Tim Gasper [00:00:32]:
Hello and welcome. It's time for Catalog & Cocktails, your honest, No-BS, non salesy conversation about enterprise data management. With tasty beverages in hand. I'm Tim Gasper, longtime data nerd, product guy, customer guy at data.world, joined by Juan Sequeda.
Juan Sequeda [00:00:48]:
Hey Tim, how are you doing? It's Wednesday. It is middle of the week. Always a beautiful time to take a break and go chat about data. And today we are going to be chatting. One of the topics that are just goes back to our core of who we are and why we started Catalog & Cocktails. The cocktails part, I mean we all know we like cocktails, but the catalog is kind of comes from the data catalogs of the metadata space. And we're going to talk a lot about metadata and really excited to have Mike Evans, who's the Chief Innovation Officer at Amplifi. Mike, how are you doing, Mike?
Mike Evans [00:01:21]:
I'm really good, thank you both. Great to be here. Great to see you.
Juan Sequeda [00:01:24]:
Yeah. Well, we're super excited to get into the topic that is very dear and ear to our heart. But hey, let's, let's kick it off with our tell and toast. What are you all drinking today or what's your favorite drink and what are we going to be toasting for?
Mike Evans [00:01:37]:
Well, I'm, I'm drinking it's a non alcoholic mojito because I've got to, I've got to drive after this. But I'm, I'm going to toast. I'm going to toast to my wife's birthday which is tomorrow.
Juan Sequeda [00:01:51]:
Congratulations to your wife and always a fun time. I love birthdays. I'm the person who tells people, reminds people, hey, it's my birthday, it's my birthday because I just like celebrating. So Tim, what about you?
Tim Gasper [00:02:03]:
Well, it's, we're, we're doing this episode a little earlier in the day than usual. So unfortunately I don't have a true cocktail. I am drinking a delicious, luxurious bottled frappuccino which is from Starbucks. It is of the pumpkin spice variety. But I will say, you know what, what's a great cocktail I've been having lately? I've been experimenting with different ones. I recently discovered the Ward 8, which is a pretty, a Decent cocktail. So recommend folks checking that out and trying to make a war eight.
Juan Sequeda [00:02:34]:
Expand on that. War eight. What is it?
Tim Gasper [00:02:37]:
Yeah, it's got a rye whiskey, it's got some lemon juice and some orange juice and some grenadine in it. And it's just, it's just a tasty drink. Tastes good.
Juan Sequeda [00:02:45]:
Sounds nice. Well. Well, also. Morning. I'm still having my coffee, but actually one thing I did recently was doing some, a sour whiskey sour. Actually don't do that that often. And I'm like, I, I mean, because I was like cooking, I needed some extra yolks and I had the, the, the whites left over. It's like, oh, there's signs right now I need to go do something. So tried that and yeah, it's great. I love. Because one of my favorite drinks of all times is a pisco sour. So I think that that, that sounds very dangerous drinks, right? You drink one, one, two of those and like, oh, this is nothing. Then you stand up like, oh, what the heck? All right, well, we got a warm up question, which is what is something that you haven't extracted enough value from and it's sitting right in front of you.
Mike Evans [00:03:28]:
Well, I guess perhaps, perhaps slightly predictably, my answer is going to be for most organizations and I include like ourselves in that is the metadata that you produce from day to day as part of your lives, as part of your jobs, and almost every interaction that you have with any system or process in your organization.
Juan Sequeda [00:03:52]:
Well, that's, that's gonna be the topic. We're gonna dive a lot into that. Tim, what do you, what do you have right now? What is something in front of you that you're not extracting of value from?
Tim Gasper [00:04:00]:
I, I will go not, not pure data and I'm not gonna go pure personal. It's gonna be someplace in the middle. I haven't extracted the full value out of this nice microphone that I have. I should be creating more content and Juan should too. We should be creating more content. So that's some value that's not tapped fully.
Juan Sequeda [00:04:18]:
Yeah, well, I think part of that is like we just need more days. We're hours in a day, more days in-
Tim Gasper [00:04:22]:
We need 48 hours a day instead of 24. Right.
Juan Sequeda [00:04:25]:
Well, I think one of the stuff I have is, I'm on my desk right now, but it, it, it lifts. I mean it's, it's a standing desk. And I realize, I mean, just asking this question, like I have this stuff right in front of me, I'm not standing enough. And then I Complain like why? Why does my back should raise it right now.
Tim Gasper [00:04:40]:
Raise your desk right now.
Juan Sequeda [00:04:41]:
I actually, you know what? I'm going to go do that. So hopefully. But in the meantime, let's kick it off. Honest. No, yes. Let's start off with examples of untapped value of metadata and how does this connect this whole concept of active metadata? Yeah.
Mike Evans [00:04:59]:
Okay. So I guess maybe the first thing to think about when we answer that question. So almost to start with the second part of the question first, if that helps. So organizations everywhere, we create metadata all the time. So data about the data that we hold, and that could be things like the definitions of the data that you store or you care about as an organization, definitions of key business entities or attributes or things like that. But it could also be data about how that data is interacted with. So it could be this data has been accessed this many times, it's flown through this many integrations, it's been included in this many reports, it's been liked and approved by these people, or it's been rated as high quality by these people. All of that information. I think organizations are kind of getting better at acknowledging that they need to capture some of that information. And obviously we're talking about data catalog as a big part of this. And data catalogs, the value of data catalogs is being, I think, held in higher regard than Perhaps it was 10 years ago or so. But kind of capturing the metadata and exposing the metadata to people and to machines is one thing, but actually doing something with it is another. So that's kind of where we start to get this concept of active metadata. So active metadata, I like to think about it as just applying the same sort of analytic techniques that we'd apply to spot patterns in data, but applying that to metadata in order to inform things about our organizations and the way that they operate so that we can make real improvements to the way that we operate. And if I think about kind of examples of that, it's quite an abstract topic. I think I find it quite a difficult topic. Certainly when I first kind of learned about the concept of active metadata, it feels like something that's quite hard to get your head around. So why would I want to do that? But when you start looking at kind of real use cases where we monitor metadata in an organization and react to changes in that metadata, it's actually A, it's a bit kind of simpler to understand, but B, it's also probably a bit closer to home in terms of it's easy to spot quite simple use cases. That can be used for improvement straight away. So if I take. So one of the, kind of, one of the interesting use cases that's perhaps along the simpler end of what you could achieve with metadata activation is using metadata to kind of prevent failure or quickly remedy failures. So spotting patterns in data, perhaps in data quality. So we monitor this data that's coming in on a stream for, for against certain quality metrics. And we've certainly noticed that the level of quality has dropped from, you know, 90% of this field down to 70%. Okay, that indicates that some anomaly, maybe something's happened upstream that we, that we need to deal with before it starts to impact things downstream. Those are quite easy, easy scenarios to conceptualize. And yet they're, they're very powerful uses of metadata activation. So we're, we're using some metadata that we have and turning it into some action that we can take to prevent a particular scenario. When you kind of abstract that into kind of looking at all the metadata that you have in an organization and the kind of insights that it could deliver, you start to think about use cases where, okay, my metadata is telling me that we created all these load of great reports and we thought they were the best set of reports and dashboards that the organization needed and wanted. And we found out that no one's actually ever accessed them or any one person's ever accessed them. Very occasionally they're not being used, they're not being run. And so first of all, you present an argument for, well, the data is telling us maybe we should decommission these, or it's at least telling us that take, take some, some action on them. It might also tell us that loads of people are extracting data from other sources and doing some work in Excel or something to bring them together. So actually maybe we want to create some, some reporting assets over the top of that. So there's a, that's a bit of a flavor of the type of thing that we can do.
Tim Gasper [00:10:11]:
Yeah, no, I think this is interesting. So, Mike, I think you've, you've. So two things. Right. First of all is, you know, you mentioned, you know, the word catalog, right. Like people, people kind of have understood for a while now that collecting metadata is important. That importance is growing and I'm sure we'll talk more about that. But you know, from quality to AI to these different trends, compliance, which, you know, make metadata even more important. But previous use cases or sort of the traditional use cases around metadata have tended to be more. Well, we want to make sure that people can discover it, or we want to make sure we can organize it, or, you know, it's a little bit more taxonomical or librarian oriented or perhaps discovery oriented. Right. But what you're talking about is this sort of contrast between maybe that's more of a passive use case. Whereas, you know, what could you be doing with your metadata that inspires action, that inspires insight, that inspires efficiencies? You know, essentially, you know, what we wrote down here in our notes, kind of Juan and I, we're always taking notes when we do this, right. Is we wrote down metadata analytics. Like you're. Essentially, you're analyzing your metadata and trying to use that to spur action. Is that, is that kind of how you would describe what active metadata is?
Mike Evans [00:11:34]:
Yeah, absolutely. You. And if you think as well, of all of the different techniques that we've got and the tools that we have available to try and extract some insight from that metadata, you start to see kind of how powerful it could be. But absolutely, it's applying those analytic techniques on top of the metadata that we're creating. And, you know, it's a, in itself, it's a very broad term. Organizations generate vast amounts of metadata, and you can start getting really abstract about the, you know, what constitutes metadata and how much of it there is. And analyzing all of it is almost certainly, you know, overkill. But there are certain use cases that I think are kind of like almost obvious applications of metadata activation. And the fact that we're getting such advances in areas like AI make us, you know, put us in ourselves in a position where the, the insight that we can gain from the metadata is that bit more powerful.
Juan Sequeda [00:12:36]:
Yeah.
Mike Evans [00:12:36]:
So.
Juan Sequeda [00:12:37]:
So I was back channeling with Tim and I'm like, you know what? It really hit me and I can't believe I'm just realizing this now that all this active metadata, we're using your metadata. This is just metadata analytics. Right. We talk about data analytics. And I just did a quick Google search. I'm like, I just. For searching for metadata analytics. That's not a term people use.
Tim Gasper [00:12:58]:
People use active metadata. But then everybody's like, well, what is that?
Mike Evans [00:13:02]:
Totally.
Juan Sequeda [00:13:04]:
And then I won't name names. But, but, but people don't like that term active metadata. That kind of seems like you're discriminating metadata. Is it active? Is it passive? Is like, wait, wait, metadata is just metadata. Right. I'm just, but so that, that's just something that came my time. Like, we. It's just basically metadata analytics. And I think I'm Going to start saying that whenever we have that discussion with folks like Active Medical, all you're doing is just the way we do data analytics. You're just doing meta analytics. So guess what? Analytics, you're trying to do your data, you're trying to do that over your metadata too. Spotting trends and stuff like that. And you see those and that's going to inform you about what, how you can fix things that are in the past and hopefully also you can do some predictive things, maybe what you can do in the future. But okay, but I want to dive into one thing which is my, what I call my drummer. Oh my, my, my question that I always bring up. Or that how do we avoid boiling the ocean? Because this seems like this. They take the same pattern as Daniel Lakes is like, oh, just give me all my data and I'm going to put it into something and we have data scientists, we're going to come up with all these insights. Right? I mean this was the whole story 10 years ago. And then you can feel like, oh, just we just need to capture all the metadata and we're going to find. I call that success. For that's a path for failure. So how do we avoid boiling the ocean but in other ways is like what are those low hanging fruits to be able to kind of do analyze the metadata. And then there's. Sorry, there's so many questions here, but is there a, is there a template that we could use that is. That is there's like a low hanging fruit template that is applicable across all organizations or is it really the. It depends.
Mike Evans [00:14:41]:
Yeah, that does it. There's a, there's a few questions in that. Yeah, go on, go, go rigged that. The first point about boiling the ocean I think is it's a really interesting one because I think where we are now as an industry, active metadata or as we can call it metadata analytics, it feels to me like a much more intuitive term for what it is. I think if it had been coined with that title it would have been easier for a lot of people to understand in the first instance.
Mike Evans [00:15:17]:
If, if we, if we kind of think about what organizations should be thinking about doing today versus what they might be able to do in the, in the, in the future. Because, because a lot, a lot of these use cases are let's say, immature. There's not many examples of the bit them being deployed in real businesses yet there are some and there are some good examples along the lines of the, of the things that I described earlier. But there's, there's not, not vast numbers of them. And yet the principle of being able to activate your metadata and being able to gain insight from your metadata is something that I think has got to be incredibly valuable to the point of being a differentiator for businesses in future. So kind of how do you so today, how do you not boil the ocean, but how do you achieve a balance between not boiling the ocean and making sure you've got the right technologies, processes in place to capture metadata that you might need to make some use of in the future? And I think there's probably, probably a couple of answers to how you address that. So the first thing is like, I mean I would say with everything that almost ever talk about in the, in the data space, don't do something if you can't see some value behind it, if there isn't some value you're trying to unlock. So look for challenges or opportunities within your business where you, where you know there's existing pain or where you know you could be more, more efficient with something. So, so if, like, if we go back to things like, like the recovery or prevention of issues, if you're constantly having, having issues because of like failure somewhere in your end to end kind of data life cycle, then maybe that's a good, a good use case for kind of some of those observability, failure detection and type metadata use cases. Similarly, if you're, if you've got teams that are constantly churning out reports and dashboards and pieces of analysis and you suspect that some of those aren't being used, then that's a good, a good use case to start, sort of. Well, okay, let's have a look at what the metadata tells us. And first of all, you don't need to like attach some big complex technical process to that. Just first of all, let's just have a look at what we've got and analyze it a little bit and say, right, you know, manually. Well, okay, is this telling us something? Could, could this help us cut out some of those, that unnecessary work? So dead simple, start, start small, start simple and start by, you know, as you would with like, I guess any piece of analysis, start by kind of pocing it and just understanding whether it's likely to deliver you any value before you try and put it into production. The other end of that spectrum though is I think it's becoming fairly evident that you will be able to gain some value from this in future. So when you're thinking about as an organization investing in your technology landscape, making decisions. So metadata should be a Part of evaluating technologies that you, that you bring in, so you bring in an application, you know, an ERP or something, you know, something into your organization that's, you know, going to provide you the functionality that you need and play, play an important role in your organization. And you should be evaluating that as well as, as well as on the kind of the functional and non functional aspects that you might traditionally evaluate it on. Can it expose its metadata? Can it expose information about how people are interacting with things? Can it expose the definitions of the data that are in there? So it, part of this is about making sure you're set up for success in future.
Juan Sequeda [00:19:13]:
That, that's an excellent point. And actually again, I never realized that what should go focus already you're going to buy a new tool or whatever, like evaluation criteria there should be is like how, like how can you expose, grab all that metadata? Because yeah, you may not know what you're going to be using it for today, but, but at the end that's something that can be helpful later on and you don't want to get tied kind of your hand sign like, look, there's this stuff I want to know, but you're not giving it to me, right?
Tim Gasper [00:19:40]:
So yeah, I mean this kind of flips things on its head a little bit because, you know, I'll, you know, we don't often put our vendor hat on, but I'll put the vendor hat on for a second. Right. Obviously Data World is a catalog and governance company. You know, a lot of times the dynamic is, oh, I have this weird system and can you please catalog it? Right? And we're like, you know, we already support 80, 80 different targets and like, do we really need that as the 80, as the 81st? And of course everybody, you know, wants their tools, right? Everybody wants their tools supported. But a way to kind of flip that on its head a little bit and to connect it to the point that you just made, Mike, is that as you're building out your stack, as you're making these decisions about technologies, maybe you should be asking the question, not always of your, of your metadata system, but actually of your data system to say, hey, when I choose, let's just pick a category here. My BI tool, right? When I'm choosing a BI tool, does it have a metadata API? What metadata does it collect? Does it have? What kinds of metadata does it have only technical metadata or does it expose usage metadata? Does it expose operational metadata? Does it expose semantic metadata? Right, yeah.
Juan Sequeda [00:20:48]:
And to add to that, I think it also flips, we talk about the whole shift left we should go do the work, right? And then so think about those tools, those vendors that you're doing. Like there's people who are putting in effectively metadata inside of these tools. And, and how do we know that? They're like, they're modeling things incorrectly or, or weirdly or whatever, right. So we're like, oh, we have some SaaS application, a CRM tool, whatever. Right. And it's super flexible. I can do whatever I want. Okay, so you're setting it up, but what the way you are setting things up, right? The fields, the new fields that you're adding and so forth, that's metadata too, that is going to get consumed further down and then later on people are complaining. Well that thing broke over here because of this fields over change over here. But it's not a field in a database. It's like you're consuming the field from the actual source of a SAS tool, which is still a proprietary tool or whatever, but it had a flexibility for you took actually decide what's, what to go put into that. But I think we're not, we're like, oh, that's just a field. I'm like, well we should probably put a little bit more importance in that. So what I'm loving about this conversation and this is like really small things, but you put them all together. Like this is what I, this is what it means to treat what I call treat knowledge as a first class citizen. Right? Knowledge, mandated context. All of this comes together.
Juan Sequeda [00:22:08]:
Like it's not like, oh, I'm just adding this one thing like no, it has, it flows through everything, just like data metadata flows through everything. And it just gives us that extra context to understand so much stuff about what this stuff means.
Mike Evans [00:22:20]:
It's totally right that there's a re. Like there's a really interesting point in there that, that you know, done well and done at scale and you, you can, you can maybe caveat this by saying, well okay, organizations only hold that they only hold as data, you know, a certain proportion of the thinking and things that goes on within the organization. But it's certainly a big proportion. So the value of that data and the metadata that surrounds that data is potentially very, very high. But if you, if you're able to analyze at scale what's going on in your data landscape, what's going on in your data ecosystem, you're able to an extent to assess how far your organization's data kind of represents the reality of your organization. So your example about data model and field Changes in a system, you might be able to discover that. Right. Okay. Someone set up a field called telephone number in this application over here. But actually what we're finding is what it, what it looks like in that field is some credit card numbers because they follow the pattern of a credit card number. So your system, the design that went into that system just no longer matches the requirement that the business has, which appears to be to store an extra credit card. I'm hoping no one's doing this.
Mike Evans [00:23:51]:
This is terrible practice. Right. But like you're spotting that design decisions that you made no longer actually align with the needs of the business and so you're able to take action and perhaps remedy some of those, those design decisions as well. There's a big kind of school of thought about being like design versus analysis led in your design of data solutions. So like my background has been designing master data management solutions. That's where I started in the data space. And I know from doing that the designs and the solutions are only as good as our understanding of the business that we're designing it for. And often that relies on the people that we're talking to and the samples of data that they provide. And if we have an incomplete picture, we might not end up with the greatest design or at least a design that matches their business requirement perfectly. So the power of metadata to help actually aid design is also incredibly powerful tool.
Juan Sequeda [00:24:56]:
Yeah, you had it. I didn't get to catch it right here. But I'll go Back to minute 24. You had a great sound bite there about like the design of the systems are just as best as you're talking to people, you figure out what the stuff is. Right. Otherwise it's just, it's your world and that's different around that. So, so how. What does good look like here? And in an ideal scenario or in your experience, what you've been seeing, things that you're like, wow, this, I'm proud. Like, this is really, this is great like this providing business value and everything. Like what does good look like there and, and how much of that is actually tooling versus again the people process and strategy. And just to kind of go back to one of, one of the use cases come to be very simple is like you say, okay, your team is trained out all reports and you suspect they're not being used. Like I could just go into the tableau or bi analytics tool and just go see the last amount of views and I can like, well, nobody's been looking at that stuff, right. So I don't have to get that sophisticated around these things.
Mike Evans [00:25:55]:
Right.
Juan Sequeda [00:25:55]:
So. So back to what does good look like and how much is it? Technology versus the social side.
Mike Evans [00:26:02]:
Yeah, it's interesting because obviously there is a significant technology component to this. Right. We are talking about technologies that are generating, consuming, analyzing metadata. So obviously that's a big part of it, I think. So when we talk about what good looks like. To your point. Yeah, I can, I can go in and scan any, you know, database worth its salt and find out, you know, how often tables have been accessed and you know, I can, I can get a level of metadata out of there that I could potentially use to drive some analysis. I think where I would say that you, there's kind of a level up from that is where a, an organization has kind of understands and is investing in the value of metadata. First of all, first of all there's a recognized importance of that metadata. Secondly, that they're looking at the breadth of their landscape and not like one individual database or report or system in isolation. Albeit that's still maybe not a bad place to start if you haven't got anything. They're looking at the breadth of their landscape. And that, and probably this brings us back round to the idea of data catalogs again is that, you know, that they're also, they're probably also in a place where they've invested in kind of more, you know, the more traditional data catalog use cases and they've got people interacting with the data catalog because that again it's, it's driving some interaction with, with the, with the metadata that you have, you're likely to, you know, for example, if you've got people who are able to log into a data catalog and search for the type of data that they're looking for and it bring up the different data assets and data products, you know, however we describe them that are relevant to them, that, that's, that's a, that's a better experience. They're more likely to pick the right things to use. You're going to get better date, better quality of metadata out of that scenario because you, you're not just dealing with people who, you know, go to one system because it's the only one that they, they know discard what's in there because it's not what they need. You know, you're dealing with a more joined up data estate in the first place. So to go. So that's a bit of a long winded answer, but to go back to your point, it's definitely part cultural and I think Having a culture in place where the sort of data community within an organization are interacting with the data catalog, they are engaged with their data and they're getting value from some of the metadata that's already around. You will gain, I think, better quality metadata and better insight from that metadata in that sort of culture.
Tim Gasper [00:29:10]:
Are there certain use cases that you think that organizations should try to tackle first when it comes to active metadata? And is there sort of a, an ordering here? Like do you do passive metadata first before you do active metadata? Or can you kind of, you know, approach it where you do active metadata but you start on something small and specific first? Curious about your. Yeah, I mean, on use cases and focus.
Juan Sequeda [00:29:33]:
Yeah, it goes back to what are the use cases that you see when. Of. Good.
Mike Evans [00:29:38]:
Okay. So, so let's, well, we'll, we'll start with the, the kind of order that you, that you do things. And this goes back to, you know, talking about that, that kind of embedding the processes and culture which says, you know, anything we do, we've got to be thinking about the metadata that we can, can gather from it. So let's start with can we do passive or active? Well, almost by definition you've got to do passive before you do active because you need the passive metadata to analyze. But you could capture the passive metadata on a much, you know, from a much smaller subset of your estate and perform some analysis over it and still get some value from that and still be able to deliver real value. And actually maybe that's, that's quite a good approach in tackling active metadata use cases because as we've said, it's a, it's a potentially confusing term. You know, you take this to the leadership of your organization, they're probably going to say, well, what the hell are you on about? What, why, why is that important to me? But if you can say, actually we utilize active metadata to prevent all of the, these failures, which would have cost us X, or we've used active metadata to reduce the spend on dashboard development or report development or whatever by this much, then we start to be able to kind of give some tangible examples and use it as a bit of a kind of shining light for doing similar things in other parts of the organization. But the things that I'd say is, so back to my point for I'd always, always look for things that are going to drive some value and think about how mature you are today as an organization and how ready you are to do some of this stuff. So let's take the kind of data issue prevention use case. If you, if you know today that you haven't, that you're not capturing any metadata from, you know, any of your data solutions, or maybe one or two in isolation, you're not, you're not doing anything with it. You don't have a data catalog in place. You don't have anyone whose job it is to kind of make sure metadata is captured and analyzed. You're probably at a relatively low level of maturity in this space. And so if you want to, if you want to pursue some kind of proactive maintenance type of use case, you're probably going to want to start in a small area where, you know, you've already got problems today, where you know, you see challenges, and then build it out from there. You can then put a roadmap where you gather the passive metadata, a limited subset of your passive metadata, you perform some analysis over the top of it that's relevant to that use case and to the scenarios you're trying to prevent and you try to demonstrate some, some value of actually preventing those scenarios from happening. If you try to do your whole estate, you'll be at it for years and it'll be a long time before you deliver any value in any use case.
Tim Gasper [00:33:06]:
Yeah, so I'm hearing a couple of areas of potential low hanging fruit here. Like one of them you mentioned was reducing spend on dashboard development or focusing it on more high value areas where it's going to have a bigger impact. Fact. Another that you're mentioning is around, you know, surfacing data issues, you know, and maybe accelerating the time to resolve and a way that you don't blow the ocean. There is maybe you focus it on those places where, you know, you have challenges and maybe where you have, you know, critical pathways of data for your organization. You know, are there any other low hanging fruit that you might recommend that.
Juan Sequeda [00:33:45]:
I want to add to this?
Tim Gasper [00:33:46]:
Yeah, yeah, Juan, go ahead.
Juan Sequeda [00:33:47]:
I'm surprised I haven't heard anything about data discovery and search, of finding data, because that's all passive.
Tim Gasper [00:33:55]:
Or is there a way to do.
Juan Sequeda [00:33:56]:
That relates to the metadata, you can argue. Well, I can't. I mean, the comment, the comments always hear like, why do you need a catalog? Well, because we can't find our data. Okay, good. We try to democratize data. We're right, we're, we're trying to be data driven. So we need to find our data. I'm like, but it's interesting then in this conversation that we've had up to now, like we haven't Been talking about search and discovery of data.
Mike Evans [00:34:20]:
Well, I guess where your kind of interesting active metadata use cases are, in that space are things like. So, well, your catalog is another source effectively for capturing metadata that can then be analyzed as well. So I mean, is it meta metadata? Are we talking here? Let's not go too crazy. But like, I come into catalog and I'm looking for a source of customer data. So I type in customer data, I'm presented with some sources, and I choose certain sources based on my needs. That journey that I've just been on is of interest to the organization. Right. That tells the organization something about, perhaps less interestingly, my habits. But if you extrapolate that to other people in the organization who are also using the catalog to access data, it says, well, what data are they trying to access? What are they searching for but can't find? Which. So once they've been presented with a search result, what are they actually choosing to go and work on and analyze? And again, it's probably relating more back to the sort of the use case that talks about, we talked about cost saving, but really it's directing your resources into the right place and directing your investments into the right place so you're not wasting money on things that you're organizing. Organization just doesn't need. But perhaps you thought it did. So that becomes another really useful source of information, of metadata to analyze, to say, right? Well, okay, what's the problem? Are people not accessing certain data sources because they're not good enough, or actually are they irrelevant? Should they be retired? Should we invest our efforts in improving them or should we save money by retiring them? Could do we. Where do we direct our spend? If everyone's looking for customer data all the time and no one's looking for supplier data or whatever, maybe we put more, more investment into improving our customer data management processes, our customer data analytics, you know, whatever it is. So that's where I think the catalog becomes. It's absolutely crucial in this, but it also becomes itself an important source of metadata. Yeah.
Juan Sequeda [00:36:42]:
And so, so I, I find this super interesting because the whole conversation about metadata and metadata analytics and it's gotten really into more of what I call like the, the data ops side. Right? It's like it's all about the operations or these things. And like now this whole search part is, in a way, the, as you just mentioned, the meta, Meta analytics, right. Like, effectively it's like, okay, now you have this stuff inside of a catalog and like now what you really want to go do is analyze the metadata of the catalog that has a metadata that stuff because see what people are searching for and so forth. Right. So, but, but that's, I mean it's so valid because that, I mean you still want to know where people are searching, where they're going and stuff like that. So anyways, that's an interesting, interesting observation I'm seeing here. And I wonder kind of for folks who are listening, where, where do they stand, right. Is, are folks who are listening, are you more in that kind of operations side or, or are you more on the like the usage of the data? And this goes back to like the different Personas, right? Are you more the technical users. Right. Of a metadata catalog system or are you more the consumers? Because the consumers are going to be looking more. I mean the way I perceive is that consumers are going to be more looking for the search and discovery use cases while the technical Personas are more about the data ops and all this operations type of things. So then it goes back to like what is the culture of an organization, right? It's like anyways, I find it, I, it's really interesting to go see kind of how metadata is like so broad and you can have like so many different angles. And I think depending on the maturity of organization, I would argue that they probably will start more from like the technical side. Like that's about right. And then because they want to get into the whole having the data that people can find and having data products and their marketplace and all that stuff. So it's really interesting. Again the dots I'm connecting is that our conversations have all been about more of the technical side. And it kind of makes sense because if you're, if you're starting your journey, you're probably going to start with your technical side. But also kind of the caution here is like don't just stay on the technical side. We need to be able to get up to the business side. So.
Mike Evans [00:38:48]:
Yeah, totally. I mean the easiest analogy, like the data product analogy really, really helps here is like if you, if you're talking about, you know, real world products on a marketplace or an Amazon or you know, whatever it is, and you, you would naturally, as a, as a, as a manufacturer or a distributor of those products, you would want to analyze their performance, their self performance and do things about what you find in the analysis to maybe evolve your product range or change the product sometimes or whatever it is, that's a, that's a very natural thing for a product manufacturer to want to do. So why should it be any different for Data products. As a producer of data products, it's the same and technology, you know, you, you, you, you want to be able to analyze the data about that data to, to either change the, the portfolio of data products that are out there or, or, or evolve the products themselves to be better or more tailored to the needs of the, of the consumer.
Tim Gasper [00:39:50]:
Yeah, yeah. You know, this is interesting. I'm just thinking about. So we've been talking about active metadata. We've been talking about different organizations that have some different strategies, different goals, different levels of maturity. You know, one of the other topics that, when we were preparing for this conversation today that we had talked about was around data fabrics. And you know, I'm curious, Mike, for you, what do you, what do you think of when you think data fabric? I think that's another one of those slightly ambiguous terms depending on which vendor or which company or organization is talking about it. What is a data fabric and is there a tie in here to active metadata when it comes to data fabrics?
Mike Evans [00:40:37]:
Yeah, well, the first thing that kind of leapt into my head when you said, what do you think, what do you think of when you say data fabric is active metadata? Like that? That is the first thing that I think that, that for me is the only thing that makes data. The only thing. Yeah, the only thing that makes data fabric as a concept different to, you know, every, every other iteration of kind of, you know, ecosystem design approach that we've seen over, over the, over the years. It's really the thing that stands out in the definition of data fabric from, you know, I don't know, logical data warehouse or, you know, an other like, number of approaches that.
Tim Gasper [00:41:21]:
I appreciate you being forward and that's-
Mike Evans [00:41:25]:
That's honest and no-bs.
Tim Gasper [00:41:27]:
There that are like, oh yeah, we, we're creating data fabric and you say, okay, well what are you doing? And they say, well, we're making APIs and we're making, you know, really well formed tables in our data warehouse. It's like, well, isn't that just good warehouse design and good API design? Is that a fabric?
Juan Sequeda [00:41:44]:
For me this is, you've really articulated it very directly here. But the data fabric is really about the active metadata about, which means like you're, you're actually capturing metadata and doing an analysis over that stuff because otherwise it's just pure blah, blah, blah. All you're doing is the same old data integration. You're probably changing some things, right? Some technology, but the same. You're just more fancy data integration. Right, But I think you take the data integration and you're improving it with best principles and so forth, but you're actually adding that layer of metadata. So that's what I really love to. But, but, but sorry, please continue. This is a really great connection that we need to make more.
Mike Evans [00:42:20]:
No, I think so. Maybe, maybe this is probably a good point to talk about sort of futures and why active metadata becomes so important to data fabric. And there's got to be a bit of a leap of faith conceptually into what could possibly be achieved in the future. But if you kind of think of a sort of situation where you have such good, a good handle over your metadata and are able to analyze it, to apply AI techniques on top of it, to almost make your end to end data ecosystem to an extent self evolving that it can, it can spot problems with itself and solve them. It can recognize where your various business systems don't quite meet the needs of the business and maybe even make changes to them automatically to bring them back into line. That's the type of thing that data fabric in theory could deliver with technological evolution. And you know, there's a good few steps forward before we're at that point, but that's the kind of, I think, utopia we need to be thinking towards. And so when I talk about kind of making sure organizations are preparing themselves for this and making sure that the systems that they have are able to expose metadata and they've got catalogs that can consume that metadata data, they've got tools that can analyze the metadata. That, that, that's why I'm saying that that thing. Because if you're, if you're, if at the point that this starts to kind of become a reality, if you're, if you're in a position where you're still, you know, haven't even got a handle on your metadata at all, you're, you're, you're a long, long way behind a lot of companies. And then we will see, you know, significant differences in organizational agility and the ability to adapt to kind of change. The, that as we've all seen over the past few years, that change can hit us and completely sideswipe us from our plans.
Tim Gasper [00:44:26]:
Yeah, we have to be resilient and not just overemphasize efficiency. And I like what you're saying here around some of the most exciting use cases that we want to unlock around data that honestly we've been talking about for decades now and we thought maybe would happen with the Hadoop craze and it didn't, unfortunately, right at things like self healing and you know, automated data operations and unlimited scalability and just AI that gets unlocked. Like all these different pieces, they can only happen if you take a data fabric approach. And they can only happen if that data fabric approach actually leverages active metadata. Because metadata is the key that unlocks all these different use cases.
Mike Evans [00:45:11]:
Yeah, yeah, absolutely. And I like, maybe just to go back on the data fabric thing, I certainly don't see data fabric as being single platform for everything. It's almost more about thinking in a joint way. And yes, okay, you need an architecture in place and a huge component of data fabric is the architecture. But it's about treating your entire data landscape as an ecosystem, recognizing that your analytics tool over here has all of these dependencies on perhaps the operational systems that created the data, perhaps master data management systems. There's data warehouses, data lakes, there's integration technology. All of those things, they're acting together as one. And one thing over here has an impact on multiple things over there. It's a bit like a butterfly effect. And if you're not, not kind of looking at. Okay, well, when I think about the performance of my data technologies and my data in general, if I'm not looking at that whole ecosystem, I'm missing a trick, I'm missing something out. And fabric really is kind of an approach to an all encompassing, an all encompassing ecosystem that leverages active metadata later.
Juan Sequeda [00:46:33]:
Mike, I want to say that this conversation here has been very, for me personally, very, very illuminating because you've taken these even like you helped me rephrase the words that I've, that I say goes through my mind. I'm like, oh, like, oh, this is just metadata analytics. Like, right, that's one thing here. And you described it too, like this data fabric. It's, it really is when you put the metadata analytics and activating that metadata, like that's what makes a difference. Otherwise it's the same thing. And I appreciate that kind of the honesty right there. And it's really that it's not a technology. It's that it's really holistically putting it all together. And kind of how I'm seeing it and tying it to my words is we treat data as a first class citizen. We now need to treat metadata knowledge as a first class citizen. And reminds me all the stuff that like Mark Beyer at Gartner always says, right, Metadata has been screaming at us. I mean, I'm a graph and it's been connecting. All you're trying to go do is connect all this stuff all over the place. And that's why it's not one technology. It's not just one thing that you go buy because you're, all you're trying to go do is just connect all this data stuff with all this metadata stuff and so forth. So, so yeah, this has been really illuminating for folks who are listening and they're like, they're. I think this conversation is incredibly complementary to all the data fabric and active metadata stuff that you'll see kind of from the pundits and the, and the analysts. So thank you very much, that's a pleasure. Well, hey, let's kick it off. Go to our lightning round right now. So we got questions lined up here and I'll kick it off. So is your catalog the home and the hub for your metadata?
Mike Evans [00:48:09]:
I, I think hub, yes, but metadata is, is everywhere and I, and I'm I'm not sure that your catalog should attempt to capture every piece of metadata and analyze every piece of metadata.
Tim Gasper [00:48:26]:
That is a well stated nuance there and I think it's pretty important. Okay, second question. Can all organizations benefit from an active metadata approach?
Mike Evans [00:48:44]:
I think I'll go a straight yes. Maybe in very small organizations there might not just be enough data to get any meaningful insight from yet. But, but, but generally I think there'll be, there'll be a case for everyone.
Tim Gasper [00:49:03]:
Yeah, I think that makes sense. You know, sometimes people ask like, well, what industries are going to be untouched by AI? You know, and you kind of be like, no, plumbers, no even plumbers. You know, they're going to use, you know, image recognition and stuff like that. It's like, oh, you know, active metadata kind of touches lot. Right.
Juan Sequeda [00:49:20]:
All right, number three, who is the driver of this active metadata? Is it the governance team? The data engineering team? CDO Office?
Mike Evans [00:49:31]:
Yeah, that, that, that's interesting. I think there is a, this, this comes back to, to kind of data culture. And so, so may. Maybe CDO Office is the, is the one that, that might, I'd lean towards. This all kind of goes back to, well, who, you know, who owns things like data governance in an organization, who is responsible for the quality of data. All questions that we've been asking, I guess for years and there are different approaches and different valid, correct answers to. I think the important thing is recognizing that and developing a culture where we value metadata to the point where if we're making a change, if we're implementing some technology or new processes or integrations or whatever, we're always a big component of that is thinking about, well, what metadata is it going to produce, is that useful to us? How are we going to capture it and how we're going to analyze it?
Tim Gasper [00:50:36]:
I think that's good. Yeah. And somebody should be accountable and responsible for driving it. But it depends on your organization.
Mike Evans [00:50:42]:
Yeah, it depends on the organization totally. And I think the fact that there is an accountability and that there is an interest and a desire to do something with it is way more important than who, if that makes sense.
Tim Gasper [00:50:57]:
Yeah, that makes total sense. All right, last lightning round question. This one's actually going to be an open ended question. What do you think is the most important use case that you think that active metadata can unlock? So not necessarily low hanging fruit, but the most important.
Mike Evans [00:51:15]:
Yeah, I mean for me, and it's again, it's that, it's going back to that futures thing again, but I, I think it's that, that self improving ecosystem piece. And I know it's something we've, we've already touched upon, but if you, if you imagine how, you know, the, the, the possibilities that you have there and the, the, the amount of, kind of directed effort that you can save on all of the things that we waste money in and technology and data teams today by being in that utopian state, that's the most important use case we can address. But I don't think we can ever see it in full today.
Tim Gasper [00:51:59]:
I like that. And maybe just to make one comment before we go to our takeaways here, that when you said that, my thought that I had in my head so I used to study physics a lot. Now it's more a side hobby than a more direct passion. But you know, this concept of entropy, right. Like today our data tools have entropy towards chaos. Like, you know, they, they, they, they, the machine runs and it becomes chaos. Right? But at some point, if we use metadata correctly, the entropy should actually go in the reverse direction. It should actually your data systems should, as they work together, become more and more effective and orderly. We're not at that today and how far it is, we don't know, but that's ideally what should be happening here.
Mike Evans [00:52:41]:
Yeah, I totally agree. That's a brilliant analogy for it.
Juan Sequeda [00:52:45]:
Takeaway time. Tim, take us away with takeaways.
Tim Gasper [00:52:49]:
Oh, always so many takeaways. This is always the hardest part of the episode. You know, we started off with, you know, what, what is metadata? What is active metadata? What does this all kind of mean? Right? And you said, Mike, that organizations create metadata all the time, right? Definitions of entities, attributes, data of how people are interacting with things, you know, technologies interacting with Things, where did it flow? Did people like it, did they view it, did they rate it, did they, you know, and you know, what we've all kind of agreed on as an industry, as a broader world, right, Is that like this is important information, you should put it somewhere. That place might be a catalog, it might be something a little more federated than that. And capturing it is one thing, but doing something with it is another. And it's really important that we do things with our metadata. Right. Bottom line, put very simply, and you know, what does that mean, do things with metadata? Well, you know, maybe put simply, it's metadata analytics, right. Metadata automation is another phrase that comes to mind. Although I know we've talked more about metadata analytics and you know, the sort of buzzword term I think in the space is active metadata. Right. And so we all kind of, you know, talked about, you know, active metadata. Is that, is that the best term or not? It's certainly the term folks are using, but it's really just metadata analytics. And if you use this metadata in an active way and actually analyze it, use it, you're going to get incredible value out of it, such as being able to spot patterns, being able to avoid data failures. When you use metadata in this way, you're going to be able to improve your data life cycle, identify what's working, what's not working. This is where we need to go. Obviously you shouldn't boil the ocean. And so you have to be careful about focusing your energy on where it's going to have the most impact for your culture, for your organization, for what matters, for your maturity. But you should find what that, what that use case is and take advantage of it. Metadata also should be part of the evaluation of the tools that you're bringing into the organization. So it's not just about, hey, we should collect the metadata for whatever we have. When you're choosing your ETL tools, when you're choosing your BI tools, when you're choosing your warehouses, think about is that metadata coming out of those systems that we can use, that is operational, that maybe has social information, make sure that you're gathering metadata. You know, my thought, don't just ask what your metadata can do for your data tools, ask what your data tools can do for your metadata. Juan's thought, treat knowledge like a first class citizen. So I love all that. Juan, what were your big takeaways?
Juan Sequeda [00:55:30]:
Well, one of the things that we, we got into, like what does good look like? So it's an organization that recognizes the value of metadata. They're looking at the breadth of their landscape and they have a data catalog, right. They have that culture of a data community interacting with a data catalog. They're always looking for things that will drive value. And then it's something you're always considering what your maturity is and how you're increasing that maturity potential low hanging fruits or reduce the spend on dashboard development or focus in on some more high value areas. You can do that by analyzing your metadata, right. It really and then also surface the data issues to accelerate time to resolve. And start by knowing the places that you know that you have some challenges. Today we got into that whole discussion like but what about data discovery and search? And I think that goes into like, like okay that's something that may happen inside of your catalog now or what are the user journeys. Right. But in a way it's like this meta metadata around there. I like how we did the talk about the orders of operations here, right. You want to embed processes and cultures that says hey everything we do needs to consider that metadata and what can we do with it. And almost by definition you have to do that passive style of like let's just kind of cattle gather all that metadata before you do any of that active stuff. But however you can always start with the metadata for part of your. From your part of your estate and then activate from those particular areas. Kind of like an iterative approach and then get into our discussion about data fabric. It's. It's when you integrate active metadata that metadata analytics into your data infrastructure and architecture. If you are not doing that metadata analytics you're not doing some data. You're just doing the same old thing. So and then what you're going to be able to get out of this activating the metadata is metadata analytics is that that kind of that self healing automations more of these scalabilities AI can actually unlock that incredible ways to be able to go improve your data infrastructure fabric is not just about a unified technology approach. It's more about treating your data landscape as an ecosystem and thinking holistically about it, especially the metadata. I think that, that, that, that was that final big tidbit right there. That nugget. Mike, how did we do anything we missed?
Mike Evans [00:57:33]:
Wow, that was pretty, that was a pretty comprehensive summary. No, I don't think so. I think the one thing that I would maybe say to businesses out there is just to restate that if you're not thinking about metadata and the potential of metadata almost forget the word active metadata, just the potential untapped source of knowledge that you have in your business metadata, you probably should start, start, start thinking about it and not, not because it's going to transform your business today, but because of the potential value it in Luxview in future.
Juan Sequeda [00:58:11]:
Well, I think this goes back to wrap up. We do our three questions right. What's your advice? Who should invite next and what resources do you follow? I think you just gave great advice right there. Who should we invite next?
Mike Evans [00:58:24]:
Well, I mean this, this is not intended as a, as a plug for Amplify, but I'd love to see my colleague Stuart Squires involved in a podcast in future. I think he. My background kind of going far back is technology. I'm I guess a technologist at heart, although I recognize I guess with most of, most of the things that we talk about in the data space that technology is only a small part of the answer. I think think Stuart is comes from a perspective of changing a business to kind of deliver on that data culture and is way more talented at that than I would ever be. So yeah, he would be a fantastic guest to have one, I think.
Juan Sequeda [00:59:15]:
And then finally, what resources do you follow? Podcasts. Yeah. Books.
Mike Evans [00:59:23]:
Obviously. Catalog & Cocktails. But you mentioned, and this is probably one that I would draw out you, you mentioned earlier on Mark Byer at Gartner. I really like the thinking that Mark presents and the sort of research that he's published. I think that that has certainly influenced me quite a lot in the way that I think about some of these topics. Other than that, you know, it's possibly an obvious one, but the, the original thinking that went into the, the kind of initial definition of data mesh. So Zhamak Degali, Martin Fowler, that, that, that, that body of research, I find that very, very interesting conceptually. And again, you know, we can debate whether or not that's the right operating model for people till the carriers come home always. But, but as a, as a piece of kind of thought leadership, I think it's quite an incredible sort of body of work.
Juan Sequeda [01:00:24]:
Well, Mike, thank you so much because this was again, I think a fascinating complimentary discussion of what you're hearing here. So I think my what I want people is like if you're getting confused around the stuff, this is the episode to get you un. Confused about active metadata fabric. Thank you. Thank you, Mike, as always. Thanks Data World, who lets us keep doing this years after years after years. And thank you Mike, again. Looking forward to having these, keep these conversations going.
Mike Evans [01:00:53]:
Absolutely. Thank you guys very much.
Juan Sequeda [01:00:55]:
Cheers.
Mike Evans [01:00:56]:
Nice to be with you.
Special guests
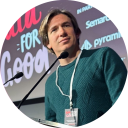