Speaker 1: This is Catalog and Cocktails presented by data.world.
Tim Gasper: Hello, everyone. Welcome. It is time once again for Catalog and Cocktails presented by data.world. It's an honest, no- BS, non- salesy conversation about enterprise data management with tasty beverages in hand. I'm Tim Gasper, longtime data nerd, product guy, customer guy at data.world, joined by co- host, Juan Sequeda.
Juan Sequeda: Hey, Tim. I'm Juan Sequeda. I'm the principal scientist at data.world, and as always, it's a pleasure. We're here Wednesday, middle of the week, end of the day. It's 5: 00 PM right now where I am on the East Coast. I'm actually live from Atlanta while Europe in Austin. Today, we have an awesome show today because we're meeting today with our guest, Alex Vayner, who is the managing director of data science and AI at KPMG. What was super awesome is that we met at the MIT CDOIQ conference last year, and we really hit it off, and I think it was the honest, no- BSness of things.
Alex Vayner: That's right.
Juan Sequeda: Cheers. How are you doing? Glad to have you here.
Alex Vayner Yeah, glad to be here. Thank you for inviting me.
Juan Sequeda: All right, so let's kick it off. What are we drinking and what are we toasting for?
Alex Vayner: Oh, yeah. Well, toasting is... I'm Eastern European, so I grew up in the tradition of making toasts. I guess maybe let's toast to customer.
Juan Sequeda: To customer?
Alex Vayner: The customer. Peter Drucker said the purpose of a business is to create a keeper customer.
Juan Sequeda: I like that. What are you drinking today?
Alex Vayner: What I'm drinking is a Lagavulin 16 Islay Scotch neat just because life is pain and suffering, and this is the best representation of that in liquid form.
Juan Sequeda: No need to complicate any more than that. How about you, Tim? I think you're in the similar vein right now.
Tim Gasper: I'm on the same page with Alex here, maybe a slightly more optimistic, but I am drinking this Springbank Single Malt. It was distilled 23 years ago, and it is very wonderful, so cheers. I'll say cheers to customer as well because being the chief customer officer over at data.world, that really resonates with me, so cheers to that.
Juan Sequeda: I'm drinking what's called here an old irv. Imagine an old- fashioned and a mule had a baby, so it's like right whiskey and ginger beer and bitters. It's actually pretty refreshing, so something very easy to go do at home. Looking forward to having more of these, and yes, cheers to customers. I remember it reminds me of what is an organization. It's a human endeavor where there's people who get together because they have a common goal that they want to accomplish, and then there's people on the other side who you want to accomplish that goal for, so yeah, to people and to who are customers. Cheers.
Alex Vayner: Cheers.
Juan Sequeda: All right, so we got our warmup question. Today's episode is all about the DNA of a data organization. What is one DNA trait you think would be awesome to have?
Alex Vayner: I don't know if this is technically a trait, but the thing that I've been after the most is resilience. I think that in today's world, when you're beaten down every time or there's a new technology up and around every time that forces you to learn and then to fail, resilience is becoming really the number one trait for me, so resilience.
Juan Sequeda: I like that. I was thinking, I don't know, is it some crazy, almost superhero type of stuff, but now that you said that-
Alex Vayner: I know. That is a crazy superhero stuff.
Juan Sequeda: Yeah. Well, I always talk about the balance between efficiency and resilience, and I think we always over- focus on being efficient, but we need to be more resilient in this world, so I'm with you on that one.
Alex Vayner: Yeah, so when I became a parent, this is about 15 years ago, I thought about what's my mission for the kids? What I defined for myself was that I was going to focus on raising resilient problem solvers, so resilience has been around for me for a while. That's the motto.
Juan Sequeda: Resilient problem solvers. Love that. Tim?
Tim Gasper: I thought resilience was great. I think my serious answer would be along the same lines, patience. I think if patience is something that you can have and pass down, then I think that's great. My less serious answer would be, can you be born with muscles where you never have to work out and you just have them?
Juan Sequeda: All right, but that's a good one too. All right, let's just kick it off. Honest, no- BS, what should the DNA of a data organization be, Tim?
Alex Vayner: Yeah. No, I think that's the problem to tackle. I guess maybe I would start with the head of the organization, the chief data analytics officer. I think that the noise that you hear a lot is the high turnover of chief data and chief analytics officers, so that's one of the things to tackle because if you don't have one consistent leader, it's very hard to have any kind of a structured well- run machine that is the organization. I think even Tom Davenport, who is the oracle of analytics, wrote an article, I think, a couple of years ago on this, and he had a perspective, which I think boiled down to it's a very new role so it's hard and the expectations are very high for chief data analytics officers, so it's the big takeaways, but I've been thinking about it for a long time, and for me, it's so much more multifaceted and complex than that, so I think that would be a great thing to talk about. I think that probably number one thing that jumps to mind is even defining what is the problem, so you've probably heard this at least, as a mathematician, defining the problem well is half the battle, so is it a problem at all? One of the things I remember being struck by is that, forgot where I read this, but it was a statistic that if you look at the S& P 500 in the 1950s, the average age of a company was about 60 years old, and if you look at it now, it's about plus or minus 15, so the average age of a company in S& P draw by a factor of four. If you think about tenure of executives, and even more so tenure of just regular data scientists and data engineers, you can't really have higher tenure of the company. Just the math doesn't work. If the average tenure used to be, you'd have three jobs in a career, 15 to 20 years, now the average tenure in general is divide that by four. Now, you're talking about three, four years, so it's all of a sudden, the average tenure for chief data analytics office, which is I think about 2.5 years, that's not so much off the average tenure period, and all of a sudden, it's a one sigma, it's not a five sigma. That's also a difference in perspective like, " Oh, okay. Yeah, it shouldn't be a little bit longer, but it shouldn't be three times longer," so that's the one thing that I was present to is just the idea of, " Wow, that's different." The world has changed and we woke up and said, " Oh, well, chief analytics officer's tenure is too short." Everything is too short.
Tim Gasper: That's interesting. I've heard that a lot before the S&P, 60 years old, now it's 15 years old. The rate of change and turnover within companies and innovation, it's accelerating, but I haven't heard it applied to things like jobs before and people's roles in organizations. Do you think that's a problem? Is it a problem?
Alex Vayner: I think it's a problem. It's just not as big of a problem as we think it is. I think that it's reasonable to think it will get a little bit better, but I think that just referencing that and having the sense of, " Okay, it's a problem, but it's not the biggest problem out there," is a good point. I think the other one that, at least as I was reflecting on this, what that jumped out at me, and it resonates with what Tom was saying a little bit, is in terms of expectations. In this role, first of all, you have to assume this person does a very good job managing hub, managing to the CFO in the business, and being the translator of data and insight to business value. At the same time, you want this person... We're in a talent shortage time right now, we got the great resignation, we got quiet quitting, all of these forces that emphasize even today after all of these rifts that are happening in the last four or five months in the data space, it's still highly desirable. There's still more jobs than there are applicants. The question is how do you attract talent? If you want to attract data scientists, data engineer, data architects, you can't be the business guy because those guys who want to come and they want to learn from someone, they want a leader who's actually an expert in some aspect of data, so that's hard. Then the other part that shocked me as I deal with a lot chief data analytics officers that's usually who I work with as a consultant is that this incredible expectation that they should know everything, not only being able to sell to the business, but within the supply side of things, that's a demand. The supply side, knowing engineering, architecture, transformation, cloud, machine learning, all of the aspects of that, it's unprecedented. I don't think it's realistic. I think that's one of the challenges.
Juan Sequeda: Let's dive into a couple of things here. One is about the breadth of knowledge of what a CDO or CDAO have, so that's one thing I want to dive into, but before we go into that one, you talked about there's a lot of jobs, and also the people want to get those jobs, they want to be more into the technical side. If you're only talking about the business, they want to track those people, but this reminds me of the conversation we had a while ago with Bill Inmon, the father of the data warehouse. He made this great statement which was technologists have loyalty to the technology and not to the business, and that's why they start jumping from place to place. I get that I want to go work in a place where they have great technology, we're I'm going to go learn, but then going back to what we were cheering on and where's the customer, this great technology isn't always providing value to the customer you need to go do. I think there's some realism that we need to ground in and sometimes it's like, " Hey, guess what? The really cool things that you want to go do is probably not what this organization needs," and then everybody's just going off and trying to look for something that actually is not what's providing business value. In a way, it's where we're in a sort of a bubble, I would argue.
Alex Vayner: I would say, to me, that feels like a false dichotomy, the idea of technology or business. As a data scientist, what I want to do, I want to be around other data leaders and I want to learn from them, and I want to be part of the conversation, and I want competence. I want my boss to be able to say, " Oh, you are saying this model takes four weeks to build? I think that actually you are being too aggressive. It should take seven because you're going to spend three weeks just cleaning and structuring data first. What are you thinking even?", or I want him to say, " Why are you building support vector machine model? Why aren't you doing something like logistic regression that's much simpler or gamings clustering or whatever that is?" I want to learn from people. I want someone who understands how long and how something gets done. That's the part that's important to me if I'm thinking about being a data scientist. Now, there's different career tracks. If I'm thinking and being a business analyst, the business side of the equation is important to me, but you got to have somebody, if it's not the chief data officer, then it's their number two who brings in that data competence. That's what I'm finding is really resonating with people. When it's someone with an MBA who's never actually written code and never built a model, they take a look back and say, " Okay, is this really the person who's going to help me grow as a data engineer or a data architect, a data scientist?"
Juan Sequeda: Okay. I appreciate that balance right there because going back to the previous point of like, " Oh, it's so broad, the CDO role," you need to involve not just all the technology, which that by itself goes into so many different kind of aspects. It's the business side, which that itself also can go into so many parts, but then if we go back to the original question of what is the problem, how would you articulate the problem that the CDO, CDAO should be focusing on addressing?
Alex Vayner: For sure, the problem they should be focusing on is the value problem. How do I get to have data connect to business value and doing it as quickly and as effectively as possible? Probably, if I was to choose between supply and demand, between strong knowledge of data and data systems, and really understanding the business and being able to articulate what you're doing to support the business and business value, I'd probably put business value first and foremost, but then you have to quickly compensate for the fact that you may be lacking in the data and the text stock, and it may be a challenge for you to hire and retain great talent, which is as we know, one of the key problems today. That's the balancing act. You always have the supply and demand, and that's the tricky part of the CDO role. I think almost as tricky, maybe even trickier than the second part, which you may mentioned already, which is being a jack of all trades, understanding enough and maybe being a true master in one of the disciplines, let's say machine learning, but really understanding cloud and architecture and integration enough to peer competent. I don't think that's possible. If you look at other disciplines that stopped being possibly long ago. If you look at math, there is no one person who understands all of the mathematics of today. I think the last person in math who was able to do that, I don't know if you guys heard of Henri Poincaré, he was a mathematician in mid 19th century, early 20th century. He's most known for the Poincaré conjecture that was just proved 20 years ago, and it was an open problem for a long, long time, but he was the last person to have been able to say, " I understand all of contemporary mathematics from topology to number theory, to combinatorics, to analysis, to group theory, all of it combined." He could talk intelligently and read up on any paper. If you take a mathematician today who's an anthropologist and give him a number theory paper, they won't be able to follow past the first half a page, or they go to a talk, after the first five minutes, they're lost because the fields progress too far. Same thing is happening in data science except faster. There is no punk terrain in data science anymore. There is no one person who understands the depth because we're moving too fast. It's that ray curse where the law of accelerating returns. It's moving so fast that change in two years in data science is like change in 100 years.
Juan Sequeda: Yep.
Tim Gasper: Yeah, it's becoming harder to keep up with everything given, again, the rate of innovation and change, and just the breadth of everything that's happening now. When you were just mentioning about having some depth maybe in one thing, but also being a jack of all trades being dangerous across a variety of disciplines, I thought of a T- shaped person. Is the CDO as the best DNA for a CDO to be this T- shaped person, maybe even a comb shaped person if they can have some depth in multiple things, but now you're starting to get a little bit more unicorny, at least a T- shaped person?
Alex Vayner: I think so because you've got to have at least some competence in some aspect of data. I just don't think that the data scientists and engineers will respect you if you're just a business person. You might be able to get the business side and get them enrolled and get them to prioritize things the right way, which is a big challenge. Getting that ROI, it's a huge challenge, and a lot of the people that are data oriented don't know even how to think about this, but you got to be good at some aspect of the data ecosystem in order to have the respect, I think, of the people so they can say, " Okay, I'll follow you as a leader because I know that you have distinctions in the data space."
Tim Gasper: I think that's actually some interesting advice because I think sometimes when folks feel like, " Maybe I only have business experience," or" Maybe I only have this horizontal experience," sometimes they think that, "Oh, I have to just continue to boil the ocean when it comes to my skills and just stay at the high level and never go below the water." It sounds like you're saying that maybe you don't know data engineering and so maybe that's not your specialty, but maybe you can get a little deeper in data science and now even though you only have one area that you've built some expertise in, you're going to be able to command more respect now because, sure, you don't know data engineering, but you know some data science so you can talk the talk there and you can provide useful recommendations, and that actually makes you more respected across all the disciplines.
Alex Vayner: That's right. It just grounds you. Somebody can connect to you and it just gives you a lot of credibility. I think that that's a part that's missing.
Juan Sequeda: Also, something I remember a long time ago, we had a conversation with Mohammed Aaser who was a former CDO of McKinsey, and he had all these different types of CDOs, which is are you the ones about security and compliance, are you focus all on your thing about security and compliance, or are you the one about the data entrepreneur like, "I have data. I want to make use some value out of my data, generate insights and monetize it"? Am I the one who's about the culture and democratization, so making sure I'm enabling everybody to do this? I think it also depends on what the phase of the company is, what they're trying to go do. What does success mean from a business perspective? I think there's a lot to consider on the CDO. One thing we were talking before was how would you compare where CDOs and CDAOs are today with respect to the evolution of CIOs?
Alex Vayner: Yeah, that's a great question. CIOs got off to a great start in the'80s. That's the birth of the CIO. Then in the early'80s, you already had hundreds of them, and now we have tens of thousands of them. If you think about it, from now till mid'80s, that's 40 years of development. I think that we are maybe five years in with chief data analytics officers, so we got lots of room to grow. I don't think though that... Again, the Kurzwell thing, the progress is much faster now than it was 20 years ago so it's even more challenging. I expect that our evolution will be even faster, and the role and the expectations for it, I even hire, so that's the challenge. Lessons to learn from CIO, for sure, and right now I think at least a third of the chief data analytics officers that I talk to report into the CIO, and that's also part of the challenge, right? The CIO doesn't consistently report into anyone. Some of them report into CFO, some of them report to CEO, some of them report-
Juan Sequeda: To COOs.
Alex Vayner: COO, right. Now, the chief analytics officers now, some into the CIO, some into the CFO, some into the president of a business, some into the COO as well. There's a lot of complexity and there's no one framework that says, " This is the best path for companies to follow."
Juan Sequeda: This is something I've been asking now, and actually, for folks who are seeing us that we're in this very intimate candle- lit room because we're at the honest, no- BS dinner we're having here in Atlanta today, we've been doing several of these dinners, and something I've been asking everybody is who do you report to? Just to go because it's very interesting to see people's like, " Oh, you report to the CFO, you report to the COO?" It's like people don't even realize, and then the conversation gets really interesting. You work with so many different customers. What are the patterns that you're seeing of reporting? Where does data end up reporting, and is there a way that you could say, " Oh, this reporting path is the one for most success," or something? Is that even possible to find that playbook or?
Alex Vayner: First, the answer is there's not one simple consistent answer even within an industry or even within a company size. If I say, " Look, I'm going to focus on a one to five billion dollar company versus a 40 plus billion dollar year," or" I'm going to focus on consumer goods and retail versus financial services," still all over the place. Here's the only thing that I've observed to be consistently, not always, but a thread where the data analytics organization begins. Usually, wherever it lives, it begins with a leader and it's completely centralized. It's a good thing in the beginning because you want some consistency in your tech stack, some consistency in your trainings, some consistency in your data. As it grows, it usually realizes and it matures that can't keep up with the demand. The demand from the business moves too fast, so what they've become to do is begin to decentralize and begin to spread, which is a fantastic thing. That's exactly what should be happening. You can see decentralization is just almost like a universal force. You can't stop it.
Juan Sequeda: The moment you're decentralizing is because you're growing, you're maturing, you're getting better stuff.
Alex Vayner: Yeah, and now you get concepts like data match and data fabric, and data products come up because that's how you empower your business partners to go and build their own products, to build their own solutions, to build their own dashboards, all of those things. That trend is happening. I think that if you are seen.... I teach, generally speaking, here's a cost center, and what you want your analytics organization to be is a value driver, so if you're part of IT, the value prop is I'm going to help you, the CIO, move from being a cost center to a value driver. What a fantastic story. If you are not, that means that you don't even have the part that may weigh you down. Now, there's a cost to that because the architects are still going to be in IT, the cloud team is still going to be in IT, the C- cell and security team is still probably going to be part of IT, and you're going to have to work harder to make sure that that team is really connected to what you're doing. The thing that's consistent is that evolution from centralized because you got to be one core and you got to have one machine that works well, and you're going to have data dictionaries, you're going to have an ontology set, you're going to have all those things, and then you begin to share that and you begin to grow, and then it's perfect because then you can actually start upskilling the broader organization. Now, everyone's data, and now the core team focuses on less. They focus on some aspects of data governance, perhaps. Perhaps, they focus on R& D. At the end of the day, you want your cutting edge development probably done within the people that are not using low- code solutions, but actually writing Python code that can scale. That's a good thing, but really a lot of the functions get democratized and get out into the business, and that's a great thing.
Juan Sequeda: Now, a lot of great nuggets that we need to go dissect that we're going to have in our takeaways, but one thing that came up to me was understand if you are in the cost center or understanding you're in the value creation, I think that's going to really change the way you speak, how you're going to convince people but...
Tim Gasper: I like that observation as well. A lot of times, the CIO organization in the company may be thought of as more of the cost center, and so we always hear this, "If the CDO reports to the CIO, then that can cause friction," and things like that. I thought you said that interestingly, Alex, that analytics is a value driver so you're implying that if it's part of the CIO group, then we need to recognize and embrace that that CIO group is going to be not just a cost center, it's going to be a value driver organization, so I thought that's interesting.
Alex Vayner: The truth is that this is not to dump on the CIO at your organization. I'm a big fan of Peter Drucker, as you guys probably picked up already. Peter said a while ago that business has two functions, marketing and innovation. Everything else is a cost center. It's not just IT. Literally, everything is a cost center except for innovation, that's create new product, and marketing, make that product accessible to the customer, and hence create a customer, so that's it. The idea is what should analytics be focused on? Well, it should be focused on innovation, creating new products, and marketing, getting new customers.
Juan Sequeda: This is another thing where should the data team, if it's going to start even in smaller companies, I'm like, " Oh, there's the first data team. We're going to do that." Who should you go work with first? Marketing. Always marketing. This is something-
Alex Vayner: True.
Juan Sequeda: This is actually a good conversation. I kind of shift this. We started from the top, right?
Tim Gasper: Let's go from the bottom. We talked about the DNA of the top part of the head of the organization around data. What about from the bottom? You're a data scientist, you're a data engineer, now what? What sorts of DNA should we expect there? What sorts of roles are most important there?
Alex Vayner: I started thinking about this question a lot more in the last four or five months because I started seeing riffs across the board happening, and then friends from my network, from companies that I worked with before reaching out and said, " Hey, I'm looking for something new." I started thinking about, so what are the different roles? What are the paths? I thought back to my grad school. When I was finishing grad school here at Georgia Tech in Atlanta, I had aspirations to getting a PhD, and then towards the start of my second year, I realized that that's not it, so it was clear that I'm going to finish second year and get my master's and leave. The only thing that was clear is I'm not going to be in academia, and that was it. The world was basically binary. There's academia that does a one, and then there's everything else that's a zero. I felt like I was selling out, of course, and disappointment to my advisor at the time, may he rest in peace now, who's a fantastic professor at the Georgia Tech, Richard Duke, but I did my master's thesis and I left. I remember applying to... It was a second thought. It was like, " Okay, I guess I'm going to go to Korea bars," and I dumped my resume in and I got a bunch of interests. Then I remember getting an offer from a consulting company, from FedEx, to be an operations research modeler, just doing optimization, which FedEx needs a lot of, and from Oracle. I got a big tech, I got corporate America, and I got consulting. My calculus for making the decision was like, " Okay, who's going to pay me most?" If I'm going to sell out and not be in academia, I'm just going to go to the highest bidder, and I did. Now, what ended up happening is I went to a fantastic strategy consultants called Mars and Co. It's a spinoff from BCG from about 50 years ago. In two years there, I got the best business education I could possibly dream of coming in as a mathematician and computer scientist, not really understanding even what is ROI, what is business, what is EBITDA, what is a inaudible. These all were foreign terms to me because I mostly spent my time studying combinatorial algorithms. It just now, as a reflection on that, I started thinking about, " Okay," and so I created these six categories of, I think they were professional services, corporate, then financial services because that's a huge machine of itself, then startups, government, and NPOs, NGOs, and I think I'm missing a category here. They will come to me. I think there was definitely a sixth one. Oh, big tech. Those were, I don't know, a six... This is not the truth or anything. You could create seven or nine or 27, but those were the different options. I started thinking about, okay, these represent fundamentally different experiences for data scientists or an analytics professional, so if you are working... My love is consulting. I spent at least two thirds of my career in consulting, and I love it because it's fast pace. I love it because it gives me a lot of different experiences. Every three, six, nine months, you got a new project, which means you have a new client, new industry, new project manager and partner, new team that you're a part of. Everything is new so that appealed to the ADHD nature of me. Just change, always change, and then you're always learning. You're always learning new things, new industries, new companies, understanding new technologies, so that was huge, but there's a cost. The cost is you are working 60, 70, 80 hour days. The cost is you travel all the time, so maybe if you're starting your family and you're a primary caretaker, this may not be the ideal path for you. Those are the considerations that I never thought of. I thought about things like what is the risk here? The risk of being fired. What is the environment culturally? Is it structured, a lot of clear paths on what you're doing while you're doing it, or is it unstructured? Is there depth of learning like, " Hey, I'm going to do NLP and that is all I do"? If you are in consulting and you just want to do computer vision, it's going to be very hard for you as a data scientist because you could be in there are a few people that are super specialists, but usually, if you're a data scientist, you might be doing logistic progression model today, in an NLP model in Python tomorrow, and a fraud analytics model the day after. Those are all things that's part of your regular year, but if you go for work for big tech, so you go work for Microsoft, that's very unlikely to happen. You're more likely to be on the opposite side. You're more likely to be highly narrowly specialized because they have tons of PhDs, and there's no reason for them to have people that do NLP and computer vision in the same camp. They'll have a thousand people just do doing an OB and they'll do have a thousand people just doing computer vision, and that is all you'll be doing. If you have a PhD and you want to specialize and you want to do one thing, it's a much easier fit into the big tech world versus the consulting world. Those are some of the considerations I started thinking through in a startup, completely different space. Again, you can be a data engineer and architect and a data scientist all in one in a small startup, potentially. That's a possibility. That will never happen in big corporate today or in big tech. You're going to be one or you're going to be the other. You can have a ton of breadth and you can have a ton of depth in a startup. That's the beauty of a startup is you're wearing lots of hats because it's small, so they want a little bit more of a unicorn. Big tech doesn't hire unicorns anymore. Big tech hires hyper specialists.
Tim Gasper: Yeah.
Juan Sequeda: This is-
Tim Gasper: Alex... Oh, go ahead. Go ahead, Juan.
Juan Sequeda: No, you go. You go, Tim.
Tim Gasper: Yeah, I was going to ask if you are... It's interesting to hear from the perspective of like, " Oh, if you're an academic, here's where you'd might find a better fit for you. If you want to try a bunch of stuff, then maybe a startup is a great fit for you." What if you want to be a CDO someday, right? If you were going to design the perfect path, what path... Do you recommend somebody start on the ground floor as an analyst? Do you recommend they start in the business and then change careers 10 years in? What's the perfect path?
Juan Sequeda: Well, this is a fascinating question.
Alex Vayner: Yeah, that is a strong question. Let me give you my jaded answer first because I'm a Eastern European sort of hyperrealist pessimist, and so that part of me-
Juan Sequeda: Sorry, Eastern European hyperrealist pessimist?
Alex Vayner: Yes. That's-
Juan Sequeda: That is so good on a t-shirt too.
Alex Vayner: I was born in Moldova, which is one of the 15 Soviet Republics, so in my experience, there's a psychological theory that says whatever you experience in the world when you were 9, 10, 11, that is embedded in your brain as how you perceive the world. My 9, 10, 11 is standing in breadlines and life is hard, so that's the hyperrealist pessimist side of me. If you want to be a chief data analytics officer, I think you should be in corporate because corporate will arm you for not only the technical skills which are important, but the political, environmental, cultural, structural skills that make for a chief data analytics officer. You'll learn that. That's number one. Number two, when some company Heidrick& Struggles or Egon Zehnder, or replace it by one of those top executive retained search firms that usually look for a chief data analytics officer or any senior executive role, they will say, " Okay, I'm looking for a corporate leader. Does this person have a corporate track record?" I guarantee if you've spent 20 years in startups and you want to be the chief data analytics officer of Coca- Cola, it's not happening. It's not. They're going to look at you and say, " Do you even know what it means to be in senior executive in an organization with 100,000 people in it?" No. Okay, so I think that if you spend your entire life at Microsoft, or Apple, or Google, they'll say the same thing like, " Yeah, he's probably a fantastic technologist, but does he know how to run a team in corporate America that is not pure tech, that is consumer goods, or manufacturing, or financial services?" Very different. I think that what kind of chief analytics officer do you want to become? Do you want to become that person in corporate America? Then go into corporate America.
Juan Sequeda: You're saying that chief data officers really don't exist in the big techs. They don't exist, or startups and-
Alex Vayner: They exist. Who is the chief analytics officer of Google? I don't even know what that means. The whole company is analytics. What does that person even do? But if you say, "Okay, you are the chief analytics officer of UPS," that's a company here in Atlanta, " Oh, I know exactly what that means. Okay," because they deliver packages. They don't build data.
Juan Sequeda: That's a very valid point and I completely agree with it, but now, there's still data work, data teams in startups, in these big tech stuff, so where do they fit inside of that organization?
Alex Vayner: Now, you're getting into nuances. First of all, all the big techs are getting sectorized. Nobody's just data. If you go to Microsoft in their professional services, they have a healthcare team, they have a financial services team, both on the product side and on the professional services side. What that means is that there, if you're becoming a leader in that team, you have experience selling into, let's say you're in healthcare, you have experience selling to payers and providers. Oh, that's interesting because now when you come to interview with, let's say Blue Cross Blue Shield, you can talk very intelligently about what do providers need, and how does it work, and what are the data sets, what are the security concerns, what are the BII issues? All of those things, you'll be very credible, but I think the more traditional path is... I'm not a chief data analytics officer. I'm a managing director in a consultancy. Now, you could say a lot of what I do is I work with chief data analytics officers, but I think that the skillset besides understanding of analytics and data is very different in a consultancy than it is in corporate, yet still you see people move to and for, right? It's not unusual for somebody to spend 10 years in the industry, and then come into consulting, and then go back and do this, and I've done this myself.
Tim Gasper: Just to go back a little bit to the bottoms up piece because I think this is a very interesting conversation where we talked about the CDO and the path that you can get to it, and so what is the best way to organize the bottom up part of the data organization? Should there be the data engineering group, and the data science group, and the analyst group, or should it be... You talked a little bit about sectorized, I think especially larger organizations that have more decentralization going on. You see more of, " Hey, let's sectorize and have the expertise more embedded," and things like that. Is there a framework that you think about as not just the CDO and their DNA, but the DNA of how the broader data organization needs to look?
Alex Vayner: There are clear structures and teams that, I think, are pretty well known. I don't think I'm going to innovate by saying, " Okay, you need to have a team that focuses on data governance and data management, and you're going to need to have a team that focuses on your integration with cloud and data integration team. You need to have a team of AIML experts that are actually building models. You need to have a team that focuses on reporting visualization because that's how your insights get consumed by the business. You need probably to have a team that focuses on actually being the liaison to major businesses and domains." Usually, in traditional American corporations, there are at least two, three, four different divisions that are focused on different products or services, and then their functions also, marketing, finance, supply chain. You may want to have, especially within your data science and IML team, people that start focusing on those major domains. You also want to have people that are part of your, what you can call, go- to market operations team that focus on being the liaisons to marketing, supply chain, finance, capability- wise, presidents or senior executives of various businesses because the idea, I remember, is that as you mature, you want more and more of your organization to move into the businesses and into the functional domains like marketing and supply chain, and to consolidate your team. When you're in your year three, four, five and you're in what we call mature embedded analytics organization, you have very little dashboarding people on your analytics team because you don't need to run that many dashboards. As an analytics team, guess who needs dashboard? The CFO needs to see what's going on financially. The chief marketing officer wants to see how his marketing mix model is actually playing out and how his ROI on different parts of the marketing dollar is coming up. The supply chain officer needs to have a dashboard that maybe pops up when there's a problem in distribution somewhere or there's an inventory problem. Those things are needed in the business or in the functional domains, so why have them in your analytics team?
Juan Sequeda: That was a very beautiful setup that you had there, and I'm really happy that you brought this up, and I think this is something that we need to highlight. This was minute 40 of the podcast to go listen to. It's really, really nice. You need to see all the notes that we have, but we could keep talking about this for hours. Luckily, I am going to keep talking for hours with you after this because we're going to have dinner, but all right, we need to move on. Thank you so much. This has been a fantastic conversation. We're going to move on to this other segment that we forgot to do last time, but we're going to bring back the AI minute. See, we didn't talk anything about AI. You have one minute to rant, talk about anything you want about AI.
Alex Vayner: Sure.
Juan Sequeda: Ready? Go. I got to...
Alex Vayner: All right. Well, I think that we are hearing a lot about ChatGPT and a lot of noise around that. I think that my rant is really welcome to the club. I think things are only going to get worse in the sense that we are going to have a lot more new things come up that completely disrupt our understanding of AI, our understanding of business, our understanding of the world. This is the ray curse while drop date units and law of accelerating returns, and we are just getting started here. I think that brace yourself, and I think that the motto for the next year at least is just get comfortable with being uncomfortable. It's uncomfortable. Now, business is asking questions. What are the implications of ChatGPT on the business side? Nobody clearly knows the answer, and to presume that anything else is true is to delude yourself.
Juan Sequeda: Perfect. One minute. That's actually a great point.
Tim Gasper: It was, yeah.
Juan Sequeda: It's time to get comfortable being uncomfortable because there's so many open questions right there, but all right, Tim, lightning round questions. Are we ready to go?
Tim Gasper: Let's do it.
Juan Sequeda: All right, I'll go first. If you can't have a CDO with both business and technical, could you start with the technical CDO who can learn the business on the job?
Alex Vayner: Yes, absolutely. Yeah, you can't coach height, right? The height here is tech skills. It's much easier to get smart and understand how the business works and start doing things like putting together guesstimates on return on investment for particular solution than it is to start coding in Python.
Juan Sequeda: Perfect.
Tim Gasper: Yeah, that's a great answer. Second lightning round question. Should analytics and the data office be bundled? AKR, are you a fan of CDAO?
Alex Vayner: My intuition says yes because that's the supply and the demand, and you don't want to separate the supply from demand. When they're separated, it's going to hurt. It's going to hurt because you're not going to build the right thing or you're not going to build it right, so neither one is a good option.
Juan Sequeda: All right.
Tim Gasper: That's an interesting analogy there. It's separating engineering and product management. If they're too far away from each other, weird things happen, right?
Alex Vayner: That's right.
Juan Sequeda: All right, next question. Is it better to have a few unicorns on your data team or 10 more specialized team members?
Alex Vayner: Depends on which team. If I'm running a startup, I'll take the two unicorns. If I'm running a big organization, it's going to disrupt everything because people are going to get jealous of the unicorns because there really are unicorns, there are very few, and I don't have as much utility for them. I have very clear structure around who's doing what fine, but if I'm starting a company, I will spend a 10x on hiring a unicorn. I've hired a few. I know exactly what they look like. They are rare, but when you hire one, oh my God, the ROI is through the roof.
Tim Gasper: Great answer.
Juan Sequeda: Wow.
Tim Gasper: All right. Fourth question, final question. Will generative AI be a net simplifier and accelerator for data teams or not?
Alex Vayner: I feel like the right answer is, yes, it will be, and it will not be. It will simplify a lot of things that we are doing manually right now, but it will also create a lot of issues. We are seeing already ethical AI and all of this stuff, and now how do you teach your college course to... How do you check that a legal essay was not done and was not plagiarized? I think that it solves problems that creates problems at the same time, which is great. That's progress, right?
Tim Gasper: It is.
Juan Sequeda: All right. Takeaway times. We had a lot. Tim, take us away with takeaways.
Tim Gasper: All right. Well, we started off with the DNA of the top part of the organization, and we talked about the CDO. You especially, Alex, started off by talking about the expectations and the challenges and the turnover that we experience at that level. It's a new role. Relatively speaking, expectations are high, and you used that analogy of the average age of the S& P companies, they were, a while ago, it was 60 years, now it's 15. Similarly, maybe you might expect a CIO or something like that 20 years ago, 30 years ago, to stay at the company for 10, 15, 20 years. The CDO, I think, they had latest date is an average tenure year of about three years or so. Obviously, things are changing. Some of that is to be expected, but some of that is undesirable. It definitely makes things challenging from a resilience standpoint. When we looked at the CDO, we also talked about what's our goal, and you really mentioned that it should be business value first. If you have to focus, you need to put that focus and put it on business value, and put that at the center, and the technology comes after. Obviously, the two together is best, and I think that was a theme also that we talked about today, but if you have to pick one, it's business first. Expectations. You got to turn data insights into business value. You have to know enough to be dangerous, but you want to have some depth. We talked about this T- shaped person, and you described having one of these deeper skills in a particular technical area that can really help you to build respect in the organization and to be able to answer those types of questions like, " Hey, I want to use this deep learning model." " Oh, do you really need to use a deep learning model for that? I really think that can be a logistic regression." I think that ability to command that respect and navigate those kinds of conversations ends up being important. You mentioned that technology versus business is a false dichotomy. You want to be able to try to address both of those. Somebody needs to be bringing the data competence, and if it's not the CDO, then that person needs to report to it. You mentioned that the CDO, where's the best place for them to really report? There's no ultimate answer for that. It definitely depends on the organization, but if you want to have consistency in your data, your systems, your training, your dictionary, your ontology, then it is good to start in a centralized fashion and grow from there, and then figure out how to decentralize over time. I know, Juan, you probably have some more takeaways about that in a minute, and that you should really think about is this person reporting into a value center or are they reporting into a cost center? If you were saying that, " Hey, analytics is going to be a value driver for the organization, and then they're going to report into a cost center," then you got to recognize that either you're going to set them up for problems or you need to change some of the mandate in the way that you're thinking about your CIO organization or your CFO organization or whatever it may be to be a value driving aspect as well, and so that's pretty important. Analytics, in general, is something that creates value for the organization. You mentioned Peter Drucker and how he pushed that really a business has two revenue centers, it's marketing and innovation, and so you connected that to analytics. Analytics should be focused on marketing and innovation. Juan, I'll pass it over to you. What about your takeaways?
Juan Sequeda: I think that's one of the key things right now. Just if there's one thing to remember is you need provide value. If you want to go do that, it's through innovation, marketing. Innovation, new products, marketing is making those products accessible to the customer. So then we also talk about the bottoms up from the data engineers and data scientists, and I like how you categorize these different types of jobs. You had six categories, professional services, corporate, fin serve, startup, government NPOs, NGOs, and big tech. The reason why it's important to think about these differently is because they all have different mentalities, different modalities around how they deal with technology. You gave your personal example, right? In academia, you got out, did your masters, and you went to join a strategic consultancy where you learned so much about the business, and that's something you could have only learned at a consultancy around that. Think about it. In a consultancy, you're going to be jumping on so many different topics. In big tech, you're going to be specializing in the topic. They don't want unicorns. While startups on the other way, they want a unicorn. They're going to be hiring. They want somebody who can do the data engineering. The scientists wear lots of hats like unicorn. This is a great interesting question. If I want to become a CDO, what is my path to the CDO? Your very specific answer is you should be in corporate, right? You really need to understand the political skills because it's really corporate America, a corporate world who's going to be looking for CDOs, who's the CDAO of Google. I don't know. Everybody does analytics over there, right? A company's looking for CDO or people who have inaudible in corporate. Thinking about the bottom to teams, you talked about what do teams need to go do when it comes to data. You said specifically data governance, cloud integration, data management, modeling, data science, machine learning, reporting visualization, and having that liaison to the business domains. Then eventually, as things scale, then you have different aspects who start focusing those domains, so your data science machine learning will be focusing on domain, the reporting and visualization will focus on the domain, right? Over time, that decentralization is really a manifestation of the maturity of the organization, and you start having embedded analytics all very part of it. Then that's why central teams won't have any more reporting dashboards teams, and they're going to be pushed out. How did we do? Anything we missed?
Alex Vayner: No, you guys did great. I feel very understood.
Juan Sequeda: Well, this was all you. We were just paying attention to what you were saying, and you had a great lot of insights in here. We're going to throw it back to you. Three final questions. What's your advice, who should you invite next, and what are the resources that you follow?
Alex Vayner: Sure. My advice is... I started doing series at KPMG for the younger analytics professionals in the organization, and it's also catered around creating your identity and connecting and networking within the firm to create value to help your career grow. The motto for that is watch your ass. This is the ass for the capital S. The three things analytics professionals that I think that I push to focus on is first S, is the sector. Everything is getting sectorized. The earlier on you decide, " I'm going to be a financial services guy," " I'm going to be a healthcare guy," or" I'm going to be a consumer goods guy, girl," whoever that is, that's going to be very, very helpful because that's getting premium more and more. Companies even like Microsoft, but even next layer down like Databricks, fantastic company that's in the compute space, they're getting sectorized. They're getting focused on specific solutions for specific industries. The second S is the hyperscaler. The world's in the cloud. Now, whether it's AWS or Azure or GCP, fine, but choose one, get certified, get good at it. That's going to be a key differentiator. You're not just a data scientist writing Python code or a data engineer. You actually know how to do this within a hyperscaler. The third S is what I call the subdomain, which is, within analytics, choose a specific function. You do fraud, or you do risk analytics, or you do NLP, or you do computer vision. Choose something you specialize in. That becomes a really a big part of who you are, and the wrap around it is that tell your dragon slain story, go network, go connect with people, and wrap it all around. Wrap the three Ss into one dragon slang story that tells the perspective customer, employer, or even an employee about how awesome you are and why it's worth getting to know you.
Juan Sequeda: That's fantastic advice three Ss.
Alex Vayner: Watch your ass.
Juan Sequeda: Watch your ass. Who should we invite next?
Alex Vayner: This is going to be a challenge. Hold on. The most interesting man in the world in my opinion is Tyler Cowen. He's a professor of economics at George Mason, and he has a podcast as well. He's an economist and he's just a brilliant, brilliant person. He's not a data scientist, analytic professional, but if you can get him on, I would highly recommend. I follow him religiously. This is leading to the next question what do I read or listen to, whatever. Yeah. Tyler's podcast is number one. I used to be a big fan of Eric Weinstein on The Portal. I don't know if you're familiar with him. He's a Harvard PhD mathematician that used to work for Peter Teal as an MD in his venture fund. Also fascinating. I listen to a podcast called Intelligence Square at least once a month. I think it's one of the most interesting podcasts that involves different topics from history, literature, politics, poetry. Not a pure data science source, but just something that I find fascinating. I read The Journal every day just because you got to know what's going on in business, and then I follow a bunch of... For technology and data, I don't have a single source. I think I use Feedly, and I just follow whatever the Feedly articles takes me to, and the sources are all over the place.
Juan Sequeda: All right. Well, this was a fantastic set of resources to go follow. Alex, this was a fantastic conversation. Just quickly, next week, our guest is going to be Ricardo Baeza- Yates. He's a research professor at Northeastern University. He's the past VP of research of Yahoo Labs. He's a pioneer in all web work. He wrote the book on modern information retrieval, so for only computer scientists out there looking about web information retrieval stuff, he wrote the book on that. He's a true expert in AI and a really good friend of mine. Next week is the web conference in Austin, which I'm the general chair of, and Ricardo's going to be there, so we're going to have a live podcast with him. He's been one of those loud voices about bias in AI and responsible AI, so it's going to be a really great conversation. With that, Alex, thank you, thank you, thank you so much. This was a phenomenal conversation. Cheers, Tim, inaudible tonight.
Alex Vayner: Thank you for having me, guys.
Speaker 1: This is Catalog and Cocktails. A special thanks to data.world for supporting the show, Karli Burghoff for producing, Jon Loyens and Brian Jacob for the show music, and thank you to the entire Catalog and Cocktails fan base. Don't forget to subscribe, read, and review wherever you listen your podcast.
Special guests
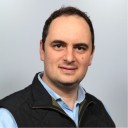