but definitely done a lot of work with Project Jupyter and a little bit with spaCy Pipelines and with Ray. And anyway, I think there's a lot of room for open- source machine learning in general. And right now, because we're seeing so much going on in AI, I think that definitely open- source models like you're seeing on the Hugging Face leader boards, it's really amazing. And I love that whole ecosystem. One of the things though is, Yann LeCun actually writes about this, that one of the things that's different about computer science, especially machine learning, is that years ago LeCun convinced the people at NeurIPS to get the papers released open, so they could do some data mining on it. And the publisher was like, " Yeah, we don't make any money off those anyway. Here you can have them, go ahead and publish them." And so NeurIPS turned its papers open and that's been a thing. Now we have of course Archive and others. But it's been a thing, especially in machine learning, where you get a lot of preprint and there's not these paid journals that are gatekeepers, that really do a lot of damage in some other fields. I'm not going to name any names. But computer science, machine learning especially, I think really benefits by having a much, much more open process. But preprints by nature aren't peer- reviewed. And so the flip side of that is you get a lot of stuff published and maybe the reproducibility rates on this are pretty low. So one of our customers does a lot of investment in AI research at top tier schools and they're very interested in the results that come out and open- source implementations of what comes out. How this can be brought to bear on industry use cases. But there's a long road between a project, a paper being published and something being deployed in a production cluster. And there's a lot of other really super amazing work that's going on right now, and there's a lot of great open- source. One of the things that I would throw out there, just for discussion, I had a little bit of a debate with a friend in DC recently who works at a large defense contracting firm and their view of the world is like researchers research, somebody writes a paper, they're done. They have no further involvement. And I was saying that really in machine learning right now, that is just not the case. The lines are totally blurred. People will do research projects, it will go out into GitHub or Hugging Face or wherever. A lot of people will start to use it. There's not this separation and throwing things over the wall from research to dev to commercial production. It's just all blurred. And if you want to be using really interesting stuff that probably the paper is only a few months old anyway. So I think that the model that a lot of people like in certain circles in DC are operating off of, I think that we've moved past that. I think the speed of what's going on in AI right now has just blasted past that. And DC is still struggling to try to catch up. In industry, if we want to be using really interesting natural language, machine learning, knowledge graphs, et cetera, I think that we need to depend on what's coming out of research, there's a lot of great open- source. And the impetus for this is that the reproducibility rates in machine learning are so low. I'm not going to harsh on people, but the rates of code being published along with the paper, that's already low. And then the rate of being able to install that code is fairly low. And having the code actually run without exceptions is low. And then having the sample applications reproduce what was published in the paper, that's almost non- existent. So the thing is that I think there needs to be more attention paid to this because again, these pipelines are collapsing. And I'm not going to harsh on someone who's getting a PhD, this is maybe their first time being lead author on a paper. You know what? They've made an accomplishment and more power to them. I'm not going to harsh on them. But somebody who's in a well- funded, top tier tech lab, they're publishing, they're saying, " Here's our code, here's what it does." And the code doesn't run. There's no excuse for that. And the same thing, somebody who's a computer science assistant professor, they know the game. If they're publishing stuff and it's not reproducible, I can see that there could be edge cases, but generally speaking, no excuse. So that's where I'm calling BS.
Juan Sequeda [00:09:40] Whoa, there's a lot here. And I think this is several points to unpack. One is it's interesting to go see how computer science has evolved. And I think I agree with you that today research papers come from machine learning AI, a lot of the stuff that comes from Europe and stuff, the people writing papers and generating code, and that's stuff. That you're like, " If you really want to go innovate, you need to start using that today because that's the latest stuff," right?
Paco Nathan [00:10:07] Yeah, right.
Juan Sequeda [00:10:08] And before there was all these gaps, it was like oh, you have to go all these steps and people would see it as there's a big separation I think now, I agree with you, that's blurred. And I love how you're calling out the BS here is like, well, okay, it's okay if for some cases the code may not be great either. But the majority of cases right now, especially if the big companies or the people who have a lot of experience are actually pushing this stuff out, they are just generating crappy code. Just very poor experience. So wait, why is that? Are people just out there to go just get their names out, get the fame out? Or are they like-
Tim Gasper [00:10:46] Yeah, why is this happening?
Paco Nathan [00:10:47] Yeah. Well, I think that probably the biggest, we have spent a lot of time looking through a lot, especially in LLM space, but also knowledge graph phase in general and natural language and some other adjacent areas where we have a lot of interest. We've spent a lot of time on our team looking through all of this that we could find that might be in category that might be useful and vetting it. And we've really, we've come up with a rubric which will be, it's in a paper that's in production right now or in draft right now. But we've come with a rubric to try to score when we encounter some code. Here's the checklist we want to go through to really see is this something we want to commit? Hey, if it's on GitHub, we can do PRs too. But is it really worth it to spend the time if it's not going to produce anything? Is it a dead end basically? And I think you can figure that out pretty quickly. And the thing that comes across most strongly to me is that so many people are chasing benchmarks. And so they go, they do their thing, they write some command line code that will show that benchmark. They publish the paper, " Here's our F1 scores and the table. Look, ours is bold in this one row." And that's their conclusion, done. The code is on GitHub, that it only does that one thing. And yeah, I get it. Okay, that's a way, that's like paper mills. That's a way to get your paper published, but it's not a way to do computer science.
Juan Sequeda [00:12:12] Okay, so this is interesting because I'll take some bait here about benchmarks because that was something that we did recently from our lab. So two things on benchmarks. So I think one of the things that happened with benchmarks is that it really drives we want to push the status quo and that's a way of measuring, have a way to go measure this. But the thing is that we get so obsessed with the benchmarks and then what happens is that the focus is not about actually solving the problem. The focus ends up on being how I can get better numbers on that benchmark. Now ideally you are accomplishing both, but then what happens is that you focus too much and then over- engineer for the benchmark. So I think that is one of the stuff that we need to call out. And I think that I'd argue that's just historical always in computer science. But one of the things that happens folks in practice in what I call "the real world", is you need to be critical. Don't just take the let's like, " Oh, we're going to go take a benchmark and look at the latest tool, the latest model," whatever it is. And that's it. " And we're just going to take the top one because that's the best one and go with it." No, people are over- engineering to that. And I agree with you, this is the stuff that I find extremely annoying. And just to, not in a salesy approach, as a kind of really research thing is what we did for people who have been following our work on our knowledge graph benchmark of large language models and knowledge graphs to do question answering over SQL enterprise, SQL databases was that. Is that you would see existing benchmarks, specifically text to SQL benchmarks and you would look at these numbers and it's like 98, 97% accuracy. People are like, " Oh wow, text to SQL is a solved problem," if you would look at that benchmark because you hit 97%-
Paco Nathan [00:14:08] For those cases, yeah.
Juan Sequeda [00:14:09] Yeah, exactly. So you've now focused so much on the benchmark and you focus your techniques to get better numbers on that benchmark. And we're like, " This cannot be true." And then when you look at the benchmark, it's like, " Oh, this is connect from the real world. This is not how these schemas are. These are not what..." So then that's why we decided to create another benchmark around that. And I know that what's going to happen is that people are going to go focus on those questions that we did on that scheme what we did. And honestly we're going to continue to work on the benchmark, but that should not be the focus of everybody's like, our goal here is now to improve this benchmark, improve our scores on the benchmark.
Paco Nathan [00:14:43] Well, it can be done better. I think case in point, okay, so there's a great paper about LLMs in knowledge graph construction Xin, if I'm pronouncing correctly, Xin Luna Dong, Luna Dong-
Juan Sequeda [00:14:57] Luna Dong, yeah. Luna Dong inaudible.
Paco Nathan [00:14:59] I think I've introduced her at a conference before. Luna Dong's paper quotes like NER that right now it's mediocre because it's running F1 scores between like 0.85 and 0. 95. But when you actually go out and look at what's available, there's Luke is like the state- of- the- art for research, but it really requires a lot of GPUs. It's running F1 scores on average of about 0. 95. But Tom Harrison, who, full disclosure, I've worked with him on a couple of open- source projects, he's now at Hugging Face, he's a fellow at Hugging Face. For his master's thesis he did something called SpanMarker and it runs on CPUs and it's running consistently, like average of the same benchmarks like 0. 95. But it's perfection quality software and it's using Hugging Face transformers to be able to download efficiently the ways and biases so you can pick the model and bring it down, whereas everything else is just a disaster in terms of loading models. But Tom really took the effort to make a general purpose research and production platform for any UR that is comparable with research state- of- the- art, but runs on CPUs and runs efficiently. And I look at that paper and it's like this is a master's thesis, why aren't the PhD papers this good? Clearly it can be done, it can be done in a short amount of time and it can be done well. And so I do think that there are counter examples for all the criticism I have. But on the other hand, I do see stuff that's just train wrecks and I think it's important to call out the people who are doing it well and give them support and integrate those projects and promote them and star them on GitHub and Hugging Face. But also reference them in your papers and your talks and whatnot and really call it out.
Tim Gasper [00:16:46] Yeah. What would your recommendations be to folks who are doing research, whether they're at large institutions or if they're in academia, to do better? You called out reproducibility, you called out focusing on maybe some of the wrong problem sets, like over focusing or over fixating on benchmarks and things like that. What are some recommendations you would give to the research community on how we can do better for everyone?
Paco Nathan [00:17:15] Well, I will paraphrase from Andrew Yang, but also just looking at what's been happening on the leaderboards for open- source LLMs on Hugging Face over the past two or three months. It's basically DPO, that's the message. And so the idea is that the data-
Juan Sequeda [00:17:31] Sorry, DPO?
Paco Nathan [00:17:32] Yeah, direct preference optimization, DPO, yeah.
Tim Gasper [00:17:34] As opposed to direct preference optimization.
Paco Nathan [00:17:37] Yeah, I hope I'm... It's out of Stanford, NLP. I know Chris Manning and Dan Jurafsky were some of the senior authors. I forget the lead author, but it's a beautiful paper. And Andrew Yang said that this is one of the only times in his life when he finished reading a paper and immediately wanted to get a standing ovation. It's that good. And seriously, go read it because this is how to publish research. But the thing is, the takeaway on DPO is we now have tools to go in and measure the data, measure the training sets, measure the benchmarks, measure the evals. And figure out where are the problem, how are those problematic? Before you spend all the money like blowing carbon in the atmosphere with GPU cluster. And when you look at what's been happening on Hugging Face leaderboards, the message is very clear. You take Argilla was one of the first ones, and full disclosure, I've been with Argilla for seven years, one of Oscar's students, inaudible-
Juan Sequeda [00:18:34] Oh, that's where... Oh, okay.
Paco Nathan [00:18:36] But also Snorkel followed up with the same thing. And Hugging Face has done its own, using DPO. And the message is this, take a model that's getting some real traction like Zephyr and go in and look at the data that's used to train it, look at the evals. And find out where the problems are there, fix them, get rid of the bad data that's working at cross purposes. And then DPO is a way of basically doing a synthetic, using human input, but then coming out with a synthetic dataset that's rebalanced. And so go in and retrain using the better data. And so I'm attacking the benchmark, saying that we have tools to be able to go in and figure out why they're bad. I'm not saying that every benchmark is bad, I'm saying actually, we could improve them. We have other tools as well. If you look at David Gleich at Purdue, I'm blanking again on the grad student who's lead author. If I had it in front of me, I could look it up. But their team came out with something that was a topological, topological data analysis on compute graphs. So when you're training models, you've got a compute graph. You can go in and do analysis to figure out what's going to work, what's not going to work before you do the training. And you can start to partition those data sets and figure out again, what to throw out, what needs to be augmented, what needs to be fixed. So there's a lot of tools that are coming out just to label GT, it's called graph topological data analysis, so GTDA and other kinds of quantitative tools. I think downstream, once you've got models, you can be using things like WeightWatcher. Are you familiar with Charles Martin?
Juan Sequeda [00:20:14] I'm not.
Paco Nathan [00:20:15] Yeah. So once you've trained a model, if you want to go in and understand what is really going on in a deep learning model, it's based on statistical mechanics and its analysis of deep learning models to really go in and find out like, okay, there are trouble spots here and there. There's stuff we really don't know what's going on over here, et cetera. But what I'm seeing is we have tooling, we have math to analyze the data, analyze the resolving models and do something smart rather than just saying, " Okay, here's a benchmark I have to beat." Why don't we go in and fix the benchmark too? That's what I'm saying. And I think DPO, what's been happening on the leaderboards over the past couple of months is showing that a team without GPUs can go back and take somebody else's training data, fix it, retrain the model and beat everybody else. And like I say, Argilla, Hugging Face, Snorkel, they've all done it. So I don't want to harsh on people again all that much, I appreciate all the research that's coming out. But I think that what Andrew Yang is saying there is we need a data first strategy, like fix your data first before you burn up all the carbon. Because otherwise, if you're working from really bad training sets or really bad evals, it doesn't matter how much algorithmic work or how much training time you put into it, you're going to come out with a crap.
Juan Sequeda [00:21:30] How well has this message been received? Because I'm hearing it loud and clear. And I think that's one of the stuff that we are super excited about, because Tim and I, we're data people, right?
Paco Nathan [00:21:43] Yeah.
Juan Sequeda [00:21:43] We're like yeah, inaudible. We're more data people than machine learning, AI people in this case, for creating models and stuff. And I take it to the next level as like Tim and I always argue is that we live in this data first world. I want it to be a knowledge first world. It's just not clean data, it needs to be data with the meaning, with the semantics of the stuff inaudible.
Paco Nathan [00:22:03] Yeah, yeah. We don't just drink the Kool- Aid, we're evangelists for this. This is the world we want.
Juan Sequeda [00:22:07] So do you feel that we are headed in this direction? Or is there still more-
Paco Nathan [00:22:13] I do, I do. I think that the results are out there in public and you're seeing really well- funded entities that are competing and you're seeing less funded entities that are beating them. It's market. You have so much capital.
Juan Sequeda [00:22:28] So this is then a call- out to, or now I kind of want to shift the conversation a little bit from research and bringing it to the real world enterprise is like, well, one thing is that you can start using all these models and stuff, but then the clear message is that you also need to start investing in having your data, knowing, cleaning your data, adding semantics to your data by focusing on a data first and a knowledge first approach.
Paco Nathan [00:22:52] Right, right, right. And I think there's a long road ahead on this. I don't think it's just a matter of, oh, we're going to clean up a couple of benchmarks that are popular. I think that this knowledge first approach, like you're saying, I think it's a very, very long road because this is the way we get past the hurdles we're facing right now.
Juan Sequeda [00:23:08] How do we shorten this? Because people are like, this is an argument. It's like, "Oh yeah, we get it, but it's so much investment and..." And then I'll throw money, I'll get some results and that's good. But then we focus on the immediate, but we know that in the long run, that's going to affect, so-
Paco Nathan [00:23:22] Yeah, it really shorten-
Juan Sequeda [00:23:23] ...how do we get to that knowledge first faster?
Paco Nathan [00:23:26] It kills me, it doesn't make headlines. Sam Altman can talk about I don't know, his race car and it gets headlines. But people actually come up with a better strategy that's more cost- effective and has better return on investment and there's no headline. That just kills me.
Juan Sequeda [00:23:43] Yeah, I'm pausing because I'm like I agree. And like where do we go from here?
Paco Nathan [00:23:50] Well, I think the thing is that we have something that works, drive it, make it work, prove it in the marketplace. I think this is a competitive advantage and it's time for startups or industry that's taking advantage of this kind of technology, take it and run with it and beat out the competition. It's time to get aggressive. Maybe that's the best way to... If they're not going to take the message, beat them in the marketplace and they'll probably listen eventually. Somebody will.
Tim Gasper [00:24:19] There you go. That's good advice. Paco, what do you see as the trends that are really impacting industry today, especially things that are coming from the research community. But curious in general, what do you see as the trends that are most impactful right now around this space?
Paco Nathan [00:24:39] Yeah. This is actually from a conversation Oscar and Paul started lunch today with us, because they were talking about their curriculums because they're cranking out master students in AI, things like that. And they're like, " What do we really need? What do you hire for that we're not providing?" And there's a few of us, and we all said the same thing at the same time. We're like ML ops. And they were like, " No, really what do you need?" It's like, " No, you need ML ops. You need people..." The thing is in corporate, HR usually sets what the hiring levels are, and anybody who's like ops, there's a cap on their salary. And it's ridiculous because Meta is going to offer them probably 50% or 100% or 200% more than where that cap is. And so corporates, the thing is you could have the best data in the world, the best ML engineers, the best knowledge graph representation for your business, and you could have killer apps. But if you don't have ops people to run it 24/ 7, you're dead in the water before you start. And the thing is that what I see is in corporates right now, in industry which have revenue bearing use cases, typically IT will take a look at what we're doing in knowledge graphs, whatever AI teams are doing and say, " Hey, look, this stuff is out of our scope. We're not responsible. If you want to run it, go run it. Hire your own ops people." And so I see a lot of projects getting starved because they can't hire triple shift ops staff to run the critical parts that they need. And it's not complete... ML ops is not easy, it's a hard job and it needs skills. But it's not rocket... It is rocket science, but it's not extreme rocket science. You can do this. The thing is it's really actually fun. But the idea of understanding what's going on in machine learning, what are the security consequences? What are the compliance and legal consequences? Because as ops people, you're there running it. You need to interface with the governance committees, you need interface as well with data science teams and apps people. So you really need to understand a lot of these perspectives in addition to observability and deployment and all the other stuff you would have. I think right now I'm really super interested in the communities of practice around ML ops because I've been involved in conferences a lot, industry conferences obviously through O'Reilly and others. And what I see right now is the ML ops communities of practice are really interesting nexus for bringing in a lot of different people. It's not just ops people there. It's like ML engineers, it's like researchers, there's product managers. There's just a whole range of different people who are there because they're like, " Oh, okay, this is important. This is interesting. I need to be there. Hey, maybe I'll hire some people." And I love going to those kinds of events. And so there's a few of them that I think are really good, especially that I could recommend and call out. But I've been trying to get involved in this kind of community because I see it as certainly a pain point for our biggest customers. But it's what we talked about at lunch today. It's like the thing that's missing from these AI masters programs. It's like, great, great. You're doing really well on the machine learning side of it. Do you know how to use GitHub even? Have you ever built a Docker container? Do you know what the word observability mean? Have you ever worked with Datadog? We can go down the list of maybe just some basic skills so that when you get the job, they can train you for the rest of it.
Tim Gasper [00:28:10] Do you feel like the area of ML ops that a lot of the best practices have been surfaced and it's a matter of just making sure that we're properly educating people, disseminating that? Or do you feel like there's a lot we're still figuring out as an industry?
Paco Nathan [00:28:29] It's pretty grassroots. To me, it feels like data science circa 2013, 2014, because we were just getting together and circulating best practices. Nobody had really even written the books yet. But there are some good talks that people could use as guideposts. But other than that, people go to meetups and trade ideas, and I think we're really at that stage right now. But the other side is that there are some natural guardrails to this because when you get into a corporation, how they handle their single sign- on, how they handle their role, space access control, these kind of concerns, it's pretty well documented. They have an opinionative way of doing it. So there's a lot of things that you'll learn on the job that probably won't be done anywhere else. But believe me, there are people there who know how to do it. So be ready to learn and understand these processes and where this stuff just doesn't fit, and how you have to be the glue. How do you deploy to the internal app store? Because that's where the users are. I could go on and on. I think that where we're at, this is actually something the breakout group that Oscar is leading. This is actually some of the stuff we've been talking about, is like how can we bring this kind of practice into software engineering for knowledge graphs and really start to articulate what are some best practices? So yeah, okay. This is what we've been talking about. I'm referencing.
Juan Sequeda [00:29:56] Well, just to keep folks more context, Paco and I are at this seminar here on where knowledge graphs are going in the future, and we've had all these breakout groups. And a report will be written, will publish soon about what we're doing. But it's fascinating, I don't know all the details of what you've been doing in your group because I've been on another group right now.
Paco Nathan [00:30:13] Your group is amazing. We need talk about your group too.
Juan Sequeda [00:30:17] Well, but this is fascinating because one thing that comes to mind right now is a lot of the organizations that we work with, they all are hungry to go do AI, but not everybody is mature and ready for it. And from one perspective, not even their data is ready for that, that's one thing. Second, you see a lot of people talking, now they see ChatGPT and they think it's magic. So they're like, " Oh, I want ChatGPT to talk to my data now. I'm expecting that type of stuff."
Paco Nathan [00:30:49] I need more wine.
Juan Sequeda [00:30:49] So okay, yes.
Paco Nathan [00:30:50] inaudible.
Juan Sequeda [00:30:49] So that's one thing. But then also the other aspect is you don't even have these infrastructures ready for this. So how much are we actually setting ourselves up for success or failure? Because there's all these foundational infrastructures, roles, but then the board is talking about, " We need AI," right?
Paco Nathan [00:31:15] Yeah, yeah.
Juan Sequeda [00:31:16] So how do we call BS on where we are today on the expectations of where we are and need to be and just lay out the reality?
Paco Nathan [00:31:25] Well, Ben Lorica and I do a lot of work together. You've been talking to Ben recently too. This is something we tried to take head on in about circa 2018. We did a series of mini books and industry surveys analysis, and what we found out is MIT Sloan and HBR and McKinsey Global and others were doing similar projects. So we just sort of summarized and coalesced it. And what it comes out to is you can think of a survival analysis because okay, here's our funnel. And at the top, the problem is there are executives who they don't want to hear about AI. Well, they do. They want to hear about ChatGPT, but as far as the application you need to deploy to calculate your supply network contingency, they don't want to hear about that. They just want ChatGPT. So up at the top, you've got executives who are probably pretty senior, and they probably grew up on things like Six Sigma. And people are saying that, " Hey, in production, we're using probabilistic kinds of methods for machine learning." They're like, " No, no, no, I don't want to hear that. I just want ChatGPT. And seriously, last year, from February 2023 to August 2023, around the world, a lot of top executives were playing with ChatGPT. It wasn't until Q4 started to loom that a lot of projects got turned back on because executives needed to file progress reports. So they really were playing with ChatGPT for six months or seven months. And drooling over the fact of maybe I could have a super intelligence and fire a third of my staff. And no, no, that's not going to happen. So when you go back to what we were looking at five, six years ago and all the analysis coming from a lot of high- powered sources, the story was this, at the top, there are executives who just don't want to hear it, they're disconnected. And then down below that, the next tranche of where you run into a survival hazard is that it's really hard for these companies to hire enough people who are basically product managers. They get the business, they understand the business, they can carry the ball through to make a business unit successful. But they also understand where the technology is, how that can be deployed, how they can really work with their engineers, with their data people, et cetera, to build the thing that needs to be done. And so hiring those kind of people, that product layer, is just difficult. There's just not enough of those people out there. And I'm hopeful that they will be there. I think that a lot of people are recognizing and growing into those kind of roles. But it's just really tough.
Juan Sequeda [00:33:54] I want to throw the ball here to Tim, because Tim is, as you say, is always a customer guy. But he's really a product guy at heart.
Paco Nathan [00:34:02] Yeah, awesome.
Juan Sequeda [00:34:02] Tim.
Tim Gasper [00:34:02] Yeah. No, that resonates a lot. Cheers. We see that everywhere. We see it in data, we see it in governance. Obviously it connects to AI and ML as well, somebody who both understands the business side of it and how to get things done as well as the technology. They can't be unversed in the technology or else they won't be able to navigate it. And then on top of that, they need to be somebody who can really work directly with the team. And so there doesn't seem to be enough of these people and they're hard, they're kind of more of that unicorn type person. There's not enough of them.
Paco Nathan [00:34:43] But hey, if you get the tech, but maybe you're working in, I don't know, a content marketing or some area that's in tech that you want to get a promotion, go out and work on that. Go back to school, get the product management jobs, work in some roles and take it on. Take it on head first, because it's a really great opportunity, I think.
Juan Sequeda [00:35:03] That's great advice right there. I think yeah, we need more product managers.
Paco Nathan [00:35:06] Yeah, get it.
Juan Sequeda [00:35:07] Get it. So I think another aspect kind of connecting to the previous conversation is on the ops side. So we also need folks who can do ML ops.
Paco Nathan [00:35:17] Yeah, that clearly, and this is probably part of the distortion of the big tech companies hiring like crazy, although of course that's backed off. But then also just corporates, HR is like 15 years out of date. So usually it's a matter of HR just doesn't really understand that this whole landscape has changed and here's a really competitive role that we absolutely need to staff yesterday. And no, we're not going to pay them$ 80,000 a year. That's the message that needs to come across and it needs executive air cover before that's going to change.
Juan Sequeda [00:35:53] So is it really something specific to ML ops or is it more a broader ops in general? Maybe I'm just by saying this, I'm like, maybe you're spreading yourself too thin, so that's why you need to specialize. But inaudible, we talk a lot about data ops, right?
Paco Nathan [00:36:09] Yeah.
Juan Sequeda [00:36:10] And ML ops, and at the end, if it's really a data first to knowledge first approach, that data is going to feed into not just those models, it's going to feed into also the dashboards, the reporting, whatever application. So it's like there should really be a whole strategy coming together. And I think, Tim, you and I have talked all about with our colleagues and executives we work with, is like, " What is your data strategy? What is your AI strategy?" These stuff that all go together and obviously connect inaudible. It should just be one strategy altogether.
Paco Nathan [00:36:40] Yeah.
Juan Sequeda [00:36:41] So what do you think? Is it really focused on being ML ops or is it more of a broader ops?
Paco Nathan [00:36:47] I'm almost thinking of ML ops, just like it's a label, it's a principal component. I can use that as a placeholder and access a lot of people in their focus right now. But two years from now, it might be a completely different label, but we know what it is. And what it's really called and really what the best practices are is not defined yet. We're in progress. So yeah, I agree. It's going to be much more of a data first, knowledge first thing. And the operational side of it is actually understanding what your business is. We were talking earlier that that was kind of like the role of the data scientist to be ethnographer for the business and understand what are the mechanisms and let's measure it. But no, it's actually operations is where you're doing the thing for the customer.
Juan Sequeda [00:37:35] So all right, because we're taking notes here. We have all our takeaways.
Paco Nathan [00:37:41] But I should say the other hazard that shows up that's really big is data availability and data quality. Because I think we both heard a talk, I'm not going to pick on anybody, but we heard a talk earlier today about how corporates have spent the past 10 years getting all of their data understood, and it's all carefully aligned in data lakes now. And you can just go get all of it. And it's like, no, you can't. No. There's mergers and acquisitions, and every time that happens it completely gets jumbled. So that is the other problem, is even in companies that invest in really big data lakes, and I could pick on a few, but I'm not going to, they probably own three or four of the major competitors in that space and they still can't get ahold of the data they need to do the work that we need to do with them.
Tim Gasper [00:38:25] It's a huge challenge. There's so many different data sources and data technologies and the rate of change is too quick and any company of significant size is really struggling to keep up. That kind of begs a larger question here of some of the companies that we talk to are trying to figure out the approach to really take advantage of ML and AI and they're trying to decide between a should I create a special island of data that we preserve and make special, that we can really do this on? Or do we really focus a lot on, oh, let's just hurry up and modernize and get that data lake in better shape? I think folks are really struggling with the trade- offs around how to prepare.
Paco Nathan [00:39:09] If you don't understand your data catalog, if you don't understand your metadata, if you don't understand where your data is being produced. Data is not a static thing, you have a business, it's ongoing, there's data exhaust all the time probably. And if you don't understand your catalog and what's going on, what you can leverage, because the thing is when you really get in and start doing the machine learning, you find out, hey, there's some other signals over there. We really need them. And this could take us from 80% to 95%. So we need to bring in that data. How can we get it? They're not letting us. Or nobody understands it, or the person who did this retired and they didn't bother to document or something like that. I've heard these, I think the case study out of Lyft that a friend of mine who ended up leaving Lyft and starting stema. org. The case study that they found there was when they started doing an internal data catalog of what their data scientists were using, what they found immediately was their data science teams, like 250 people at Lyft, which is you look at the lowest salary per year, that's a hefty investment. And they were spending about roughly 25% of their time rediscovering the catalog over and over on every project. And when they put a catalog in front of them, they were like, " Windfall."
Juan Sequeda [00:40:22] So these are the stories that we, I mean we work with all the time too. And I'm just curious to get your advice for folks listening is we started this conversation on hardcore, what is the latest stuff going on in research and translating it. And then going off on Hugging Face and all we've gone into, we started this really hardcore research and then we're like, wait. But then also we need to have ops, we started talking about the ops stuff. And then we're like going back inaudible. And then we're going back and I can't find my data-
Paco Nathan [00:40:53] Yeah, yeah, yeah, yeah, yeah.
Juan Sequeda [00:40:53] ...now we need a catalog. And it's like so you're telling us we got to go back to basics or we got to have the base foundations in place. So what is your recommendation? You speak to so many people, you work with so many people, what is your recommendation right now? This is the strategy, you need to be able to get from here to A to B. What do you tell people?
Paco Nathan [00:41:17] Well, I think you can start out, maybe you can bootstrap. You don't have to boil the ocean the first day. You can bootstrap by just starting to understand for the apps that are critical for you, what is the data that's going into it? Where are you getting it? So basically who's using it and why? And then number two, who's producing that? Do you know that product manager? Do they even know that you exist? Can you go out and have coffee with that product manager and say, " Hey, your stuff is cool, we want to help you, is there anything we can do to make sure that this keeps moving smoothly? And by the way, we know three other people in the same boat." Just start taking even just a real simplistic consumer- producer view of that and start building our catalog from there, because those are going to be priority based. And I guarantee for most enterprise, once you do that, you're going to have a lot of surprises and you'll probably need to call your SVPN to resolve some things. Just my take.
Juan Sequeda [00:42:18] Who should be doing that work, you said?
Paco Nathan [00:42:20] Yeah, well that's it. I think that again, business unit managers should be shielding it, but at some point you need to get exec cover because you're crossing across divisions probably, if it's anything useful. I hope there's some data from customer support, but I hope there's also some data from production and probably some data from sales. You're crossing a lot of lines and you really want exec air cover, because if you don't, people are going to shoot you down. So you need to get the attention of somebody who really matters, who can come in and say, " No, no, no, this is what we're doing." And if you can't get that, seriously, shake your network. Find a better place to be.
Juan Sequeda [00:43:03] That's the honest, no BS there. Tim, do you want to... Time is flying by and we going to keep going.
Tim Gasper [00:43:13] I know.
Juan Sequeda [00:43:13] Any your final comments, thoughts?
Tim Gasper [00:43:16] Yeah. I'll ask just one last question before we move to our lightning round, which is that we talked about a couple of different trends today including and especially around ML ops and the importance around that. Any other trends that you would point out as like, " Hey listeners, folks, you got to pay attention to this, this is some of the stuff that's changing a lot over the last couple of months here,"?
Paco Nathan [00:43:40] Yeah, that's a great one. Okay, so I do think, we talked also about data first strategy or knowledge first strategy, that kind of thing. This is also really crucial. And it's a counterfactual to a lot of what's in the headlines. So that's why I think it's so important. But also, I do think that there are really interesting communities of practice and you need to get involved with them. And even if your head's down in a corporation, you still need to understand and have some kind of input, because you're not going to wait until you read this in a Gartner Report because by then it's going to be way too late. If it even shows up in a Gartner. So I think go out and get involved with communities of practice. And I think there's a lot of benefit, that's a two- way street, and it's a great way to also understand who in your organization might really resonate with this kind of role and want to move into it, and promote within as well. So I think those are maybe three or four things that people could take away as overarching inaudible here.
Juan Sequeda [00:44:47] So let's head to our lightning round.
Paco Nathan [00:44:50] Okay, cool.
Juan Sequeda [00:44:50] All right, so first one, so you mentioned Andrew Yang's comment about data first, we talked a little about knowledge first and you mentioned it's been a long road. Will this become a mainstream in let's say five years?
Paco Nathan [00:45:05] I think it's shorter. I take a look at the timelines of when the ideas of data science and data products was what was being called back then. I can remember DJ Patil did to talk about data products and it took off like fire. Obviously these things, data analytics was going a lot longer before, but it really catalyzed circa 2009. And I don't think that it was really mainstream until maybe five years later. There's this thing that we have called J Curves when we look at technology adoption. Have you ever?
Juan Sequeda [00:45:37] J Curves?
Paco Nathan [00:45:38] Yeah. There's usually about a 15, it's maybe 12 to 15 year between them when there's like there's a couple of people who are doing a weird thing and it works for them to like they talk to some other people and there's a period of time before the ginormous corporation that never does anything early is putting it in a white paper for all their customers. There's about a 15- year span usually on average. And so, gosh, where am I going with this? Do we think it's going to hit within five years? I think it'll be sooner, because when we do look at how data science was adopted, you get past the early adopters clearly within four to five years. But I think that for the people in the know and seeing return on investment by people who are doing it right, that'll come much earlier. That's usually like two to three years out. And I think when people are looking at the proposition of doing more smaller specialized models, doing a lot of RAG and fine- tuning, whatever, they're burning a lot of money. These GPU instances in the cloud are not cheap. And you're a manager, you have a budget, you could hire people or you could burn up GPUs. And if you find out that your competitors are doing something a lot better as far as that cloud budget and they're hiring better people as a consequence and making more efficient budgets, I don't think that takes five years to propagate.
Juan Sequeda [00:47:00] That is a fantastic insight right there because you can say, if you don't invest in data and knowledge upfront, you're going to be spending more compute on doing stuff that's not going to work as well. And then your competitors who will be doing that, they will be getting there faster, spending less money in compute, they'll be able to go hire more people, they're going to go beat you.
Paco Nathan [00:47:25] Yeah.
Juan Sequeda [00:47:26] So the call here is go invest in high quality data and semantics and the knowledge before somebody else beats you over the head with it.
Tim Gasper [00:47:34] This stuff isn't cheap and make the investments and you're going to save a lot of money in the long run.
Juan Sequeda [00:47:39] I'm so excited, I can make this investment now. I could spend this money right now. And GPU is like, " No. How you spend that money prepping in your foundations, which it may not feel like you have an immediate return, but it's like that thing is just going to compile more over and over again.
Paco Nathan [00:47:53] And you look at how much you're spending on GPUs and then how much of a fraction of that would be to hire a product manager at large who's reporting to an exec to actually try to ferret it out where are these problems? And let's at least get a plan-
Juan Sequeda [00:48:05] So this conversation is making me realize that we should have a, I'd love to inaudible should out write something. I think it's probably actually inaudible, it's probably going to come out inaudible about the argument that you should be investing in product managers that's focusing on semantics and knowledge-
Paco Nathan [00:48:23] You should be investing in data and people. Well, people and data.
Tim Gasper [00:48:26] Yeah, there's a bigger picture here in terms of return on investment.
Juan Sequeda [00:48:31] Yes. Tim, you go.
Tim Gasper [00:48:32] All right, second question. So you mentioned machine learning talent and best practices and communities of practice. Can ML ops be solved with technology? How much of it can be a technology problem?
Paco Nathan [00:48:48] No, no, no. It's a person problem, I think. Part of it is just that it's an evolving landscape, rapidly evolving. You need to have people who are committed, who number one, like their job and want to do better in it. And number two, they understand the business. And number three, everything's rapidly evolving underneath them. So they need to be keeping up on their skills. So to come in and say that you're going to buy a magical vendor that solves all these problems, no, no, no. This is a people problem.
Juan Sequeda [00:49:18] All right. I'm happily surprised with that answer because I feel a lot when it comes to the ops, it's always tools and more tools, and buy a real platform.
Tim Gasper [00:49:28] Yeah. There are a lot of interesting tools there. I know one of our customers is using Dataco, for example, to wire a lot of stuff. But there's people, there's people in process and stuff and things change rapidly, right?
Juan Sequeda [00:49:38] Man, the amount of emails I get every day right now of just some vendors saying like, " Oh..." Anyways, I don't want to throw anything inaudible.
Tim Gasper [00:49:45] End to end data ops pipeline, right?
Juan Sequeda [00:49:47] That will increase this inaudible, anyways. Well, we're vendors, we understand it's all part of the work. Yeah. Anyways, next question. Is a catalog part of the AI stack?
Paco Nathan [00:49:55] Oh God, yeah. It's foundational. It's absolutely foundational, because if you can't get a comprehensive picture of what's going on in your data, in your business, where do you go? What are you doing?
Juan Sequeda [00:50:11] Perfect. This is music to my ears, obviously.
Paco Nathan [00:50:16] I want to jump up and down and say that, but I wish I could underscore that more. But it's a simple message. If you do not have data availability and understanding of things like data quality and also who to talk to, who are you really partnering with inside your company? And understand that people aspect of it. If you don't understand that, but yet you're basing your business on the results of that data. We know how this story ends and it's not pretty.
Juan Sequeda [00:50:46] Tim, take us away with the final lightning round.
Tim Gasper [00:50:48] Right, final question. We discussed the need for product managers. What would be, in your opinion, better, taking a product person and trying to immerse them in the sort of machine learning and AI and operations side of things? Or somebody who's immersed in machine learning and AI and help them become a product person?
Paco Nathan [00:51:14] Having been a manager and a manager of managers, my question would be a little bit different. I'd like to find out who the people are who are curious and somewhat hungry. And I think you could go either route, but unless the person's really got it inside themselves to go after this, it's not going to happen. So I think there's a lot of great ways for somebody who's not an expert in machine learning to start picking it up and really understand what does this technology do? It's not complete and utter magic. And I think it's not a long learning curve to really get a feel for what are some case studies? How can we apply this in business? So I think that can be acquired. If you are a product manager and you know that role and you want to learn more about what's going on in contemporary and machine learning, definitely that can go. But the other way around-
Tim Gasper [00:52:01] Yeah, I think that's great advice. Yeah, that's great advice because I do think that some folks who either are product people or maybe more business folks, they look at what's going on around AI and ML and they're like, " Oh my gosh, this is magic, this is black box. I don't understand." But they do understand some around data and they probably actually do have the curiosity and the hunger as you mentioned. So if you're listening and you're feeling intimidated, don't be. Dive in.
Paco Nathan [00:52:28] Yeah, no. One of my favorite examples, I had a friend who'd come out of being a lieutenant in the army and he knew how to manage people in real critical situations. But he really liked tech and he was like, " I'm going to dive into this." And those are the kind of people skills, that's the kind of motivation you need and the rest of it you can pick up.
Juan Sequeda [00:52:48] All right, takeaway time. Tim, take us away with takeaways.
Tim Gasper [00:52:53] All right. Well, we kicked this all off around the honest, no BS around taking open- source innovation and trying to translate it into the real world and what are the problems around that. And you started off by saying, well, there's a lot really going on around AI in general of course, but especially in the open- source community and the research community, there's a lot that's going there. And it's very exciting. And one thing that's been really good to see is how much maybe some of the previous hesitancy around really bringing research and putting it out there, a lot of that hesitancy has gone away, which is really, really important. And there's also a really tight loop now driven by all the innovation that's happening, the fast changes that are going on. Also, the partnerships between the research community and industry. There's actually a really fast and close iterative loop going on right now between research and the use of that research, which is great, and hopefully that really continues. And AI has really been pushing that. The speed of AI is moving so fast. If you want to innovate in AI, machine learning, and knowledge graph, you need to look at what's happening in that research side of things because there's a lot happening there, a lot of change. And a lot of it can translate into the real world. But you put up an important and big caution here, which is that there's a few things that are not going right with this. One of them is, for example, the reproducibility, which is not there enough and more work needs to go there, which might be okay for an academic student, but not okay for a well- funded institution. And you mentioned also bad behavior like chasing benchmarks, and we really need to look at not just the benchmark itself, or not just doing better in the benchmark, but can you improve the benchmark? Look at the underlying data, look at the underlying tooling. And you mentioned for example, DPO, direct preference optimization and some of the different tools that are coming out of Hugging Face to measure the data, training sets, benchmarks, evals-
Paco Nathan [00:54:57] Model cards for product, things like that, yeah.
Tim Gasper [00:54:59] Exactly, exactly. And the thing that I wrote down in my notes in bold was we need to have a more holistic approach and thought process around the full data to AI chain. And so not just thinking about one piece of it or just the performance piece or just the training piece. You got to look end to end and think about, hey, maybe it's not even a chain, it's more like a pyramid. And you got to look at the foundational aspects, not just what's on top. So I thought that there was some really good takeaways there. Juan, what about you? What were your big takeaways?
Juan Sequeda [00:55:33] So I'm really happy we connected on the whole data first, knowledge first and you said it's going to be a long road. And one of the things that frustrates is when it's successful, you have a successful data first, knowledge first approach that doesn't get the headlines. But then at the end of the day, you know what? We just need to go focus and just win, show how we are beating the competition, being successful because we invested in data and knowledge first. I think that we just go drive that and we got to be doers here. What are the latest trends today? Well, the one that you're highlighting a lot is that we need these ML lops. You can have the best ML engineers, you can have the best knowledge graphs, the best apps, but you need to be able to have these ops tools and the talents otherwise will fail. You have to deal with all these security, legal and governance consequences and pushing things to production.
Paco Nathan [00:56:17] And I should footnote that I don't mean drop everything and only devote yourself to ML ops. It's like if you're in software engineering, cool, that's extremely important. Pick up some ML ops as well, and by definition, you start to become that unicorn.
Juan Sequeda [00:56:33] And it's really interesting is that there's all these communities of practice around ML ops and stuff, so much to be done there. But it's still grassroots, kind of similar to what data science was in early 2010s. So I think we're changing a lot and growing together as a community here. That data first strategy and the knowledge first strategy, I love how we were just talking briefly, is like you can go invest so much and spend your time on GPUs and all that money on compute, but you can also be spending on making sure you're doing your data very well. And then you can actually have more people that you can go higher around that. And then connecting all this to the enterprise, it's like how do we set ourselves up for success? One, be careful about if executives are just hearing, they just want ChatGPT and so forth, they have to be careful, they're too disconnected from the reality. It is hard to hire people who are product managers, that's one of the things. And we don't understand the business and the technology with the team. Again, we talked about ML ops and one of those things is that it's hard to go hire them because it's a competitive role, the HR is also out of date about this stuff. And also, it's a placeholder. This name can change and so forth, the end. We talk about data availability and catalog is still an issue. If you don't understand your catalog, your metadata, well that is a problem. And we wrapped up with this, your strategy recommendation. One of my favorite quotes always is don't boil the ocean and just go find those critical apps in your organization. Go find how the data gets there, what goes in it, what depends on that. Go find the product managers behind that. Go have coffee with them saying, " Hey, we want to go support you guys and how do you do this?" And then build a catalog from there. But it's really important, you need exec air cover because you're crossing boundaries and if you don't get it, then start shaking your network and go find the next place because you want to be in a place that's going to succeed.
Paco Nathan [00:58:14] Right.
Juan Sequeda [00:58:14] How did we do?
Paco Nathan [00:58:16] Awesome.
Juan Sequeda [00:58:16] Cheers. Well, this was all you. This was all you. Wrap it up. Three questions. What's your advice? Who should we invite next? And what resources do you follow?
Paco Nathan [00:58:27] Advice, what's my advice?
Juan Sequeda [00:58:28] About data, about life-
Paco Nathan [00:58:30] Everything.
Juan Sequeda [00:58:30] ...whatever you want.
Paco Nathan [00:58:31] Okay. Well it is my birthday. Wow, I need water. Yeah, no, it's my birthday-
Juan Sequeda [00:58:39] By the way, that was a big clock right now, if you heard in the background, so you survived your birthday too.
Paco Nathan [00:58:43] Yeah, it's no longer my birthday. No, okay. So full disclosure, I'm in my 60s, I write code every day. I love it. I have gone in my career all the way up to being CTO and board member for two different publicly traded tech firms. And I really hated it because of all the lawsuits and crap that goes on. But that's like any stocks, that's the problem. But I've gone through different roles, been in different exec staff, I've been in high- flying startups, I've been in low flying startups as well. I've seen a lot of disasters. But I've been through a lot of roles and I've really enjoyed doing sales engineering. I really enjoyed going in and closing a deal. But at the end of the day, in my 60s, I love writing code. So I would just say, dammit, figure out what you like, get a lot of background because it's going to come in handy. Keep going at it. A lot of people say that you should spend five, 10 years being a programmer and then become a manager and work your way up, and it's so much bullshit. If you're good at what you're doing, do it and figure out what's even better next.
Juan Sequeda [00:59:46] That is, I love this advice.
Tim Gasper [00:59:48] That's good advice.
Juan Sequeda [00:59:49] You get your honest, there'll be a spirit right there.
Paco Nathan [00:59:51] Okay, good. Trying to be true to this form.
Juan Sequeda [00:59:54] Yeah, all right. Who should we invite next?
Paco Nathan [00:59:56] Okay, so we were talking about communities of practice. There's a few people there. One of them I would highly recommend, there's a guy named Demetrios Brinkmann and he is running a thing called MLOps Community. And it's worldwide, there's chapters meetups in real life all around. He's actually based close to here, I think in Frankfurt. But there's people, it's global, it's all virtual. And there's a conference coming up, Feb 15. I helped with a lot of the speakers. It'll be Feb 15 and Feb 22, it's free. But I would say he's got a real finger on the pulse from a broad spectrum of this. Grill him, get his no BS take.
Juan Sequeda [01:00:35] Awesome, that's great.
Tim Gasper [01:00:36] Great idea.
Juan Sequeda [01:00:37] Cool. Finally, what resources do you follow?
Paco Nathan [01:00:40] Wow.
Juan Sequeda [01:00:42] From people, from blogs, from LinkedIn, from magazines?
Paco Nathan [01:00:46] Yeah, cool, cool. Okay, so one thing that I follow religiously is Team Cymru. It's a security vulnerability analysis, comes across usually about 10, 12 items per day, but summaries of top vulnerabilities or top attacks, just the security space. I used to work in security and I really want to keep a hand in it because it's so important and so much is going on and it's also our customers care about that. So I really watch the security space because it's so strange and it evolves so quickly. Another one I listened to is actually geopolitics is War on the Rocks. It's$ 150 a year for a subscription, but I love it. Some of it's out of Austin at the Business School I think. But other parts are out of DC. But it's like a bipartisan, non- denominational look at what's happening in the world, but from a military perspective. I used to be in the military. I've done a lot of work for DOD. So those are things that are important for me to get perspective on, understanding what was going on. I'm also on Hugging Face all the time and I love the new papers. And I go through and do a lot of cataloging on my own to put together collections of like here's papers of things that are emerging, because there are really great AI tools on Hugging Face, like here's my collection, what am I missing? Go out and find the lookalikes and recommend to me a reading list. And yeah, I use that for a lot of bootstrap, but I also just look at what their top summaries are and what's on leaderboards and what's interesting. So those are probably my three biggest things, other than I don't really read US media as much. I actually read The Guardian, the UK version, and it just gives me a little bit better worldview of things. So that's me.
Juan Sequeda [01:02:28] All right, well just quick, inaudible. Next week we have Eva Nahari. We're going to be talking a lot about the state of VCs and startups investing and it's up and down time. But with that, Paco, thank you so much. This is fantastic. As always, thanks Data. world who lets us do this every Wednesday. And with that, cheers.
Tim Gasper [01:02:47] Cheers.
Paco Nathan [01:02:47] Cheers. Thank you so much.
Special guests
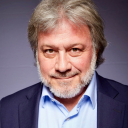