Tim Gasper [00:00:06] Hello everyone. Welcome. It's time for Catalog& Cocktails. It's your honest, no- BS, non- salesy conversation about enterprise data management with tasty beverages in hand. I'm Tim Gasper, longtime product guy, customer guy at Data. World, joined by Co- host Juan Sequeda. Hey Juan.
Juan Sequeda [00:00:20] Hey, Tim. How are you doing? I'm Juan Sequeda, principal scientist here at Data. World. As always, it is Wednesday, middle of the week, end of the day, and it's time to chat about data, about knowledge graphs today and oh my, today's such an exciting day. So first of all, we're here live from the Knowledge Graph Conference, and we actually have a little bit of a live audience. We'll talk about them in a second. And I'm super excited to have our guest today who is my great friend and also co- author, Ora Lassila, who is the principal technologist at AWS Neptune, who is actually one of the original editors of the RDF Graph Standard from 1997, the author of the Semantic Web article Vision Article, it was in Scientific American; I think the grandfather, what I want to call it, about knowledge graphs. So I can keep going on and on. Ora, finally we have you- inaudible.
Ora Lassila [00:01:14] You have to understand, I was just a wee kid when I did this, because obviously otherwise I sound old.
Juan Sequeda [00:01:22] Well, welcome. Thank you so much. How are you doing?
Ora Lassila [00:01:24] Good, good, good. Thank you so much for inviting me. It's really great to be here and looking forward to chatting about good stuff.
Juan Sequeda [00:01:34] We got a lot of... We asked questions to the folks on LinkedIn, what we wanted to ask. We're going to go through that. But in the meantime, let's kick it off. What are we drinking and what are we toasting for? Tim, I know you're coming in hot. What do you have for us today?
Tim Gasper [00:01:47] I have a Dark'n Stormy, but it's with mint in it. So I came in hot, but I still got to have a cocktail, so we're good there.
Juan Sequeda [00:01:57] You got a cocktail? We actually just got something downstairs. We're in New York right now, so we got a Brooklyn lager.
Ora Lassila [00:02:02] It's not bad.
Juan Sequeda [00:02:03] It's actually pretty good. It's a classic one- inaudible.
Tim Gasper [00:02:06] That's a good beer. Yeah.
Juan Sequeda [00:02:06] And let's cheers to, I mean, I've always liked to cheers to be in person when we have guests. We're at the Knowledge Graph Conference, it's the fourth, five, fifth year here. So anyways, cheers to that.
Ora Lassila [00:02:17] Cheers.
Tim Gasper [00:02:17] Cheers to a great event.
Juan Sequeda [00:02:19] All right. So we have the question. So the title of this podcast episode is Everything you Wanted to Know about Knowledge Graphs, But Were Afraid to Ask. So the question is, everything you wanted to know about X, but you were afraid to ask, what is X?
Ora Lassila [00:02:36] What is X? Well, the one thing I don't understand, and I suppose I should ask questions, is all this GenAI stuff. Call me a skeptic.
Juan Sequeda [00:02:48] Oh, interesting. All right, you Tim. How about what's your X?
Tim Gasper [00:02:53] For, and specific to Knowledge Graphs?
Juan Sequeda [00:02:56] No, anything, just in general. I'm thinking about outside of work right now, outside technology.
Tim Gasper [00:03:01] Oh, it could be anything, huh. I think the biggest X topic for me right now, it's GenAI as well, but it's not the skepticism part of it. It's, how are these applications going to be built? I have a lot of questions about the right architectures and things like that, which I bet connects very close to yours. So that's my nerdy question, or my nerdy answer there.
Juan Sequeda [00:03:27] Cool. Yeah. Well then it goes some way different. So for me it's, what happens in the boardrooms of publicly traded companies? I've always wondered what happens there with all the stuff. There's so many questions I have there that now, how do they prepare for the big reporting and stuff like that?
Ora Lassila [00:03:44] You should ask your favorite LLM.
Juan Sequeda [00:03:48] All right, well let's dive into this discussion. We actually have a series of questions. We're going to change the podcast a little bit today because we always just kind of dive into the topic and just start honest no BS, but we went on LinkedIn and I have a bunch of, I think six, seven questions we got from folks. So we're going to go through these questions, and we've separated them from technical questions and business questions. But to kick this off, for a honest no BS, okay. Tell us the history about all this knowledge graphs and how did we get here? It's 2024. We're at the Knowledge Graph Conference. How did we get here?
Ora Lassila [00:04:17] It's the old guy stuff again. You asked me about history of things. But, sure. So none of this is new. So of course all of this goes back to the idea of knowledge representation as a sort of a branch of, subfield of AI. Knowledge representation is interested in the kind of representation of the world and representation of data that lends itself to drawing conclusions from and doing, reasoning over. So that's like the prehistoric backdrop of this. And then in some ways, then we have to go, there's the idea of the semantic web, which kind of serves as sort of the predecessor of the modern enterprise knowledge graph. The vision of the semantic web came about, jeez, 25 years ago or so. I was somewhat involved in that, and in many ways we could say that, we could kind of redefine or reimagine that vision today. The original vision was had a very broad scope. The scope was the whole web and things like that. But now I think the scope is more like the enterprise. In some ways all the things we said about the semantic web and dreamed about it, we can now realize in a sort of enterprise context, and enterprises can, those organizations inaudible.
Juan Sequeda [00:06:07] So how would you define the semantic web vision?
Ora Lassila [00:06:14] Well, let me tell you a story. Back in, I think this might've been 1996 or something like that, I was at MIT and Tim Berners- Lee comes into my office and he says, " So, Ora, what's wrong with the web?" And this is kind of a scary question, considering who's asking. And I'm like, " Well Tim, I'd like to build agents. I'd like to build these things that go out there and do stuff on my behalf, but I can't do that because the web was built for humans and can't build these agents." And he gets kind of excited that night and says, " Yeah, that's it. That is what's wrong with the web. Now how do we fix that?" And I said, " Well, I am not sure, but what if we try things from the world of ontologies and knowledge representation and see if we could apply that?" Then he gets very excited. He leaves and when he's at the door, he turns around and he points at me and says, " Okay, now you do it." So this is kind of my story about being in the wrong room at the wrong time, because that's all I've done ever since essentially. And so what is the vision really? So the vision is that we have knowledge, information, data, whatever, out there in such form that these automated agents, however you want to define that, can really take advantage of that. And so we are able to combine information from different sources, and all of this can happen without human intervention. That's really where all this got started. So now if we fast forward and we think about what is a knowledge graph, really? Okay, so first of all, I work with a lot of people who want to use a graph database, but they're not building a knowledge graph. So not all graphs are knowledge graphs. And knowledge graph in some sense is, I think of it as a way to take information from many different sources and integrate it and in the process sort of insulate the applications that you're then building, whether those could be agents or something else, insulate them from all the crap that has to do with the legacy data sources that you have. So that kind of architecture I think is the quintessential typical knowledge graph architecture. You have data sources that are, who knows what they are, relational data sources or anything else. All of this stuff comes into your knowledge graph. You have an ontology, which maybe we should explain what an ontology is. So ontology is really just kind of like a rich expressive data model, the extreme manifestation of a logical model in some sense. And you have an ontology that essentially describes the data. So all the legacy data, it gets mapped into this graph. The ontology kind of models that. And once you have that, you can now build applications on top of that, and they don't have to know anything about the legacy where that data came from. And now all of a sudden... The historical view of course is that applications are gatekeepers to a particular kind of data, and this is not a good situation obviously, because it leads to these silos and things like that. So now when you have the knowledge graph, applications change their nature. They're no longer gatekeepers to a particular kind of data. They're more like sort of an expression of the user's intent. What do I want to do, rather than where's the data I somehow have to have to do something. To me that's kind of like the high level view of knowledge graphs. And then of course there's all kinds of technical details to this, and I don't think the technical details really are all that important. I mean, we wrote this book and we didn't really get into technology very much. We talked about building knowledge graphs and never really talked about technology very much.
Juan Sequeda [00:10:42] I think from the knowledge, from our book perspective, we talked about how to map and how to bring in all the data, which is in relational databases, but even the notations that we used in the book was specifically agnostic on what mapping technology, is it RDF? Is it property graph? Having said all of this, I want to, let's dive a little bit into the technical side. Let's just get the technical stuff out because people have questions about this, and then we kind of pass it on to on the business side. So technical side, one is let's talk about tables and graphs. I think historically the focus in the data world has always been about tables and columns and stuff. How do we convince people or what does it mean to say, " Oh, from tables to graphs," and does that mean we stopped doing tables and we should all live in a world of graphs, or basically tables and graphs? What do you tell people there?
Ora Lassila [00:11:33] I'm really, really sorry that you posed the question that way because it sort of implies that tables are somehow important. And of course they are. I'm just kidding. But there is a distinct difference in how do you explain graphs to people who come from the table world, versus people who have sort of... Like children, how do you explain graphs to children? They don't know anything about tables or anything else for that matter. And graphs are an incredibly intuitive way to express things and to model the world. And I'm sorry to say that I feel like the more you are exposed to modern enterprise IT practice or the more computer science education you have, the less ability you have to sort of accept graphs as an intuitive way to do things. I don't know what it is. I mean maybe you can become jaded or something, but. We put that quote in our book from Ludwig Wittgenstein, which goes, " The limits of my language mean the limits of my world." And Wittgenstein was onto something. Now of course in this discussion, when I say language, I mean Sequel, right? So people who have been doing Sequel for a long time and have been thinking in terms of tables, it's kind of hard sometimes for these people to make the leap to this. Essentially, it is a completely different way to think about how you organize your data. And then yet again, it isn't. But the reason why I like graphs rather than tables is that table- based representation usually means that you have to think upfront what your data will look like, and then you kind of shove the world into this thing. Then the world may not fit very well into your set of tables. And graphs give you more flexibility. And even if you didn't think about everything up front, you can easily add them later. And I really, really like the flexibility.
Juan Sequeda [00:13:57] So I think this is one of the arguments again, they're always about the graphs are the flexibility and it's that natural way. And I agree that the limits of my language are the limits of my world. And frankly, if you're just used to everything's a table, and you've been doing it for decades and decades, it's hard to convince you otherwise. So I think this is the opportunity to start educating the next generation, to say, " Oh, there's more things than just tables, and there's graphs." And just even how we think about things and how we model and how we conceptualize and how we have conversations and how we try to conceptualize and codify what we're talking about in this room. And there's a whiteboard, let's go talk about that stuff. I think that's more than... We need more...
Ora Lassila [00:14:36] Well, I mean the whiteboards are an important component in this, obviously, because we all know graphs because that's how we draw on whiteboards. We draw circles and arrows and we label them somehow. And people,, sometimes they're so kind of surprised at what they've drawn on the whiteboard and I tell them, " Well, that's the graph." And they're like, " Wait, what?" But graphs are not enough. There's more. It's like inaudible. I sound like an infomercial. " Wait, there's more." So modeling and vocabularies and how do we identify things? Those things are important because if we're truly talking about taking data from multiple sources and wanting to build applications that have kind of access to the breadth of data regardless of where it came from, you have to have really solid means of, first of all, expressing the models that you're using, and then really solid ways of identifying things. I always tell people I work with that when you're building a knowledge graph, it sort of lives or dies based on how well you deal with identifiers. It sounds kind of trivial in some sense, but it's one of those things that good choice of identifiers, good way of minting identifiers, is something that really helps you when you're building a knowledge graph.
Tim Gasper [00:16:17] Is that different you would say, than something you have to worry about in the table world? Is this something that's more unique to the graph world, and why is it so important?
Ora Lassila [00:16:27] Well, okay, so now I guess we will have to talk about the open world assumption and the closed world assumption. Because databases historically, and this is whether they are relational databases or something else, kind of operate on the idea that everything that's in the world is in the database, and stuff that's not in the database doesn't exist. And so the data in your database choice of identifiers is often easy, because there are no identifiers outside the database. So you're never worried about getting some other data that has some other kinds of identifiers. But when you're building these, which by nature are open systems, these knowledge graphs, you have to assume that there's going to be data coming from outside, and thus you cannot rely on some scheme of identifying things that is only in your database. I don't know if anybody understands this explanation, but that's essentially what we're talking about. So people who have been building applications using let's say relational databases, those applications are closed. What's in the database is the whole world. Nothing else exists, nothing else matters. And in a knowledge graph that basically integrates data from here and there, simply, it would simply be foolish to make that kind of an assumption.
Juan Sequeda [00:18:13] Well, I think that when it comes to identifiers, because we're so used to dealing with the stuff that's right in front of us, so you think it's that closed role, it's right there. But when you're, want to go deal with what I call the known use cases of today and those unknown use cases of tomorrow that you want to be able to facilitate, connect the data of stuff, I don't know what's going to happen tomorrow. I need, how do I connect things? How do I link things together? That's where the identities come from. That's why you should be invested in that. Now I think identifiers in general is something that we just don't think about it. We don't treat it as a first class citizen. And I think that's one of the reasons why we'd do well-
Ora Lassila [00:18:51] Because it's been so easy. You can just take integers starting from one and go from there, because there's nothing else outside your database. So why not? But I gave this talk once about RDF and building RDF graphs, and I said that RDF is the best technology for your use cases that you haven't articulated yet. And people thought I was joking, but I'm not joking. There is, these technologies that we now use for building knowledge graphs. There is this almost magical sort of characteristic of serendipity about them. You build these things and you bring more data in and new data connects with your old data, and you find out you can ask questions that you couldn't ask before, and things like that. And there will always be use cases that you didn't think of. There will always be applications that you didn't think of but somebody else will think of. And having sort of a foundation where introducing some new use case or new application doesn't mean that you have to completely overhaul how you represent your data or redo your scheme or something like that, is super important.
Juan Sequeda [00:20:14] So I think the other topic we need to hit on this, on the technical side. Now we said about RDF, which by the way, that's a T- shirt quote right there. I have this here. " RDF is the best technology for the use case that you haven't been able to articulate yet."
Tim Gasper [00:20:28] Perfect.
Juan Sequeda [00:20:29] Another T- shirt, Tim.
Ora Lassila [00:20:30] I will want one of those.
Juan Sequeda [00:20:31] All right, let's talk about the whole RDF and property graph. I know that's a question people will always have. You are the co- chair of this, the new RDF working group, and now there is a GQL standard query language that just came up. So RDF property graph, RDF star, GQL. Please enlighten our audience and take it away.
Ora Lassila [00:20:54] Looking for my soapbox because I'm going to start ranting now, but okay. So yeah, so the backdrop of this is that there, graph market, kind of nascent market, and there's this rift in our industry. We have two different kinds of graphs. We have RDF graphs, and we have labeled property graphs, and they're not the same. And sometimes I feel like there's almost like some kind of religious following of this. And generally speaking, I think that this rift, it hurts the industry and it hurts the adoption of these technologies. There is still a question, do people really want to adopt these technologies? And if they're confused about exactly, " Oh, there's multiple technologies that I have to choose between," that's not going to help. So a couple of things are going on. So we'd like to find an alignment, better alignment between these two different kinds of graph models. And when I say we, I'm now talking about my team, the Neptune graph database team at AWS. We support both kinds of graph models, but the way we do that is that the user basically has to choose upfront what they're using. And when they choose, they also choose the query languages that they can use. And sometimes later on they find that they made the wrong choice. And walking back that decision is not always so easy. So what we would like to do is to basically say, " Well, even if you made a choice this way and you now want to go this way, so for example, you don't want to use SPARQL anymore, you want to use OpenCypher, that should be possible." So we have this ongoing effort that aims at doing what we call graph interoperability. And it really means that your choice of graph model doesn't dictate the other technology choices that you have. So you have more freedom. We like to give the customer everything. So that's kind of like what we want to do. One of the things that customers often cite when we ask them, " Why did you choose property graphs and not RDF?" And they say, " Well, property graphs lets you put properties on edges." And so there's this longstanding activity in the RDF community to make it easy to do just that in RDF as well. And of course in RDF since the very beginning, we have this mechanism that we call reification that technically would let you do something, logically speaking, something like that, but it's very awkward and kind of cumbersome and nobody wants to use it. And this gave sort of a rise to this new activity that we call RDF Star. And first the W3C, the World Wide Web Consortium, they organized what they call a community group that's kind of like sort of an exploratory activity. And then about a year and a half ago we formed an actual working group. And in W3C, working groups are the ones that actually produce the W3C specifications, which W3C parlance are called recommendations. And so we're working on this, and the aspiration is that the outcome is a new set of specifications that we would call RDF 1.2 and SPARQL 1. 2. And if this all goes well, RDF will have a mechanism that brings RDF in a way sort of closer to labeled property graphs. And that will allow us, the Neptune team, to fulfill this aspiration of ours, to build this graph interoperability. I mean we're going to build it anyway, but having the support from a W3C specification of course would be a really great thing. We like open standards, But bottom line is there's this rift. People have religious feelings about these things, and there are, many times there are use cases where it's very clear which kind of graph model you really would like to pick. And sometimes it's not all that clear. RDF, in my mind, is much better suited for building knowledge graphs because RDF has support for the kinds of identifiers that we need. RDF has predictable behavior when you're merging graphs, and RDF has the support for defining vocabularies and schemas and ontologies, which lets you do this modeling of the graphs. But sometimes you build graph applications that don't need this, and they might be closed systems, and property graphs are often a good choice for something like that.
Juan Sequeda [00:26:45] Well, I appreciate how you've walked us through this, and I think what's really important, something you said at the beginning, which is having this rift is not beneficial for the industry. And I was, actually early this morning, one of the cool things that, Bob Metcalf is here at the Knowledge Graph Conference. You've met Bob Metcalf, Turing Award winner? He's actually been coming here for many years, so if you want to know where the puck is heading, Turing award winners are coming to the Knowledge Graph Conference. This is the place to be. And he was telling me, I was like, " Yeah, it's very evident, these are religious wars." And he's like, " Yeah, well I think I've seen these religious wars before." We were talking about things like ether and stuff. So it's like, " Yeah, this is just common in the industry, it won't happen and it'll get over it." And I appreciate the work that you're doing and think all the standards work is because that's how we're going to bridge together. And it'll take time, but it will happen. So.
Ora Lassila [00:27:38] Bringing religions together.
Juan Sequeda [00:27:39] All right, well there's more technical stuff to get into, but enough technical stuff. Let's move on to some other side. Tim, I want to throw it to you.
Tim Gasper [00:27:48] Yeah, we've got some more business- oriented questions now for you, Ora. And one of the first things that I'd love to explore with you is around the use cases. And I think one thing that we sometimes see around knowledge graphs is that sometimes folks get excited about almost toy kind of use cases, or more just the look and feel of the knowledge graph. They can say like, " Oh, look at all my nodes and edges, I visualized it and you can explore it. Isn't that wonderful?" But of course even Juan and I sometimes get skeptical about why are people so obsessed with visualizing the graph? What is that doing for them?
Ora Lassila [00:28:30] I'm with you there. I'm totally with you there.
Tim Gasper [00:28:33] Where are the biggest use cases that you think people should be focusing their attention when it comes to knowledge graphs?
Ora Lassila [00:28:40] If we really think about the issues we have in the enterprise world and what the problems with data that enterprises have, I think data integration is a great sort of, shall we now say category of use cases? This thing that I described earlier, that you can really do data integration and then in a way sort of hide the nasty details of the source data, and basically provide this layer that's much cleaner and build applications on that rather than on having the applications separately deal with the integration issues. So I think this is a big use case. Now, other use cases that I've seen that really seem to resonate with many organizations is basically sort of the ability to capture the knowledge that exists in an organization in a way that makes that knowledge and that information accessible to all the members of the organization. I tend to call that the democratization of data. Just make it easier to access data. Graphs are, as a representation, they're easy to understand. I mean on a sort of purely kind of logical level, forget the technical details, but accessing graph data really is just walking in the graph, hopping from one node to the next. And when people ask me, " What are graph queries?" I always say, " Graph query is basically the answer to the question, how do I get there from here?" Seriously, oftentimes it is. And people basically, if you give people access to data that they otherwise would have to be experts in SQL and they would have to be database administrators or whatnot. If you make it easier for them to access that data, then all kinds of things can happen. I mean there's all kinds of opportunities that gives to organizations and people in organization. I think of that as a very, very positive thing. And then one more is something that's very, very popular nowadays, is what people call digital twins. And I think of this as kind of a special case of a knowledge graph. A digital twin is essentially sort of a digital facsimile or some kind of representation of something that exists in the physical world. So the folks who are doing things like the internet of things, they have sensors and other things, they want to have a digital representation of this that lets them basically manipulate the network of sensors they have and all that other stuff. People are building digital twins of their supply chains, which then lets them sort of better understand what's going on in their supply chain and things like that. That's a very big use case nowadays.
Tim Gasper [00:32:20] That's interesting. I've heard digital twin coming up more often lately. And why is graph the best representation for digital twins? Is it because of how you want to have a very flexible model to represent the relationships between these things and analyze their relationships and things like that? Or is it something else?
Ora Lassila [00:32:41] No, I think it is that. I mean flexibility and essentially just the inherent diversity of the real world. I mean, like I said in the beginning, you can't always shove the world in a sort of predefined box that the table or tables would be. Things in the real world are more diverse. And if you want to model the full diversity of the real world, you need something like a graph to really do that. And don't take my word for it. There's ample evidence of that.
Tim Gasper [00:33:22] A lot of different companies implementing different digital twins use cases on knowledge graphs for this reason. And so data integration, I know a lot of people are talking about semantic layers and things like that, and in many cases you look at what some different companies are trying to do around that, and then you look at knowledge graph. I know sometimes Juan you joke that you have a worry that companies are going to try to reinvent RDF that are trying to develop semantic layers and things like that, right? It's like there's a thing already.
Ora Lassila [00:33:54] That's not a worry, that's happening. I mean that worry has been realized big time. People have always been trying to reinvent, kind of recreate the RDF. And that in a way that's sort of, I don't want to sound melodramatic, but it's truly heartbreaking, because people put so much effort into building something and then I go tell them, " All of this is already in RDF." They're like, " Oh crap."
Tim Gasper [00:34:24] And it wasn't easy, either. Some folks are like, " Why don't we just slap a semantic layer on everything?" And it's like, " Yeah, we should, and it's a very hard problem." We inaudible a lot of it.
Juan Sequeda [00:34:35] My grumpy person hat on is like, " Oh, they hear that this semantic web thing is a failed vision and that's old and no, therefore, let's go do it again." I'm like, " Even if that's all the case, the principles are there. You're going off and starting to recreate everything." So much wheel reinvent-
Tim Gasper [00:34:54] Yeah, don't reinvent the wheel.
Juan Sequeda [00:34:55] But it happens every time, all the time.
Ora Lassila [00:34:58] My friend Jim Handler and I, so we inaudible the original vision for the semantic web, and five years after we gave a joint keynote at one of the semantics conferences. And the backdrop of this is that when our original vision was published, people accused us of being science fiction authors and whatnot. Some people were excited and other people were just like, " This is just BS." And so we gave this keynote five years after, and it was really fun because we could say, " Hey, this happened, this happened, this happened. We already have this working. And the joke's on you now." I think that was 2006. And a heck of a lot of stuff has happened since, and now we have truly scalable graph databases and people are building these enterprise knowledge graphs. And I look at that as the realization of the semantic web vision.
Tim Gasper [00:36:09] I think one kind of last major question I'll ask you on the business side, and there's sort of a couple of sub questions that go to it, are around the adoption and traction of knowledge graphs in the enterprise. And so I think we have a question here which is, " So knowledge graphs are the worst kept secret for compliance, legal and security management due to how well suited they are for those use cases. Yet the data fabric knowledge layer has been around for a while with mediocre interest and adoption." This person says, " I have my own thoughts on why knowledge graphs aren't widely adopted, but what is your opinion on why they're not widely adopted?"
Ora Lassila [00:36:51] I can't decide if the first part of that question is just sarcastic. And data fabric, I have no idea really what that means. All these terms that come up, honestly, I can't keep track of what these things are. I think that there is adoption. We see adoption, and there are a lot of people in this conference, and this conference is growing, the knowledge graph conference where we are right now. So there's interest in this. I think the rift that I talked about earlier, that hurts because people are, it's to create some confusion as to what people should do and what technologies they should adopt. But yeah, the first part, I really don't know how to answer the first part of that question. What do you think it means?
Juan Sequeda [00:37:55] Well, I mean, I think, I have a hypothesis, which is many of the big large companies, Google's, right? LinkedIn's. They're all here, right? All the big companies, all the big banks are here. The JP Morgan's and Morgan Stanleys, all these companies are here and we know they're using knowledge graphs around this stuff. And I hypothesize a lot that people are like, " You know what? This stuff is working really, really well and it's actually giving me a very positive edge that I don't want other people to know about it. Therefore, I'm going to kind of keep quiet about it." And actually start looking at the people who's behind these systems, who's moving around. Go do some LinkedIn, people spelunking and like, " Oh, you see the heavy hitter?" So it's not as popular, but I think it is driving a lot of value, but quietly. So I mean that's a hypothesis I have there.
Ora Lassila [00:38:48] And I think there's a lot of adoption. And I honestly, somebody a couple of years ago asked me, " When do we declare victory? When do we say that knowledge graphs have succeeded?" And I said, my thinking was at the time, and I guess still is, that the day somebody builds some system and doesn't feel compelled to tell anybody that it's a knowledge graph based system, I think that's when we declare victory. I think that this is another one of those things that is going to be moving into the mainstream and then nobody thinks anything of it anymore because it becomes so natural. " Oh, yeah, of course we use a knowledge graph, of course we built a knowledge graph, of course we do data integration using a knowledge graph." I mean, heck, this has been sort of the repeating pattern in AI anyway. I mean, we don't talk about expert systems anymore, but at some point IBM started using the word business rules and all of a sudden the thing that was AI stopped being AI, and rule- based systems have just been absorbed into the mainstream. And similarly for many other technologies that kind of had their beginnings in the field of AI. So AI is kind of this thing where we come up with something and then we throw it over the fence to the mainstream side, and it stops being AI and we work on something else.
Juan Sequeda [00:40:19] I think, and we've been seeing this, the whole knowledge graph, the Google knowledge graph and stuff, it's was really over 10 years ago. And I think this has been one of the driving factors, and this stuff is in many, many places and people just don't want to, they don't know their history.
Ora Lassila [00:40:36] And I'd like to remind people that graphs are not new. Leonhard Euler published his first work on graph theory in 1734 or something like that. So there.
Juan Sequeda [00:40:50] Okay, so there's this other fantastic question we got from, actually Omar Khawaja, who's also a former Catalog& Cocktails guest. And the question is around the knowledge economy. So actually I want to go a side note. I'm very excited and privileged that we have a standing one- on- one every week, and Omar and I also chat usually once a month. And this is a topic that I had, we're chatting with over one of our days, and he said, " Do you see a future where with flourishing and sustainable knowledge economy, where we have a demand and supply of knowledge work and knowledge workers from industry and from academia? How do we make this beautiful future a reality?"
Ora Lassila [00:41:32] Well, that is an interesting question. Seriously, I don't know about knowledge workers, but the thing that maybe we should talk about is knowledge products. We've already kind of started talking about data products. Could there be such a thing as a knowledge product? And what would that look like? And I think most importantly, what would be the business model for something like that? For the semantic web vision, we sort of perhaps a bit naively thought that, " Oh yeah, everybody's just opening up these SPARQL query endpoints and data can move freely," but nobody thought about the business model for that, and it costs money to run a reasonable server that has a SPARQL endpoint, and somebody can come and write a query that brings the whole server to its knees. So we need to figure out exactly what is the business model for this. Many, many years ago, I used to work in a venture capital firm, and the one thing I learned that if somebody isn't going to pay for it's not going to happen. Okay? So I'm being slightly facetious here, but in some ways we have to figure out how it is that somebody can build knowledge graph and then essentially start selling that knowledge to others. I don't know yet how that would happen, but I would like to see it happen. And would that lead to some kind of knowledge work and knowledge workers? I don't know. I don't know anything about that.
Juan Sequeda [00:43:20] But what did we want to do that within the organization? And isn't the business model kind of, pretty... Let's think about this. You have, what is a business model internally that I want to be able to go share my knowledge? I mean, I want onboarding of employees to be very fast. I want to make sure that things don't get lost of how we do things. Right?
Ora Lassila [00:43:37] Okay. Right. Okay, good. Yeah. So in some sense, the transformation of the semantic web vision from a scope, that's the whole web into the scope that's the enterprise, has simplified a lot of things. Not everything, but I think that some of these things would be simpler. We're inside the organization, yes, we want to onboard employees or just generally share knowledge inside the organization, and we can make it easier that way. But one of the things, so I've worked in many large organizations and one of the things I learned is that when you cross the organizational boundaries inside a large corporation, you go to a different business unit. It's like you're in a different world. It's like in a different company. You are on somebody else's turf and there's questions of ownership, and people hold onto their data and things like that. And these are all things that we somehow need to figure out. But I think that doing this first inside an enterprise certainly would simplify some things. But I still would like to figure out what would it mean? Could I start a company that sells knowledge, and how would I do that? I mean, companies sell data, but in some ways I think knowledge is being somewhat different, particularly if I had a knowledge graph and people could come and let's say ask questions and stuff like that. How would I charge for that?
Juan Sequeda [00:45:19] I mean, you see all these companies, you can buy data from all these data vendors. What does it mean to go buy knowledge? But at some point you and I were starting to talk about, one person's data is another person's knowledge. I want to buy knowledge about the GDPs of every company.
Tim Gasper [00:45:38] Knowledge is data, right?
Ora Lassila [00:45:41] Well, yeah. I mean this is real food for thought.
Juan Sequeda [00:45:48] Tomato, tomato?
Ora Lassila [00:45:49] We may have to do this again someday after we've been thinking about.
Tim Gasper [00:45:53] No, it's not late enough. We need more cocktails, maybe.
Ora Lassila [00:45:55] More beer.
Juan Sequeda [00:45:57] More beer. All right, well look, there is so much I want to go through, but we want to go through our takeaways here. And I think in this episode we're switching things a little bit, no lightning round because we've had all these questions for folks. So I'm going to kick it off with our takeaways. So Tim, take us away with takeaways, but actually I'm going to take away this time.
Tim Gasper [00:46:16] This time, I'm going to say it. Okay, ready?
Juan Sequeda [00:46:18] All right, go inaudible.
Tim Gasper [00:46:18] Juan. Take us away with your Juan aways.
Juan Sequeda [00:46:23] All right, because I wanted to do this on the history because I'm super passionate about the history, and I learned something I didn't know, that story about Tim talking to you. So all right, history of knowledge. First of all, remember none of this is new. Knowledge representation is a subfield of AI, representation of the world of the data, of the world, and all the reasoning we're doing. Semantic web, we'll call it the predecessor of the Modern Knowledge Graph. And the original scope was really broad because it was a scope for the entire web. Remember it's the web. You usually say the word internet, but it's actually the web. My pet peeve. And the scope today, knowledge graphs are within an enterprise. So the semantic, but vision, I love the story. So you're at MIT and Tim Berners- Lee just walks through your door and says, " What's wrong with the web?" And you said, " I want to build agents that can do stuff." That's another T- shirt quote. Build agents that can do stuff, which guess what, that's kind of what people are talking about right now. But the web was built for humans. And how do we solve this? We should look at these ontologies and knowledge representations. So Tim said, " Let's go do it." And so at the end of the day, our goal is here to combine data from different sources without human intervention, and really knowledge graphs are this combination of data sources plus ontologies, which are this rich expressive data model, which can go to the extreme manifestation of what a logical model is. And the goal is that you want to go build apps on top of this, because today applications are gatekeepers to particular type of data, which leads to silos. With the knowledge graphs, they're not gatekeepers. Now it's about expressing about the user's intent. And something to realize is that not all graphs are knowledge graphs. So a couple more things. Technical questions. So we talk about tables and graphs. There is a big difference in how you explain graphs to folks that come from the data world, from the table world. Coming from the SQL world, it's hard to make that leap. It's different, but at the same time it isn't because it's like, " Yeah, it's good, I'm thinking about a table." But you're still kind of conceptualizing in a way. Graphs give that flexibility. Graphs are not enough though. We need modeling, we need vocabulary, we need to identify things. We talked a lot about identifiers as a very key thing to understand how you uniquely identify things. And I think what's really happened, which is interesting, is this open world and closed world assumption that if you think about it... I love this. RDF is the best technology for the use cases that you haven't been able to articulate today. Talking about RDF and labeled property graphs, you don't need to make that choice. I mean, the vision is that you should just be able to go choose whatever model it is and let it be SPARQL or cipher, and these things are coming together. But at the end, if you look at it today, RDF is best suited for knowledge graphic because it supports identifiers, predictive behaviors to merge graphs and the support for ontologies. And sometimes you just want to have a graph application where you really don't need that. So property graphs are fine. Tim, you go.
Tim Gasper [00:49:11] So much good stuff. All right. I was asking some questions around the business side of this, around the use cases and around adoption. And Ora, you mentioned that the biggest use cases around knowledge graph are kind of centered around three main things. One of them is data integration, you said to hide the nasty layer of the data. And I know that when you look at things like the data centric architecture and everything that's been written about that at the comms, I think there's a big aspect, an exciting opportunity here to leverage all the amazing work that's been invested in knowledge graphs and RDF as a way to solve some of our hairiest data integration challenges. And actually, Juan and I were back channeling a little bit even during this interview talking about, " Oh my gosh, what would a world look like if data integration was easier?" So data integration, one key thing, right? Second key thing, capture the knowledge of an organization and make it accessible, what you called or the democratization of data. I think today knowledge is thought of as fleeting and it's more, we think about Wikis and documentation or the conversations people are having in email and Slack conversations, and we need to be thinking about, and there's exciting opportunities and examples of knowledge graphs being leveraged to represent the knowledge of the organization, organizing it and allowing you to leverage that in a much more impactful way. You said, " Graph queries are the answer to the question, how do I get there from here?" Which I think is a great way to think about how graphs are democratizing the kinds of questions that you want to answer around your company's knowledge, around your organizational knowledge. And then third, you mentioned digital twins, which are sort of a special case of a knowledge graph, a digital facsimile of a real life physical phenomenon. And whether you're applying it to things like internet of things or supply chains, there's a lot of flexibility and power that knowledge graphs offer that allow you to capture what you said, the inherent diversity of the real world. So I think those are three excellent use cases. I know we're doing a lot more with knowledge graphs now, even as you look to the world of generative AI and some of the interesting use cases of combining together knowledge graphs with generative AI. But a lot of that has to do with taking the knowledge of your organization, the knowledge of the digital physical world and how they're connected, and data integration and bringing it all together. So I think those three use cases hold true, even if it's agents and AI that's leveraging that information into inaudible.
Ora Lassila [00:51:56] Absolutely.
Tim Gasper [00:51:58] And then last but not least, around adoption. You said, first of all don't quite understand that question, but second of all, there is adoption around knowledge graphs. There is interest, but there's more work to do. And the true victory lap might be when people are building applications and there's no novelty in saying, " And by the way, this was a knowledge graph powered application." Like, ooh, right. At the point that that's not interesting and novel anymore is when we've truly entered a new realm.
Ora Lassila [00:52:31] When people build things, nobody says, " Oh, and this is a relational database based application." Nobody says that. I haven't heard of that.
Tim Gasper [00:52:40] So that's victory, right? Graphs aren't new, and there's so much opportunity here. And last but not least, Juan you asked, or a question that Omar and you have talked about around the knowledge economy, or you posed, there is a lot of exciting opportunity around what might shift as we move to more of a knowledge first world, as we maybe introduce this idea of a knowledge economy. What if there were knowledge products? What if there was a business model where you could actually sell that knowledge to others? This could allow a new vision and expansion of what's possible around the semantic web. So definitely something very interesting there.
Juan Sequeda [00:53:21] So how did we do? Anything we missed?
Ora Lassila [00:53:24] What's that?
Juan Sequeda [00:53:24] Anything we missed in our discussion?
Ora Lassila [00:53:26] I think it was good. There's always more, right?
Juan Sequeda [00:53:30] Well, one thing I really liked about was we talked about the use cases, data integration capture, the knowledge of organization. The digital twin is that perfect mix of both. You need to integrate data coming from sources and that part of that integration is not just the data but the knowledge. All right, we got to wrap up before they're kicking us out of this room, because we're supposed to leave here in less than three minutes. So quick, what's your advice? Who should we invite next? And what resources do you follow?
Ora Lassila [00:53:57] What resources do I follow? So honestly, I don't have time to read all this stuff that's like the what's new today kind of stuff. There's so much old stuff I don't understand that I'm still reading that stuff. I tend to be the kind of person who has to go way, way, way deep to claim that I actually understand something. So, which usually means I have to write some software that does it. That, to me that's like understanding something. So I talk to people a lot. So a lot of my information sources are kind of like word of mouth. And then I go and I go deep. Who should we invite next? When should we invite some academic next, and see what's going on, what's coming over the horizon for enterprise?
Tim Gasper [00:54:54] All right.
Juan Sequeda [00:54:55] Well, Aura, thank you so much. We went through, I think most of the questions that people wanted. We were grouping them together. Quick reminder, next week Tim and I will both be live in Gartner. We're going to be actually in London all next week and we are going to figure out... Well, we're going to probably have a live moment. I don't know, we're going to figure out next week. But the coolest thing is that next week, it's actually our four- year anniversary of doing Catalog& Cocktails. So folks listening to us, we are going to be in London. So Friday, next Friday the 17th, we're going to do a happy hour. So for folks in London, let's go hang out and let's go celebrate doing this podcast for four years.
Tim Gasper [00:55:34] Can't believe it's been four years. It'll be a lot of fun.
Ora Lassila [00:55:36] That's amazing.
Juan Sequeda [00:55:37] And with that Ora, thank you so much. Thanks Tim.
Tim Gasper [00:55:39] Thank you.
Juan Sequeda [00:55:40] Cheers.
Tim Gasper [00:55:41] Cheers.
Special guests
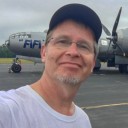