Tim Gasper [00:00:32]:
Welcome to Catalog & Cocktails. It's your honest, No-bS, non salesy conversation about enterprise data management with tasty beverages in hand. I'm Tim Gasper, long time data nerd, product guy, customer guy at data.world, joined by Juan Sequeda. Hey, Juan.
Juan Sequeda [00:00:45]:
Hey, Tim. I'm Juan Sequeda, principal scientist here at data.world. And as always, it's Wednesday, middle of the week, end of the day, and let's kind of take that break and let's go talk about data and quality data and AI and all that stuff and all the good stuff. Super excited to have today Kevin Hu, who's the CEO and Co-founder of Metaplane, a company in the data observability space. Kevin, how are you doing?
Tim Gasper [00:01:09]:
Hey, Kevin.
Kevin Hu [00:01:10]:
Good, good. You know, what do they say? Long time listener, first time caller. It is like being here in the virtual room with you all. I'm pumped. I got a nice fun cup too.
Juan Sequeda [00:01:23]:
Well, let's kick it off. What are we drinking and what are we toasting for? What's what? Tell us about that fun cup. For those who can't see, you have to describe it.
Kevin Hu [00:01:32]:
It's a Monty Python Spamalot cup that they gave out at the Broadway show. It's a hilarious show and I got it when my friend had his Broadway debut as an actor. So I try and use it only for special occasions because otherwise his autograph is going to rub off.
Tim Gasper [00:01:50]:
Wow.
Juan Sequeda [00:01:52]:
Tim, what are you. Tim, what are you drinking today?
Tim Gasper [00:01:56]:
Well, first of all, Kevin, thank you for being willing to wear a little bit of that off for us today. That is a true honor. I am drinking. Actually, I'm keeping it a little simple today. I got a little bit of balconies, brimstone. It's got a real oaky, kind of smoky flavor to it. It's a tasty bourbon that I got as a gift. So drinking a little bit of that. How about you, Juan?
Juan Sequeda [00:02:20]:
Well, I actually am super happy with my drink and I want people to pay really good attention to this. This is an Aperol Margarita, 1oz Aperol, 2oz Tequila Blanco. I have a lot a lime and also mandarin orange. Shake it. Boom. You'll, you'll love it. This is fantastic. I'm on this. Like I have, I had this whole bottle of apparel. I Think I just have like 3 ounces left or something. I need to go buy more. I've been really enjoying kind of figuring out my, my nude cocktails with apparel. So that's it. And, And Kevin, what do you want to go toast for?
Kevin Hu [00:02:56]:
All right, let's. Let's toast to our health. You know, we're going to be talking about AI and data and metadata. What does it matter if we're not, you know, healthy?
Juan Sequeda [00:03:06]:
We're talking about quality. Like we have to quality health too for all this. Sorry, it's a reminder. It's like we need to remind us that we have to go to the eat our vegetables and go to the gym and be healthy. Like that too. Right?
Tim Gasper [00:03:18]:
Self care.
Juan Sequeda [00:03:18]:
Self care. Right.
Juan Sequeda [00:03:20]:
We'll get into this.
Juan Sequeda [00:03:20]:
All right.
Juan Sequeda [00:03:21]:
Cheers to that.
Kevin Hu [00:03:21]:
Cheers. Cheers.
Juan Sequeda [00:03:23]:
Okay, so we got our warm up question. Today the topic is quality data. I mean high quality data. What else do you want? Like, do you strive to have high quality in your life? Like, what are the things that you really need? This must be high quality for me.
Kevin Hu [00:03:37]:
Oh my. You know, I'm. I can be a little bit prissy sometimes. You know, I would love to say something like a high quality conversation or high quality friends, but I gotta say high quality whiteboard markers. Oh, you cannot go back. It's. Yeah, it comes out and it's such a clean line. It's the B green Pilot V board master. Definitely use my affiliate link in the comments below.
Juan Sequeda [00:04:08]:
Hey, we're supposed to be non salesy here.
Tim Gasper [00:04:10]:
Non salesy about data vendors. But when it comes to Amazon affiliate...
Juan Sequeda [00:04:19]:
That's a good one. That's a good one. I did not expect that answer. But that's actually one of the things that's super freaking annoying is right, Is you have that marker and then it just ends and then you keep using it like just freaking throw it away.
Kevin Hu [00:04:30]:
It's like Robitussin, right? It's just like you keep trying to like, you know, make it work, put in more water, makes more Robitussin. It doesn't work like that. But I like your whiteboard. I said that last time. I have one right here too. Yeah, which is just a big post it on it.
Juan Sequeda [00:04:45]:
Just wait.
Tim Gasper [00:04:46]:
Nothing fancy.
Juan Sequeda [00:04:47]:
All right, Tim, how about you?
Tim Gasper [00:04:50]:
You know what, Kevin, you were just starting to make fun of it, but I'm actually going to say a high quality conversation. And the reason why I say that is like, you know, sometimes you just talk in with somebody and you know, especially people you work with on A regular basis and things like that. And there are those moments where you just, like, you really connect on a human level with them. And I was actually almost late coming into this episode recording today just because I was having one of those really human, very intense conversations with somebody. And, you know, it just makes you feel good to remember that we're all humans, we're all alive. And, you know, those are high quality moments in your day.
Juan Sequeda [00:05:26]:
Oh, I agree. And I. But I'm going to go off a completely different angle.
Tim Gasper [00:05:31]:
Yeah, go for it.
Juan Sequeda [00:05:32]:
Wine. Life's too short for.
Tim Gasper [00:05:37]:
The most unexpected thing Juan could say is high quality wine.
Juan Sequeda [00:05:41]:
Life's too short for shitty wine, period.
Tim Gasper [00:05:45]:
All right, I agree with that. I agree.
Juan Sequeda [00:05:47]:
I agree with all you. The market is the high quality conversations, friends. And like, imagine that, right? You're having a fantastic conversation with folks. You need to be at the whiteboard. You have a beautiful mark and you're drinking wine. Like, I mean, that's kind of.
Tim Gasper [00:05:59]:
That's the answer right there. Whiteboarding statue with great markers with some wine in your hand.
Juan Sequeda [00:06:04]:
All right, I should switch my cocktail for wine. I got you guys. Have a conversation. I got my whiteboard here, so let's go.
Kevin Hu [00:06:10]:
Whiteboards and wine. That's like the catalog and cocktail. Oh, that's like the Never Call Saul equivalent.
Tim Gasper [00:06:17]:
Better hurry up and trademark it or else we just. We just inspired our competitor.
Juan Sequeda [00:06:22]:
Yeah, you got already a good quote here. Whiteboards and wine. All right, let's kick it up. All right, we're five minutes in. Let's get it. Data. All right, Honest, no bs. Data quality has always been something we talk about. It's over and over again. And now GenAI, Generative AI is bringing up to the forefront. How is it, Is it anything different or is it just the same thing? And kind of like we're using this as a quote unquote excuse to kind of talk about it or what's. What's changing?
Kevin Hu [00:06:48]:
In a word, it is different. But just to back up a little bit the, you know, maybe former academics talking to each other. I love using the historical lens to make sense of the present moment. And when we see GenAI, right? And in this case, let's constrain ourselves to enterprise data applications of GenAI. So data quality, when it comes to training data for LLMs, we can put that over there. Very important. If you're listening to this podcast, you're probably not training an LLM. If you are, that'd be very interesting. But when it comes to applying LLMs and combining it with enterprise data, typically we're talking about very high frequency, very high stakes use cases. Right? But we've been talking about that for quite a while, right? We've been talking about business intelligence and decision support, especially in operational context, right. Going back like 40 to 50 years. We've been talking about automations also for decades. And for machine learning and data science, at least for the past decade, if you call it statistics, you know, you pull off the, you pull out the blanket and it's like statistics underneath, you know, that's a couple decades old too. And you know, in that case it's a continuous evolution from what data practitioners have been doing for generations now. But I will say that it is different in a couple of respects. One is that most GenAI applications no longer have a human in the loop, right? The moment you cross that boundary, it's very, very difficult to maintain high data quality. Most of the time there's a direct conduit to the customer. Right. So sometimes you're using, you know, a GenAI application internally, oftentimes it's directly a chatbot with the customer, then you stack. On top of that, the fact that now you're dealing with a non deterministic black box, you're dealing with introducing unstructured data which many folks don't know how to work with or govern, and the fact that you're dealing with natural language which is much harder to evaluate. Okay. It's like there's some ways in which it's the same, but many ways in which it's different. But on net, the complexity which is introduced with GenAI is significantly larger than it is for many other data applications. Curious where you all think about that.
Tim Gasper [00:09:26]:
I like that you kind of made sure to mention the non deterministic part because I think when you say, you know, that a human in the loop without having a human in the loop, that it actually makes it harder to have quality. It's actually a bit non intuitive, right. Or unintuitive because people usually think, oh well, is it the human part of the problem? And so like taking them out of the equation should make quality better, right? Kind of the promise of robotic process automation and all that. Right. But with GenAI we're talking about something different. We're talking about non deterministic, we're talking about unstructured, where the human in the loop would actually be better from a data quality standpoint. And we're trying to take that out. And that creates some pretty intense problems. Right.
Juan Sequeda [00:10:15]:
So first of All I appreciate the historical perspective and again, as one of the things that Kevin and I have connected is that we're both academics and kind of got into the startup and enterprise world around this. So I really appreciate this connection and getting to know you, Kevin. And I think that's one of, that's one of the important things. I think we both highlight that a lot. It's like, hey, we all think that we're inventing the newest thing, but just remember there's a bunch of history and what you're doing today is probably not that new. Right? Therefore it's important to understand it. And, and things are changing. So. But we want, don't, we don't want to reinvent the wheel, basically. Right. So, but what is changing here? Again, I want to highlight the, the non deterministic aspect, but I think when we talk about data quality, usually in our circle, data quality con means structured tables, your relational databases, equality over that. And so what I want to unpack more, and you brought this up, is that specifically for generative AI, we're dealing more with the unstructured. So what does data quality mean for unstructured data? What does. You also brought up the word governance. What does governance mean for unstructured data? And how is that changing? And the data professionals who do data quality and they're kind of in the structured world, is this the, is that, is this their task or is this the AI engineers who are doing things, engine AI, they have a new quality stuff. And then we're like, that's your quality, this is my quality. And like, I don't know, what's your perspective?
Kevin Hu [00:11:44]:
You know, the one reason why I'm so enthusiastic about GenAI is not only as a user, but also because it holds so much potential for data teams to flex their muscles. Right? Know we've been working on data like governing large volumes of data for years, decades, right? And to get a seat at the table, quote, unquote. But the problem is that Feynman said something along the lines of the same keys that open the doors to heaven, also open the doors to hell. Now in that case he was referring to the Manhattan Project, in our case referring to lm. So the, what I'm afraid of, to your point with the AI engineer, is that if data teams start holding up yield signs or stop signs, your CPO is still going to Invest in gen AI I.e. no longer going to talk to you about it, right? They're going to go spin up some shadow AI stack somewhere else the same way that they've spun up shadow data stacks. It's like, wait, you're getting, you know, you're getting your sales reporting from what, and put in like 2/4 to build this liquor dashboard for you. So the, I kind of want to call out that human and organizational side upfront before we dive into the technical side, which I think you've caught out, Juan, where, you know, personally, I take a very broad definition of data quality, where it's any sort of lapse between the state of data as it is and the state of data that's needed to meet a business use case. Now, this can be very inclusive of like performance and cost, but also more traditional definitions like accuracy and freshness. So to your question about unstructured data, the I think that the practices of current data teams and data governance initiatives, some of them can be applied, some of them also cannot be applied. Right. Some of them that can be applied is, okay, let's define some validation tests that make sure that our behaving, our system is behaving end to end as expected. Right. These are some questions that we have a ground truth about that our GenAI application should always get right. And you're always referring against those. We do that with data systems, I hope. Right. We should do that with GenAI systems. The but in other cases, there's new methodologies like you have large amounts of unstructured data, let's say it's too much to fit into a prompt that now you're dealing with chunking to, you know, chunking, embedding and inserting into a vector database. What exactly is quality control when it comes to that chunking mechanism? Right. What exactly does it mean to have anomaly detection on embeddings? Right. These are practices that, you know, we still had to develop. And I don't, I haven't seen any best practices emerge over time. I'm very curious to see, you know, five years from now when we look back, what those are going to look like.
Tim Gasper [00:15:02]:
Do you think that that is the big open surface area right now is kind of these, let's call them the gaps. Because, you know, obviously one thing that people are talking a lot about right now are kind of AI readiness. And actually Juan and I just did a webinar earlier today. We were talking about AI readiness and what's the difference between sort of data, you know, data governance and AI readiness? And there's a lot that's overlapped. Right. And you know, even if we just zoom in on data quality, there's a lot that's overlapped, but there's some new concepts in AI, like chunking, right? Like context windows, like the unpredictability. Right. Of like, sometimes you could ask the same question 10 times, and maybe nine out of 10 times it does it.
Tim Gasper [00:15:45]:
And then that 10th time it's like, what the heck? Like, did you. Why are you being creative right now? Like, that's not what I'm looking for. Right. So is that the mismatch, the big difference here, or do you think there's some broader differences as we talk about data quality in an AI context?
Kevin Hu [00:16:04]:
Now, when it comes to AI readiness, I feel like one bar that we at least need to clear is, is the data. Like, would you let a customer, like in the. An end user just query this data? Right? Because we are letting an LLM query it, right? And you know, potentially close to real time and to pass the results directly to the user. So if you're not comfortable having a customer, like directly query it because the data quality is not good, I don't think a team should feel comfortable having an LLM directly query it without a human in the loop.
Tim Gasper [00:16:42]:
That's an interesting litmus test.
Juan Sequeda [00:16:44]:
That isn't. Yeah, I was going to call it out. Just wrote that here without human loop. This is a really important test that you have there. I mean, that's a way of flipping it around. It's like, take the LLM out the window, right?
Tim Gasper [00:16:55]:
But Kevin, aren't LLMs magical and they can just understand our data like magic.
Kevin Hu [00:17:02]:
You know, not without. Not without some semantic understanding right now. Well, you know, Juan mentioned that podcast this morning, and I had to go back and listen to it if it's available. Or maybe the webinar.
Tim Gasper [00:17:14]:
Yeah, a webinar.
Kevin Hu [00:17:15]:
Yeah, like the webinar. Like, how did you all define data quality in that situation?
Tim Gasper [00:17:22]:
Well, that's a good question. Well, I would say that we didn't hone in on just data quality and unpack it all the way because it's kind of hard to fully unpack it. We honed in onto data governance, right? And how data. And how data quality is a part of how you need to be applying smart governance to your. Not just your data, but also your AI, you know, your gen AI applications. And so quality is a part of that. But, you know, even in that context, right, Quality is, you know, you know, not just the underlying data. Is it clean, is it normalized, is it available, is it queryable? But also, you know, what. What is the. What is the timeliness that matters? Right? What is the, you know, anyways, there's just a lot more to it. So I would say we didn't go into enough detail.
Juan Sequeda [00:18:10]:
I wanna pin this, this discussion about the quality that we had. The discussion because I wanna share our, what I call our profound take in a minute. But before I get there there's, there's something I want to. We have. One can argue that we have not been able to deal with quality in our current non genai world. So it's like what? I can't even, I can't even tie my shoes and you're telling me I just now go run. I'm like, so what's the deal?
Kevin Hu [00:18:41]:
So I have the bear case and the bull case for you and I hope that both are no BS cases is that, you know, the bear case is companies are going to do this no matter what, right. And the best that we can do is, you know, try to make sure that as much laces are tied as possible but ultimately your kid is going to walk and stumble, right? And then the question is how do you help them get back up and walk a little bit more confidently next time around, right? Without being like a know it. All right? Being like, oh I told you our data was bad. But being like, hey, you know, we had an, we had an LLM issue where hallucinated in a chatbot with $100,000 enterprise customer. I, you know, I can help you with that, get data reintroduced into the conversation. But the bull case is that the data team can actually be like the conduit for this data and the conversation where let's say you put a bunch of data into snowflake, right? That is the, you manage to have the like unstructured data that you care about. Let's say it's customer conversations, you have an ALM on top and puts sentiment into snowflake and now you use this to for like sales rep coaching at the end of it and you have and you're using Cortex or some sort of LLM within the warehouse on top is that now the company understands the purpose of the data governance best practices that you've already put into place and it lets you confidently keep building up more and more muscle in the organization as you go from like sales coaching to maybe go to market team coaching to maybe external applications. So that's more of a you know, eat the elephant, bite by bite approach. I've seen both, obviously, you know, I, I would prefer the latter but unfortunately I think the reality is that more folks are on the bear case where it's like, all right, just like buckle your seatbelt, like, let's see what we can do.
Tim Gasper [00:20:57]:
That's probably what more, more people are experiencing. There you go. Buy laceless shoes. Is that a new metaphor for the space?
Juan Sequeda [00:21:06]:
Usually we have, like, sometimes we get these metaphors that we continue going and going and going and we're like, we struggle. So we kind of like broke it.
Tim Gasper [00:21:13]:
So audience data slides or data loafers, let us know in the chat. So, you know, Kevin, bear case versus Bull case. I like that you posed these and I like that you've tried to kind of run with our metaphor a little bit that we've, that we've created here around the kid learning how to tie their shoes. You know, whether or not on a company, by company basis, it's the bear case or the bull case or, or as an industry, excuse me, as an industry, we're facing more of the bear case of the bull case. Are the strategies and are the tactics different? And being more specific, is data quality for GenAI kind of the same, regardless of whether it's the bear case or the bookcase?
Kevin Hu [00:21:54]:
So I'll give you one example from one of our customers. Like, no shill at all. But I think this is a useful example where like Ramp, they have. Ramp is a financial intelligence platform. They issue credit cards as part of it. I can use my ramp card for Metaplane, for example, and they have so many transactions as PDFs and they can put that all into Snowflake and then service it in their SaaS application as a spend benchmarking tool. So let's say that we're paying for liquor, right? How much am I paying per se, and how does that compare against everyone else? And how elastic is this price? So this is doubly an LLM application, right? Like, it's used in part for the ingestion of the unstructured data and also at the end for the querying of it. So the governance of the data that's within Snowflake, now that those best practices, I believe can be carried over from our existing structured data best practices. You know, combining tools, it could be data quality tools or metadata tools, generally having the right processes for input, validation, and ongoing checking and rotation of ownership and assigning ownership, you know, we can list all of them and also the right people, right? At a sufficiently large company, having folks who are responsible for governing the data and for developing the data platform and having the right metrics assigned to them. So when it comes to the structured warehouse, I've seen that Be very successful also in the LLM application. But it's not enough now, right? Because now you need quality applied on the unstructured to structured step. It could be very, very simple, right? So say use great expectations within this pipeline to say okay, you know, let's make sure that we don't have any like ingestion is what we'd expect. And two, to have more guardrails downstream, right? To make sure that answers are relatively, you know, fall into some balance. Some of the metrics might be new so using LLMs themselves to evaluate the output. LLMs like you know, what is the fluency of this output or is this coherent at all? It can also be referencing against like a test set like we said have 10 thou like a thousand curated answers that you expect to be correct. Or you can reference against the context as well. Like how much does an answer match up with the context? How much does the retrieved context match up with the question? Slash the prompt. So there's some new methodologies up and down, but I believe within the warehouse, if the warehouse is the conduit, it can be.
Juan Sequeda [00:24:52]:
This is a really interesting perspective you're bringing up because you're saying you, you're, you're. If, if the, if the center of the world ends up being structured, which one could argue that it's kind of most probably going, going to be the case because you want, you want to be able to go query it. I mean querying things at the end of the day are, I mean if you, if I pass, if you think about LLMs, if I pass the text as is. I mean I'm querying asking questions over that stuff now. But we know that from prop size and stuff that's gonna happen. I still need to go store all these things. And now what's happening is that that storage of stuff is happening. One kind of, I would call it a first wave of like rag. And all that stuff is it's being stored in vectors, right? And these. So you have things embeddings which is some sort of making some type of a structure in a way. You can do that, right? You're now see another wave of, of graph rag. Say no, I'm. I'm being able to go do extract things now in a more structured way. Happens to be in graph and so forth. Right? Because we, maybe we're seeing the evidence that graphs and knowledge graph really improve the accuracy for these things things. Then you can take it to the next level saying no, no, I'm actually extracting the tabular data. I'M going to store this in a relational database as is. Right. So then at that point like you're taking it to extremely like that's the known world, we know how to go deal with that stuff. But kind of the. So at somehow in the center you're going to get into some more structured stuff. So getting into that stuff, right. There's kind of like the same strategies approaches that we can use which I mean we, we won't apply them as is because it's, I mean I've already heard this tell it's text, textual etl, right. So you're gonna like it's probably not as, but the same principles can apply. But then the output of that you're also going to have the LLM consuming all these things and I want to have. So one thing I want to highlight here is on that latter part I've been thinking a lot about this kind of from, from our, from our labs and our research perspective and I mean again not, not, not a salesy thing but from what it's something we do at Data World that we're really trying to understand a lot of like the accuracy and asking Questions to with LLMs and structured data and testing is something really, really hard and it's new, it's interesting and I actually want to highlight. I was, I was at a last week I was at a conference in the the Data Engine Bytes conference in Sydney and I was hosting, I was monitoring this panel with Adi Pollock, Chip Yoon and Sunita Mall and one of the topics that came up was evaluations and I read my notes, I said the biggest challenge because we are shifting from a closed task such as like classifications or stuff to open ended tasks where we don't even know what the expected answer is. So if I ask you what is a summary of the book, how do I know it was a good summary? Well actually I won't know until I read the book. And even my definition of summary may be different from somebody else's. Right? So what does good mean? And the other example is many people can say if a first grade math problem is incorrect. Not many can say if a PhD math problem was incorrect. Right? So, so problem we're, and then we're now kind of pushing the barrier and saying oh these, these, you should be able to go deal with these problems, right? But it's like not even the humans sometimes know what the answer is off, right? So, so we should really need to go focus on test driven development on testing based on use cases and actually we need a lot of qualitative approaches. Anyways, my, my, my rant is quality is so different and testing is going to be such a big thing that we don't even know what we're entering in. And it's all because of this non deterministic systems that.
Kevin Hu [00:28:15]:
That is so true. And you know, it's, it's been fascinating to see folks both from the enterprise data world and from the machine learning world kind of approach this question of data quality for GenAI. Like the summarization that you, the summarization I guess question that you propose of like how do I even evaluate that? Right? That's a great, you know, a great example of. It's true that on the enterprise data side I don't think that we've, you know, who has dealt with that before, but I believe in nlp they probably have some metrics that we can bring to bear on that. Not perfect, right? It's not a yes, no or binary but if we can take some of those metrics but then ask one level deeper, it's like how do we improve this metric? Well, we have the answer to that, right? We know you gotta have someone on the case that you have to continuously measure what they're doing and you have to have policies assigned every step of the way. So maybe there's some, for lack of a better word, dialogue that can happen between the machine learning and the enterprise data worlds that are happening right now.
Juan Sequeda [00:29:27]:
Do you feel that, that dialogue, how much of that dialogue is happening or is it still kind of siloed communities?
Kevin Hu [00:29:35]:
No, I, it still seems pretty siloed amongst the different architectures because you know, it's, you know it's true that you see a lot of gen applications built on top of the snowflakes of the world, but you also see many that are not. And the. It's a little bit difficult to say. I've seen both. I will say that the most successful gen AI applications that I've seen have incorporated the data team. It's not like a software team just going nuts on a data lake with your PDFs, like 10 million PDFs in there.
Tim Gasper [00:30:17]:
No, I think that's a good, I think that's the right takeaway. Is that like, yes, the data team needs to be involved and it works better there, I think. Interestingly, I don't know if you guys would agree with this or not. I haven't seen a ton of GenAI applications yet that are really good at doing deterministic things like that. The use cases that are really going well are the ones where, you know, for example, summarization like, oh, it's so hard to test what is good summarization. Well, you know what, there's a flip side of that. The benefit is, is that there's a pretty wide range of answers which are. Is this good? Yeah, this is pretty good. It's pretty good, right? And, and, and the range is so wide that I've actually seen. You know, I remember a talk at the, the Data Council that was here in Austin. This was like six, six plus months ago where they were saying that they were using GenAI to test GenAI. So basically they would create like a summary, right? And then they would use maybe a different LLM just to kind of mix it up a little bit. Right? So now instead of OpenAI, now you're using Claude or something like that and you say, hey Claude, is this a good summary? And Claude says, yep, this is a good summary. And you're like, okay, cool, test pass. Right? But like, I don't know, it's like at that point, like, are you, you know, who, who's minding the, the hen house, right?
Kevin Hu [00:31:33]:
Yes, the. So that's yet another introduction of complexity that I don't think we have really grappled with yet as an industry. It is like, you know, we talked about other sources of complexity of the non determinism being a big one, but yeah, just like the layers upon layers upon layers of LLMs. The hope is that the ones that are doing relatively, you know, simple tasks, you like, evaluating outputs, are less at risk than the ones who are, you know, querying 10 years of enterprise data to give a result to a customer, but not, not so sure about that. It is a big risk.
Juan Sequeda [00:32:14]:
Yeah, I think we're at a stage right now that we're just figuring out what we can go do and we have to go test all these things and we're figuring out the limits and figuring out like I'll like, I don't think if this is a task that's well defined, A logic test is defined by deterministic algorithm. Like why would I go do this with a non deterministic approach? I mean the reason why I would do that is because I'm lazy. That is, that's a valid reason, that's a good person. It actually may not be cheaper. Right, because you probably have to pay for this stuff too, right? I mean, I would say, you know what, the best, the most cheapest way of answering question of your structured data is write a freaking query. Don't, don't tell the LLM to go memorize all these things. But people are gonna go try that stuff, we're gonna go find the limits and then yeah, we'll. So I think that that's going to happen naturally. But I think one of the, one of the things that I am curious about to go talk about here is here's this question that somebody brought up. Who's responsible for this now? And because you could argue that before GenAI, all that stuff it was, we still didn't even have a concrete answer. And now it's even more like you just said that there isn't enough dialogue between ML and data communities. Right. So yeah, so we're just, I guess we're, it's always going to be a bunch of silos, a bunch of shadow stuff. And I mean that's how a lot of modern data management has evolved. I mean, I don't know. What are your thoughts?
Kevin Hu [00:33:48]:
You know, I have one answer with two parts. One that I think data professionals will like, one that I think that they won't like. One is that it's built off of the joke. Why do doctors have bad handwriting?
Juan Sequeda [00:34:10]:
Why?
Kevin Hu [00:34:11]:
It's because they, they never have to read their own handwriting. Nurses do. Pharmacists do. Right. And as we know, one of the biggest cause of data quality issues in addition to code is input data. Right. First party data, third party data. Right. And so from that perspective, the business has to get their act in order. Right? Like ask your sales rep to take a minute, right? Just like ask your Salesforce admin to like take one hour to have input validation. How much time is that going to save? So much time down the line. And yet. So that's the first part. The second part is that I do think it is the data team's responsibility to be the ones who are like really, really pushing to close that feedback loop between the people in the business inputting data and the people in the business using data. Right. And to give them the path, like show them the way to lace up their shoes and then start running. Right. Because if it's not the data team, then who, Right. Do you think that sales rep is going to go out of their way to change the salesforce input validation? Probably not. Not because they can't, but because their incentives are not there and the data team's incentives are definitely there. What do you think of that?
Juan Sequeda [00:35:41]:
I mean, well, going back to the question that was posed here is like there is no consensus. So your answer is I agree there is no you gave two possibilities.
Kevin Hu [00:35:49]:
Right. So you're right, you're right. I would say that my take is that it's the data team responsibility, but that it's, it's not their responsibility to fix everything. It's their responsibility to set up the incentives and the dynamics for other people to fix things.
Tim Gasper [00:36:10]:
Okay, so it's not just maybe to put differently or put it in a different way. Right. Is that like sometimes we think of the data team being responsible for data quality or in some organizations the data governance team being in charge of data quality or you know, but really what, what you probably need to think of is that like, no, they are, you know, whether it's the data team or the governance team or maybe they're both part of the same organization. Right. Is they are trying, they are trying to create a framework for data quality to happen, to happen predictably to happen in the right ways to be fit for purpose. It's about establishing a muscle for data quality. But data quality itself is a shared responsibility across the entire organization and sometimes across into other organizations that you deal with.
Kevin Hu [00:36:59]:
That's a much better way to put it. Yes, that is, yeah, that, that is significantly better.
Juan Sequeda [00:37:06]:
Okay, sowithout being salesy and you're. You founded a data observability company. So based on what Tim is saying, are you seeing that in practice or what are you seeing how are the users of your tool actually? Are they playing frameworks or stuff or are they playing firefighters? Or something else?
Kevin Hu [00:37:33]:
It's the adoption of data observability tools is definitely still quite reactive. Right. Like data teams want to become proactive as we know and start building production grade data in reality. Often, you know, poop has to hit the fan first and then it's like, okay, no, let's bring on Metaplane or never again.
Tim Gasper [00:37:55]:
Right?
Kevin Hu [00:37:56]:
Never again. Like I'm not. Yeah, it's. But what I am seeing through sometimes is through things like a Chrome extension next to a looker dashboard. So when someone looks at a dashboard like, oh, you know, I see that this data is delayed more than expected. Other times it might be through someone subscribed to a Slack channel where they see the alerts and are keeping their eyes peeled for tables and dashboards that they use. I am seeing. Or at least our customers are telling us that there is more and more behavior change because on two levels. One is that now business users are kind of like disintermediating the data team in a healthy way. It's like, wait, I could check this data myself. I don't have to do it in an ad hoc way. But more importantly, they're trusting that the data team is on top of it. It's like, okay, yes, you have a tool and you have systems in place and that kind of gives a little bit of the breathing room to start establishing real. I wouldn't say like an SRE for data playbook. Like when, when poop hits the fan, what now? What exactly does a postmortem look like? Do we even have one? But this is, this is not every situation. These are the best situations.
Juan Sequeda [00:39:18]:
That's a fantastic point that you brought up. And I don't. And I don't see this that often.
Juan Sequeda [00:39:22]:
I don't know, like, is a post mortem, like, we're not doing another. I think about it like a, A win loss analysis. Right? I mean, think about. In sales, you do win loss analysis. Okay, we won this deal. Why did we win it? Right? We lost the steel, why did we lose it? Like, let's learn that. Like, hey, we are being successful at this. Like, let's make sure we understand why we're being successful to make sure that we put those into our frameworks and so forth. And this stuff is failing the time. Like, and we fixed it perfect and it stopped. Like, what, what happened? Like, don't just tell me what was a fix, but, like, what actually made it. Like, I don't think we are doing that analysis.
Kevin Hu [00:39:59]:
Yes. And when we do, it's always in a very negative or often in a very negative context. Right? It is like, oh, dashboards were down for the past 48 hours and happened to coincide with a board meeting. So we have egg on our face. Why? Right. Yeah. But, you know, that's something that I would love to be reframed in the data world is. And it's not because of data practitioners. It is like, if everything is going according to plan, that's not a win. That's your job. Right. If things aren't going according to plan, that's a loss. So there's no wins, only losses. And it's like, okay, come on now. Which I think is a. Where GenAI does hold a lot of promise is that you can get those quick wins. The last 10% is a headache, but the first 80% is hopefully much easier than other data use cases in the past.
Tim Gasper [00:40:56]:
I like the way that you're kind of positioning this. And the thing that I think in my head when you talk about this is that it helps to explain to Me a little bit. Why data teams? I think there was a period of time where it was kind of like, call it like the early 2010s so long ago, where, you know, like, data engineering was kind of its own team. And I was like, oh, we're going to have like the infrastructure team that's kind of like focused on data. Right. But I've seen more and more lately, like bundling analytics into the sort of the data team. And now some gen AI type initiatives are getting bundled into the data and analytics and AI team now. Right. It's kind of coming all together. And to bring it back to what you said, I think part of that is because data teams are a little sick of always being like the firefighters and always being the ones that have to react to, oh, we got egg on our face. And like, oh, it's because the pipeline's not quite good enough or because we had that data downtime for that one minute and it happened to be exactly when the CEO hit present on the tableau dashboard. Right. Like, dang, like, let's get the right tools in place. Like, they want to be at the front of creating value for the organization. They want to be able to create innovation for the organization. And unless those things get bundled together, it can be hard sometimes to turn data quality from a reactive conversation into a proactive one.
Juan Sequeda [00:42:16]:
So to follow up on this, I want to get your perspective, Kevin, is. I feel that we have always this kind of celebrating the reactive firefighting. Like, yeah, it. I mean, it does freaking suck. You have to get woken up in the middle of the night because something's happening. Right? Like, but, but I feel that sometimes the sentiment is like, oh, yeah, like, poor you. Like, you have to go do, like, when shouldn't we be flipping that script around and saying, congratulations, nobody's been waking up. Like, congratulations. Like, we, we really need to flip it to be more proactive and, and stuff like that.
Tim Gasper [00:42:51]:
Like, it's like the manufacturing, when you walk into the power plant and it says like, 35 days, no injuries.
Juan Sequeda [00:42:58]:
Yeah, exactly, Right. Like, I feel that we. Is it just like a. Is it. Is it a cultural shift, an easy cultural shift that we can go do, or is it like, we're just so bogged down. I can always talk about the urgent versus important. We're so. He's. We have to go with so much of the urgent stuff that we never get done with the important stuff. And therefore, the important stuff is what would make us be proactive. And we're always being reactive. Like, is it the saddle. Or is it. Or, or do you think there's like a quick win on, like, let's just change our attitudes a little bit and like, we can be more positive but instead of being negative around this stuff.
Kevin Hu [00:43:33]:
You know, it's, you know, when you describe that, you know, 37 days, I imagine like one for LLMs of like, you know, 37 seconds since hallucination. It is like, oh, let's go. Good gpu. Good gpu. Well, you know, I, I think it really depends on. Well, one thing is for sure, humans are difficult to change because relationships are hard to break and change and habits are hard to change. But that it is an important, you know, it's very important to do in order to flip the script to one of celebration rather than loss aversion. And I, I believe part of it is our belief in the malleability of culture. So it's like, I think a lot of times in conversations about data culture we talk a lot about like the etiology of it, like, why is it this way? Right? Oh, it's because our execs are coming from like engineering backgrounds or sales backgrounds. They're not really data people. And our data stack was built by a software engineer who left five years ago. And it's not enough about like almost like the teleology of our culture, like, what do we do today and what is the purpose of it? Right. And that's a decision that we can make in this given moment, right? Okay, yes. You can't really change everything, but like, let's start putting that postmortem in place, right? Like, let's just try it. We're not. Everyone has to buy into it. But just to say, okay, I went online, I found a postmortem template, I'm going to try it, right? And then kind of starting to build this virtual, this virtuous cycle of like, oh, wait, I, you know, I see what Jim did over there and actually I thought that was the most useful force, more than we've had in the past month. I'm going to take that. Right. So I think like this kind of agent of this, you know, is very, very difficult. I'm not going to throw the first stone, but I believe that data folks are in a good position to do that, especially with gen. Yeah, I, I.
Juan Sequeda [00:45:44]:
Really like this stuff. I mean, I honestly, this was like completely unprepared or like getting this conversation. I mean, get into this post forum. This is like, for me the most important highlight of this conversation, this takeaway is we need to go we, we need to have these win loss analysis of, of celebrate the wins and and, and, and not just cry because things are going bad but also because let's. This is how we're gonna go learn, right? And let's go do these post mortems and yeah, I'm gonna look up now post mortem templates and I mean I'm just gonna ask GPT right right now create me a part.
Juan Sequeda [00:46:20]:
I'm gonna stop.
Juan Sequeda [00:46:21]:
I'm sure I'll do something fantastic there.
Juan Sequeda [00:46:28]:
Look man, times flies. We gotta go to our next stuff. Tim, any final comments, thoughts, questions before we go into our lighting round?
Tim Gasper [00:46:36]:
Oh, that's a good question. I mean there's a few different directions I feel like we could go. We could literally do another hour of this back and forth. Maybe I'll do a pre lightning around question. So if you can keep this pretty short because I know we're starting to get crunched on time here. If you were going to give some recommendations to folks who are like, okay, data quality for AI, right, For GenAI specifically, what would be like a couple of your hot tips for folks that are like, hey, we're thinking about data quality. We're getting into GenAI. What are some tips that we should be really paying attention to?
Kevin Hu [00:47:07]:
Oh, I resist the technical tips because as much as we can talk about them, they will, you know, we'll see if they test. They stand the test of time. I think something that has been really useful has been the organizational research on this that truthfully speaking, like there's this one research study from Russell Reynolds. They are like an executive recruiting firm. You know, they placed the Starbucks CEO, for example, and they asked a thousand CEOs. It's been a while since I read it, but a thousand CEOs about their approach to implementing GenAI. And I won't forget that before implementation, I think data quality and data governance were not very important priorities or they weren't listed as barriers. And then as you kind of progressed from exploration to design to implementation, it became more and more important until after the fact, when leaders implemented GenAI. Now data quality was the most important initiative. So can we learn from their aggregate experience and say, okay, well there's hundreds of leaders out there who in hindsight wish that they invested more in data quality. Are we actually that different from them? So that would be maybe my first tip is that, you know, look at that research, look at some of the, you know, I hesitate to say it for like the Harvard Business Review surveys on implementing GenAI. I know, I know some of these numbers are just pure bs, but honestly they highlight important points that I think they do hold water in these conversations about whether or not to pursue GenAI and how to do it.
Tim Gasper [00:48:59]:
Interesting. Yes. That sounds like a great piece of sort of research to dig into that like, you know, learn from the past. Like to go back to your comments about history here. Right. And people who have gone through this, companies that have gone through this have seen that data quality is a really important and key missing ingredient. If you, you really want to take advantage of more advanced capabilities, more impactful capabilities and GenAI very much falls into that category.
Juan Sequeda [00:49:27]:
When we post this on LinkedIn stuff, please share, share a link to this, to the survey. I think this is really, this is a great point.
Kevin Hu [00:49:35]:
Yeah. You know, one other quick one, I know we're running short on time is, you know, the hypocrisy between, I believe something between like 75% of execs are feel an urgency to implement GenAI or have plans to implement it. And I believe like the Same number like 75% of execs don't trust their data. Like what, how is this so like there enough time has passed that we can start to learn from this first cohort of GenAI, you know, the early adopters. And I think we should use that to our advantage in the conversation because otherwise it's very easy to get overpowered with sheer zeal and competitive fear like oh, we gotta do it and fomo. Right. This is a case where I think you gotta use data to your advantage.
Juan Sequeda [00:50:28]:
All right, this is great segue into our lightning round. I'm gonna kick it off for the first question. So as of this moment right now, you think data quality for unstructured. They unstructured is well defined?
Kevin Hu [00:50:43]:
No. Are these yes/no questions?
Juan Sequeda [00:50:47]:
I mean you can give a little bit more context.
Kevin Hu [00:50:51]:
I don't believe it's well defined because there are, one, there's often a lack of a schema. What does a schema even mean in these contexts? And I would say most of the data quality when it comes to a structured data is kind of derivative from this idea of having a defined schema. And two, because like Juan was saying, even something like what is good? What is a good summarization is hard to define. So if you don't have like the high level structure and you don't have the specific metrics, what the heck is in between?
Tim Gasper [00:51:28]:
Yeah, you don't have a lot to work with. Right.
Juan Sequeda [00:51:31]:
That's an Excellent point. You lack the schema, the structure, and you lack the metrics. Like, we don't even know these things yet. Excellent point.
Tim Gasper [00:51:40]:
One random comment, and then I'll keep things moving, is that this conversation is making me realize how much value comes just from the process of loading data into a schema. Sometimes we overlook how like, oh, well, I got to get the data in the database. We just kind of like, we trivialize that. But actually, so much quality and semantics is both implied and explicitly enforced simply by moving data into a database.
Juan Sequeda [00:52:11]:
And, you know, just to kind of. Sorry I got you excited. I was thinking about this the other day is like, if you think about the schema and the metrics, you're like, okay, this is what well defined means. It means that this column must have these things and this thing. Perfect. I define the constraints. I know if it satisfies the constraints, it's all good. Got the schema. Go put the data in. Oh, shoot. It doesn't satisfy the constraints. There means an issue with data. Okay, gotta go clean that. Okay, so let me go figure out what's going on with the data. In the meantime, you're being screamed at. So I need this report right now. Right now. Right now. What happens? So you. Okay, you need it right now. I'm just gonna drop those constraints. I'm just gonna put the data in and like, okay, the data is in. Now you can go query, Create your dashboard. But then you just didn't. You just dropped the constraints, you dropped the semantics, you dropped the meeting, and then you talk, and then the quality just keeps pushing. Right. So, yeah, anyways, one last digression.
Tim Gasper [00:53:03]:
Bring on Ramona's latest comment here. I liked her. Her analogy here.
Juan Sequeda [00:53:08]:
Oh, I love this here. Unloading your groceries and putting them on the covers.
Tim Gasper [00:53:13]:
Yeah. It's not just about buying the groceries. It's the act of putting them into your fridge in your cupboard. All right. I love it.
Juan Sequeda [00:53:20]:
There's some people who have, like, very messy fridges and cupboards too. Right. They can't find stuff. Oh, there's. Here's a great analogy. Here's a great metaphor.
Tim Gasper [00:53:27]:
Are you talking about my house, Juan? All right, seriously. All right, Number two. Number two. All right. We're gonna. We're gonna. We're gonna be here all night. All right? We talked about data quality for GenAI. What about. Is GenAI gonna help us do data quality?
Kevin Hu [00:53:43]:
Oh, wow. I. I'm. Yes, I'm very optimistic about this. That I think, at least when it comes to semantics. Okay, I'm going To invoke it. I know Juan, like okay, we could talk about this for another hour, but in a, in a past life I did a very small bit of research into like semantic type detection almost like as a precursor to, you know, just inferring data quality in let's say a given column of a table in a database. Right. It's very helpful to know what you're referring to. Are these numbers, weights, are they latitudes, whatever. And LMS are surprisingly good at that question. Right. Of inferring categories and ontologies about the world. I would say that one of the strengths of an LLM is that it can infer concepts and relationships about the real world in a more abstract, non predefined way. And is that not kind of what a data model is? Right, like objects and relationships between data like within data database. So I, I'm pretty optimistic about it. I don't have anything much more specific than that, but I know Juan has something to say.
Juan Sequeda [00:54:58]:
No, no, I agree. Which actually happily is my next question. So we've talked about semantics is key for gen AI, right? Context providing everything. I mean we've been put all this research out. Is semantics going to be a bigger part of data quality going forward?
Kevin Hu [00:55:13]:
I feel like I can't, I can't say no otherwise I'm going to get kicked out of this room. I am so optimistic about that. I think that might be the missing link between what we have today, which is slapping LLMs on top of structured databases. Hit or miss, even when you have like infoschema and your query history loaded in. Right. To having something that is connecting what is readily observable from the database and the concepts that are actually somewhere in the LLMs. It's a little bit hand wavy, but I can see that working.
Juan Sequeda [00:56:01]:
We're good, we're good, we're good.
Tim Gasper [00:56:04]:
By the way, it's totally okay to say you hate semantics if that's what you really feel. So please don't hold back. All right, fourth question I've been seeing lately in the sort of broader data space, AI observability companies. And my question for you is, are data quality and data observability tools going to cover the AI use case or is there really a meaningful difference here?
Juan Sequeda [00:56:34]:
Oh, Tommy called the astronaut. Yeah, the non salesy, non sales again scientific academic head on the.
Kevin Hu [00:56:47]:
So my honest, no BS take on observability companies is that we're all basically building the same thing. We're all building metric stores and anomaly detection on top with some, with some thing to model relationships, to help you debug you. Call it traces, call it lineage, whatever. I would say that the real difference is that we're selling to different people and that difference is actually extremely important. Right, like the, you know, can you use Metaplane to track the, like the F1 score of the data that's included in LLM output? Like you can, but you're not going to because we do not market to you and none of our ergonomics, none of our messaging is designed for you, you know, Ms. AI Engineer. So there's no way in. How are you going to.
Juan Sequeda [00:57:34]:
That is a truly honest, No-BS answer.
Kevin Hu [00:57:39]:
Real.
Tim Gasper [00:57:40]:
That's a great answer.
Juan Sequeda [00:57:41]:
Great answer. Because it's true. It's like, yeah, we're all about the same shit, just market different people and the pie is so big.
Juan Sequeda [00:57:49]:
All right. I love that. What a great way to wrap this up, Tim. Take us away with takeaways.
Tim Gasper [00:57:55]:
All right, well we started with, you know, honest, no bs. What is dequeue for gen AI? Are they different? You know, is data quality different in the context of data and gen AI? And you said that. Yes, there are some differences. Right? And data for AI in the enterprise is kind of one aspect that we've been, you know, we've been talking about for decades, like how to do decision support, how to do bi, you know, under the hood. It's really all just statistics, machine learning that, you know, there, there is a difference here, but it is all part of sort of a shared spectrum. Right. And you kind of said that, you know, the main, main kind of thing that's different is that gen AI doesn't really have a human in the loop. And so that kind of non deterministic aspect combined with trying to actually use it for some of these different use cases has a pretty big implication in the shared aspects of data quality. But some of the different aspects of data quality and certainly we didn't go into it too much in our chat today, but you started to say that unstructured data in particular creates some different aspects and challenges around quality and governance that I'd love to unpack at some time. We'll have to follow up and chat more about unstructured data, which I think is sort of the, the wild unknown for data management. So, you know, why are you enthusiastic about gen AI and data quality in this context? You had mentioned that there's so much potential for data teams to really be able to flex their muscles here. And I love the quote that you had mentioned that comes actually from referring to the Manhattan Project, which is the same keys that open the doors to heaven, open the doors to hell. So hopefully that gets our listeners excited and not a little frightened about maybe that's good timing with Halloween coming around the corner here. So you also talked about if you don't get involved in the GenAI Project, it's going to happen. Organizations are excited, leaders are excited. CEOs everywhere are telling their boards and their shareholders that AI is the future. It's showing up 60 times in the stockholder report. And so, you know, it's going to happen. You're going to get involved. And actually we didn't go into it too much in our, in the conversation today, but one of our profound truths in our webinar earlier was actually around the fact that you can become overly obsessed about data quality and some of the foundation for AI when really you kind of have to take it in stride. Right. And I won't get ahead of. I think Juan will go a little bit more into the bear case versus the bull case. But you know, we got to be agile around this. We got to move quickly. Things are evolving fast. And then you said data quality is any lapse between the state of data where it is versus where it needs to be for the use case. And I thought that's pretty interesting. I mean, obviously that's very broad and could even capture things like ETL and some other aspects. But you know, that connects to maybe a broader theme to our conversation today, which is that data quality is kind of embedded in everything. It's not like this one thing that sits off to the side. It's even a part of the schemas of the databases that you're constructing, which is something we talked about in our lightning last. Before I hand it over to Juan, there are some unique challenges that you mentioned when it comes to data quality as it applies to the world of gen AI. For example, chunking. What is data quality on chunking? I don't know. Right. What is anomaly detection on embeddings? I don't know. These are all questions that we're going to have to figure out as we go forward. So there's shared things around data quality that we all can understand and there's some new frontiers that we're going to have to solve as we go go forward. All right, so much more. But Juan, what about you?
Juan Sequeda [01:01:41]:
I like this, the whole litmus test, right. Would you let it, your customer query and access this data right now? If you wouldn't, then why would you let an alum do that right. Without a human loop. So I think that's a great report test. I like to hold the bear case in your bull case.
Juan Sequeda [01:01:53]:
Bear case, companies are going to do this no matter what. So we have to ensure that. We're going to go enable them and help them. Right. We want to help the kids learn how to tie their shoes and yes, they won't fall, that's okay. But we're there to support them as they go. The bold case is that the data team can be the conduit for that entire GenAI conversation. Right? Just eat that elephant bite by bite. I really, we had this full great conversation about like if you think you're, if you're your structured databases or your warehouses in the center, right. Things are going into it. LLMs are going into it. LLMs are consuming it. But you have, you have ways of applying kind of the traditional data quality approaches in here. Go use that. We'll figure out what needs to change, change around that. One thing that needs to change that we don't know is really testing, testing. GenAI is new and it's hard, right? I brought up the conversation like how do you even know what is a good summarization? I mean like we don't even know what the metrics are. What good is. That's what makes it interesting. Today we need to have more dialogue between the machine learning and the data community. It's still very much siloed and what you, what you observe is that successful. Jenny applications are the ones that involve data teams. Then we're like who is in charge of data quality for GenAI? Like you have two perspectives, right? Like why don't, why do doctors have bad handwriting? Because they have to read their own handwriting, right? So kind of you have all these issues actually comes in from the input of the data, right? So one argument is that the business has to get their order in the house. Like hey, you have some mandate or responsibility or incentives for the Salesforce admin to show make sure that they have input validation because that's going to save so much time down the line. Or you can say that data teams have that responsibility but they, but it's also about setting up these incentives and dynamics so other people can go fix this. I think today we acknowledge that quality and observability is a much more reactive scenario. And I think there's, we've had this whole conversation about there's opportunities of turning this kind of into more of the proactive. A T shirt quote of the day Here is 37 seconds without hallucinations. Yay. Talk about so much about culture. And for me, the most important key takeaway here is we should really put these post Morton kind of analysis in practice.
Kevin Hu [01:03:57]:
Right.
Juan Sequeda [01:03:57]:
We need to make this into a habit. And there's a survey you mentioned, right? Data about a survey of over kind of the top CEOs or executives. Data quality is becoming the most important initiatives. Like in hindsight they realized that they needed to invest in more, invest more into that. And especially as you move on the maturity curve, it just becomes, becomes more and more important. All right, Kevin, how did we do anything we missed?
Kevin Hu [01:04:22]:
I think in terms of summarization score, you guys are a 10 out of 10.
Juan Sequeda [01:04:27]:
All right, LLM GenAI GPT. Let's compare.
Tim Gasper [01:04:31]:
Kevin was the test and he said it was a good summary.
Juan Sequeda [01:04:36]:
All right, what's your advice? Who should invite next and what resources do you follow?
Kevin Hu [01:04:44]:
You know, one piece of advice I think back on all the time is, you know, remember when you wanted everything that you have now it's, it's a little bit trite, but you know, it's so important to remember that there's so many, you know, life can always be better, but we're always on this, you know, hedonic treadmill wanting more and more and more. But 20 years ago, you know, the little, the little kid versions of us, you know, keep going back, like, what would they think of you today? I think for, I like to hope that for a lot of us they would be proud to see you. And I just always want to remember that fact.
Tim Gasper [01:05:20]:
It's a nice little confidence booster. Yeah, it's like, unless, unless your younger self would look at you and be like, what the heck?
Kevin Hu [01:05:29]:
Maybe a little bit of both. Like, oh, this is cringey. Yeah, but who should we invite next? I think Ian Machamer, the head of data at Ramp. I was trying to think of like, you know, insight density. Like, who do I learn something from every time I talk with them? And it's Ian. Very truthfully, Ian is the man. Super sharp both on the data side and on the business side and I think would have a very interesting AI perspective too.
Juan Sequeda [01:05:58]:
Nice. And then what resources do you follow.
Kevin Hu [01:06:05]:
When it comes to ML? I think Vicky Boykis and Eugene Yen are excellent. Both thinkers and writers. Same thing goes for more on the LLM side. SWIX swx. He runs the Latent Space podcast and is a really great guy. Recently I've been reading this blog called Common Cog by Cedric Chin. It's all about like business expertise, like, can we get better at doing business? And his answer is yes, he has a much more elegant way to put it. But I think it's very useful for data folks too, to think about, you know, how does what I do impact the business and how do people in the business think about the business? Highly recommend.
Tim Gasper [01:06:56]:
Interesting.
Juan Sequeda [01:06:57]:
Great.
Tim Gasper [01:06:57]:
I gotta check that out.
Juan Sequeda [01:06:58]:
Yeah, I've actually been. I've seen Cedric around, post like. That's great. Thanks. Woof.
Juan Sequeda [01:07:05]:
I tell you an hour. We're past the hour. Kevin, thank you so much. Quick reminder, next week we have Mike Evans, who's the Chief Innovation Officer at Amplify, and we're gonna be talking about active metadata, which, you know what, it's just analyze meta analytics, so it'll be a fun conversation. Also, we're both going to be at DBT Coalesce next week, right. So if anybody's around there, come find Kevin, come find me. I'll be there Tuesday at dbt, cos I'll also be at the Snowflake Data World Tour in London on Thursday. So if you're on there, come, come find me. And with that, Kevin, thank you. Thank you so much. Thanks. Data World lets us do this again every single Wednesday. And cheers.
Kevin Hu [01:07:47]:
Cheers. Thanks for the opportunity. Great to chat with you both.
Special guests
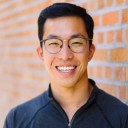