I love blogging about the exciting innovations that data.world is building on top of Snowflake. As a data catalog platform powered by Snowflake, data.world not only increases the efficiency and effectiveness of its internal data and analytics programs, but also quickly brings cutting-edge innovations to the market by leveraging Snowflake’s native governance features. Check out this YouTube video in the Powered by Snowflake series where our co-founder, Jon Loyens shares more about why we chose Snowflake as our analytics engine. In this article, I will cover what data.world provides to joint customers as a part of the Snowflake Horizon partner ecosystem.
Vision for Snowflake Horizon:
Snowflake has always been committed to providing a strong governance framework for technology partners to integrate seamlessly into its cloud platform. The latest launch of Snowflake Horizon introduces a built-in governance solution, featuring a unified set of compliance, security, privacy, interoperability, and access capabilities. Let me delve into the details of how data.world, the leading data catalog and governance platform, is leveraging these native features to provide our customers with advanced Data Governance capabilities.
Compliance: Extending Snowflake Data Metric Functions to everyday workflows
Last week, we announced our native integration of Snowflake Data Metric Functions (DMFs), which is currently in Private Preview. Customers can create their own metrics by writing custom SQL to monitor the quality of their data. For organizations seeking maximum flexibility in building their own Data Quality monitoring solution, this announcement is particularly exciting.
While measuring the quality of your data is crucial, it is equally important to share and distribute data quality information to relevant stakeholders effectively. The integration to data.world accomplishes this objective by automatically delivering data quality status and metrics to the people who need it, when and where they need it. By doing so, we streamline communications between data teams to drive better data quality. In the diagram below, you can see how data.world’s Hoot, an embeddable trust signal, is used to 1) detect data health issues from these Snowflake metric functions upstream, and 2) broadcast the results in downstream analytics applications.
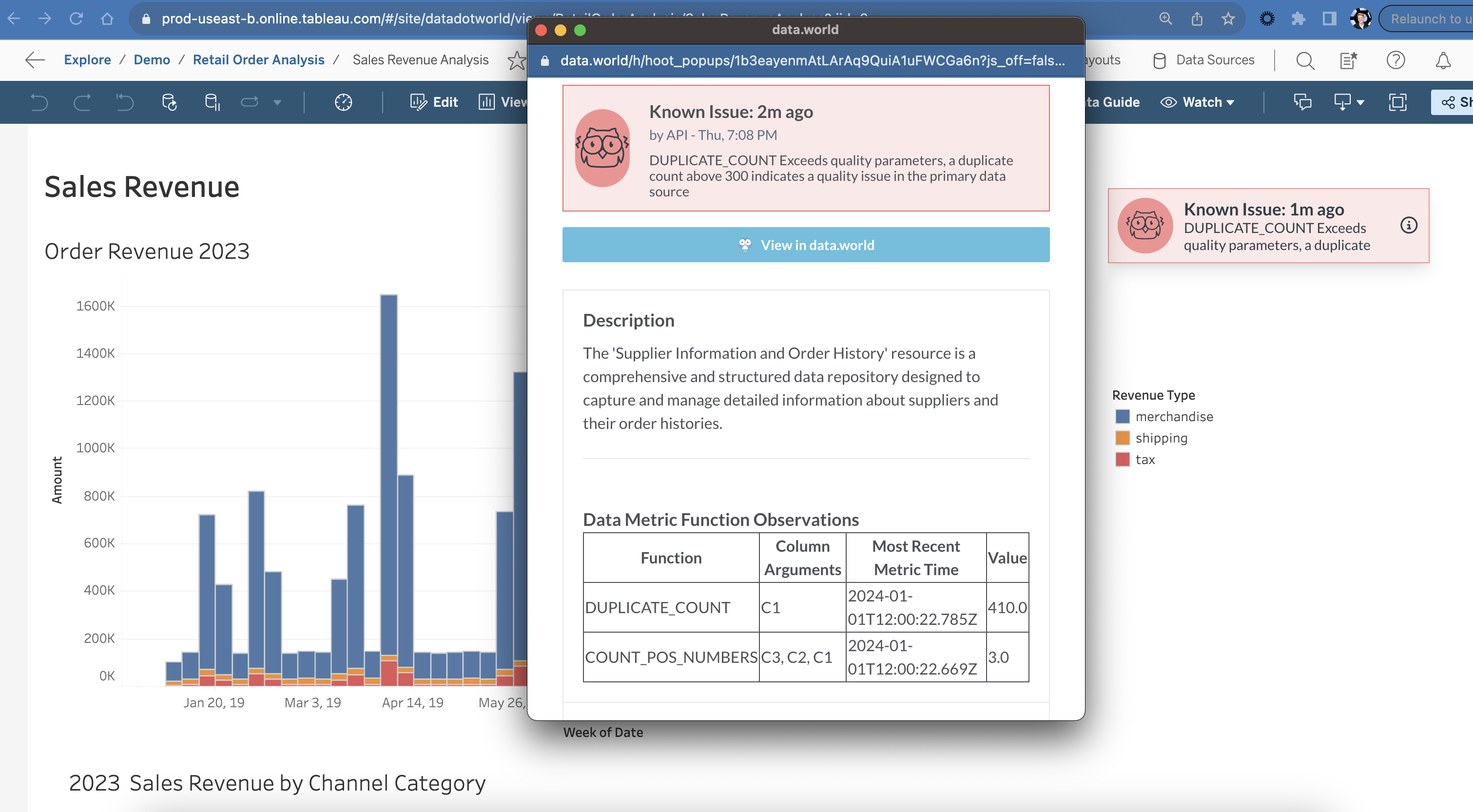
There are numerous advantages to integrating data quality into a Data Catalog platform. For more details, please refer to this blog.
Security: Analyze access control and governance through the lineage of Snowflake RBAC model
Data administrators use Snowflake Role-Based Access Control (RBAC) to control access and security in their environments. It enables admins to create granular levels of access controls for different Snowflake objects and resources.
In large enterprises, where deep and complex user hierarchies are common, it becomes difficult to understand the whole picture. Imagine a company has many departments, each one may consist of multiple levels of management and teams. Trying to understand the path of how each user was granted access can be a challenging task, even for a highly technical data administrator.
While Snowflake provides this information through Account Usage views, it takes a technical user who can write SQL to query the complex relational data. This is where data.world’s underlying Knowledge Graph technology can provide clarity by connecting roles, access permissions, and data resources with relationships. For example, you can model your advanced permissions structure in data.world’s knowledge graph, and then use our Eureka Explorer™ lineage graph to visualize user and access privileges for each Snowflake object. Now, you can easily visualize and answer questions such as who has access to what data objects, how they got the access permission, and detect abnormal access patterns with just a few clicks.
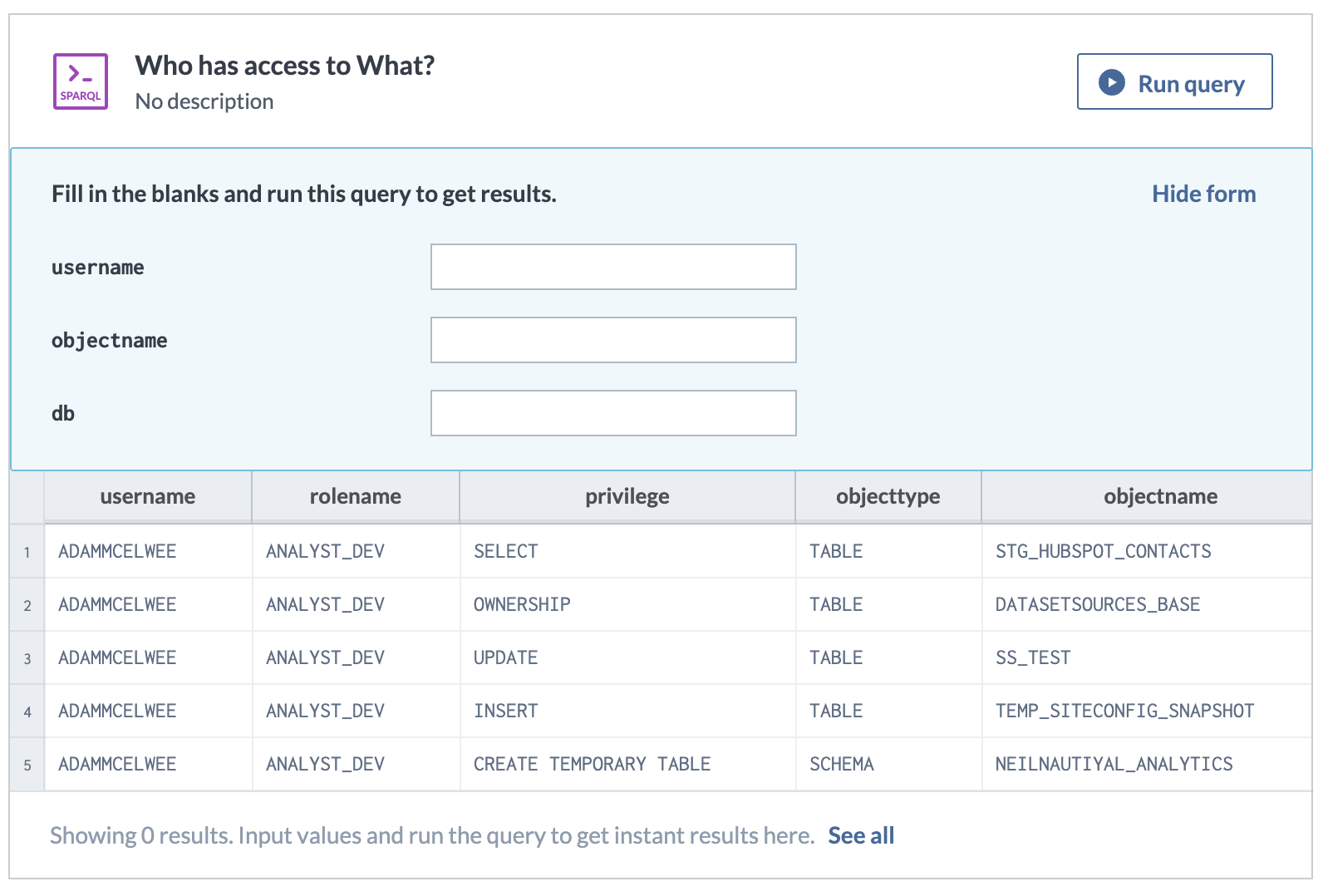
To learn more, I recommend checking out the blog: “How to use knowledge graphs to manage Snowflake RBAC” written by my colleague Anish Vaghasia. He outlines the reasons for needing a graph and provides the steps to effectively model your Snowflake RBAC access controls in data.world.
Privacy: Democratize insights sharing with privacy protection
It’s important to balance increases in insights sharing while protecting sensitive data. In addressing privacy concerns, Snowflake provides the following native governance features for their customers to protect sensitive data:
Object Tagging - attach descriptive metadata labels to categorize, organize, and manage data. When used in conjunction with Snowflake/3rd party Data Classification functionality, you can define policies to manage different representations of the data that users can view
Tag-based masking policies - enable dynamic data masking by applying the assigned tags to the data object
Access History - provides historical records of how users interact with the data cloud platform to understand who accessed what data, when, and how
While these native Snowflake Governance features provide the foundational building blocks, they are targeted at technical data admins. To enable broader usage of these powerful Snowflake features, we integrate them into data.world, with the following goals in mind:
1: Making Snowflake object tagging and policies easier to use
Both Snowflake object tagging & tag-based data masking policies are natively integrated into data.world. Any user can search and understand how they are being used, who is responsible, and which data objects (e.g. columns, tables/views, databases, warehouses, etc.) are related to them. In addition, users can request access to these catalog’s resources and suggest changes for the snowflake tags even if they are not the data owners.
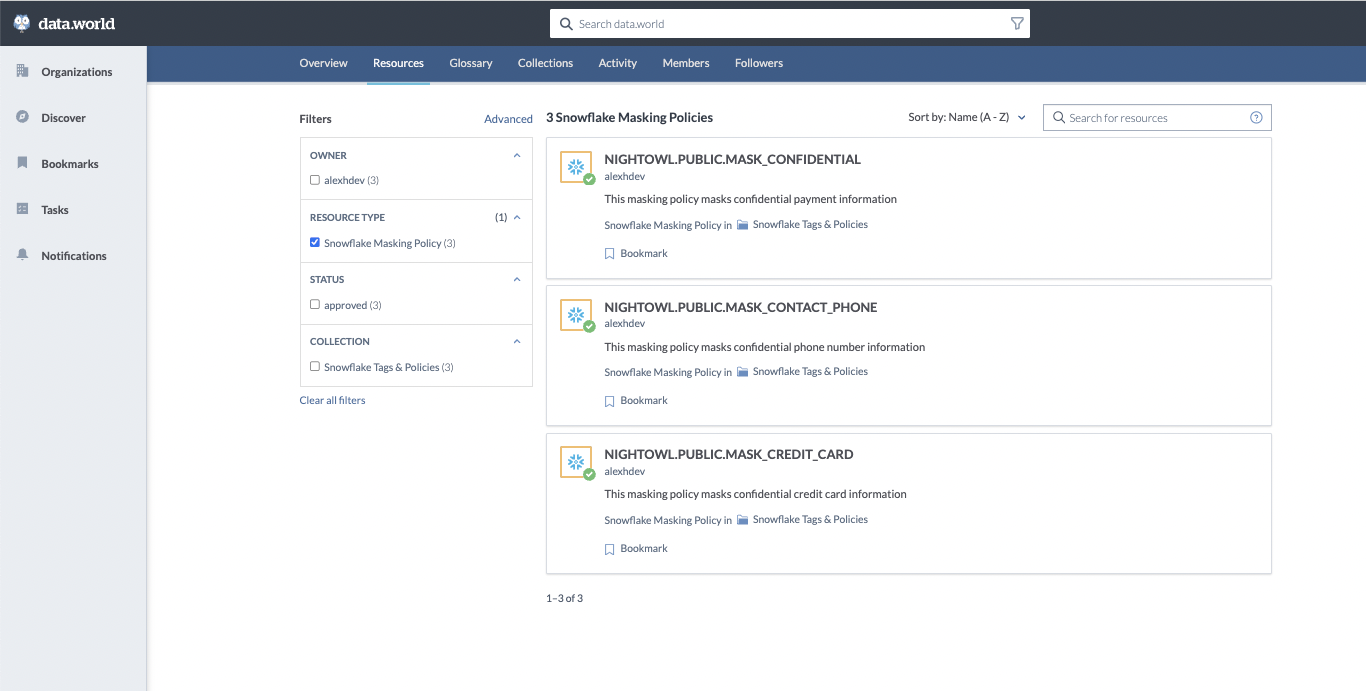
2: Enriching Snowflake metadata to derive additional insights
The Snowflake Data Governance Access History tracks activity including read and write operations on Snowflake’s tables, views, or any columns. By augmenting this additional usage data with data.world metrics, our customers gain deeper insights on the relative popularity of each data asset to the business. Data that is being used most frequently is likely more valuable, while low usage or unused data can be considered for archiving or deletion to save storage space and cost and improve efficiency for data discovery.
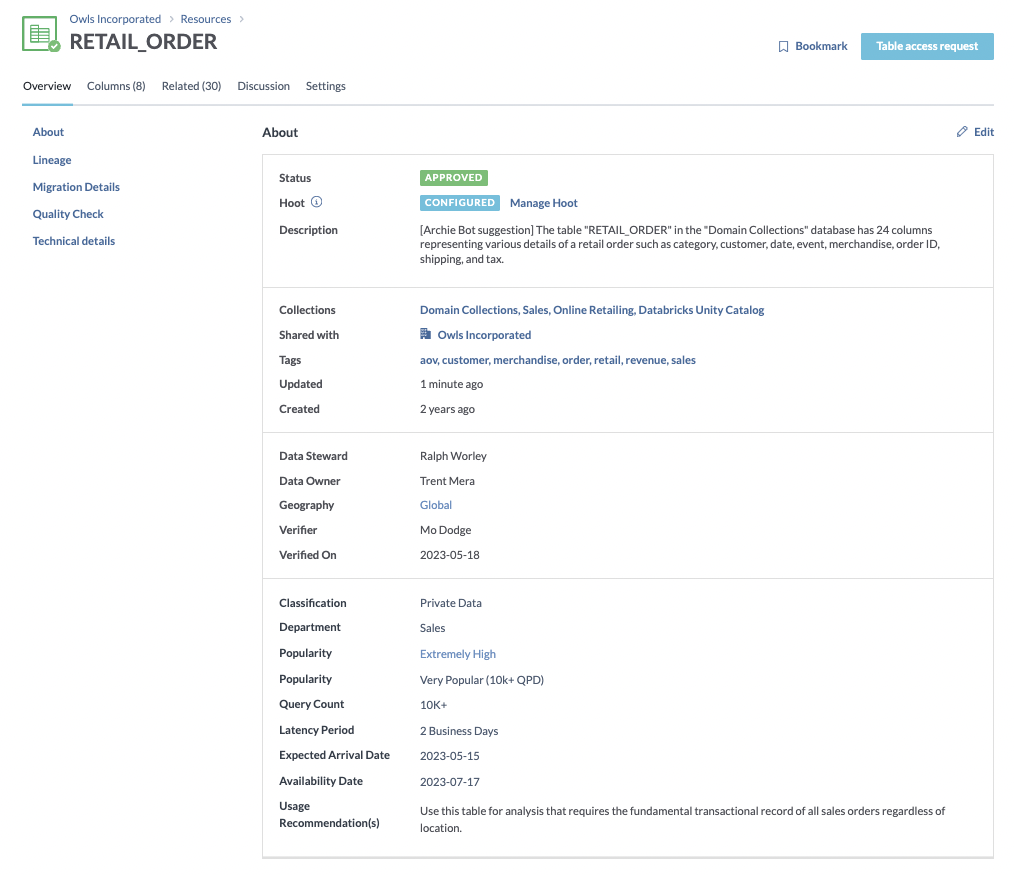
Interoperability - Enriching metadata from Snowflake and Iceberg catalogs
The recent announcement of Snowflake supporting Iceberg catalogs is very exciting. Now, customers can search across all iceberg tables to find and understand data residing in the Data Lakes, just as if they were natively in Snowflake.
data.world is best known as the only data catalog and governance platform built on a knowledge graph. This distinctive architecture sets us apart in the realm of other solutions. Since all of the metadata and data is logically organized in a graph, it is extremely easy to map data assets to key business concepts, bridging the gaps between business and technology. By integrating the Iceberg catalogs into data.world Knowledge Graph, we essentially unlock two things:
Improve discoverability across all iceberg and Snowflake tables in one place
Search in Snowsight is confined to each individual Snowflake account that you might operate. This constraint can be overcome by cataloging all your Snowflake assets across accounts into data.world. This cataloging will now extend to Iceberg tables as well - enabling users to search and understand the usage of the Iceberg tables across all Snowflake accounts.
Enable end-to-end lineage across different data sources with business context
By linking the technical metadata and business terminology in data.world’s knowledge graph, getting answers to critical business questions such as “which data products can I use to understand buying patterns and predict churn,” or commands like, “identify redundant third party data sources to save money,” are fairly straightforward.
Access: Sharing insights while keeping strong data protection intact
To provide customers a seamless and secure method for sharing data between accounts, Snowflake has introduced two features which work hand-in-hand to make this happen: Snowflake Secure Data Sharing and Marketplace Private Listings. Snowflake Secure Data Sharing ensures that no actual data is copied or transferred between accounts. When coupling it with private listings, data can be easily and securely provided to our Snowflake customers.
In a world where insights are the currency, data.world takes advanced analytics to new heights by sharing event and usage metrics data of our catalog platform through Snowflake listings with our customers. These metrics from data.world provide a profound understanding of how each data asset contributes to an organization's business objectives, including analysis of user interactions with the catalog, how each data resource is consumed, by whom, and for what purpose. The result? Customers now can seamlessly combine data.world’s metrics with additional information to unlock new use cases:
Build custom governance dashboards and reports
Conduct governance forensic investigations and compliance audits
Generate detailed data adoption and usage reporting
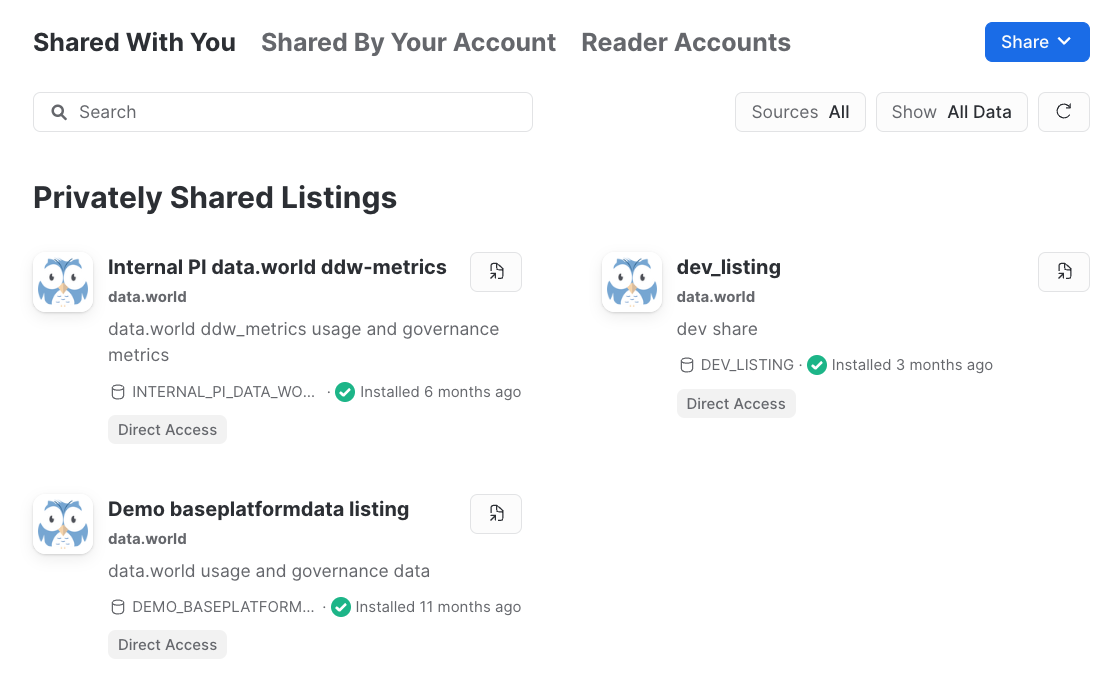
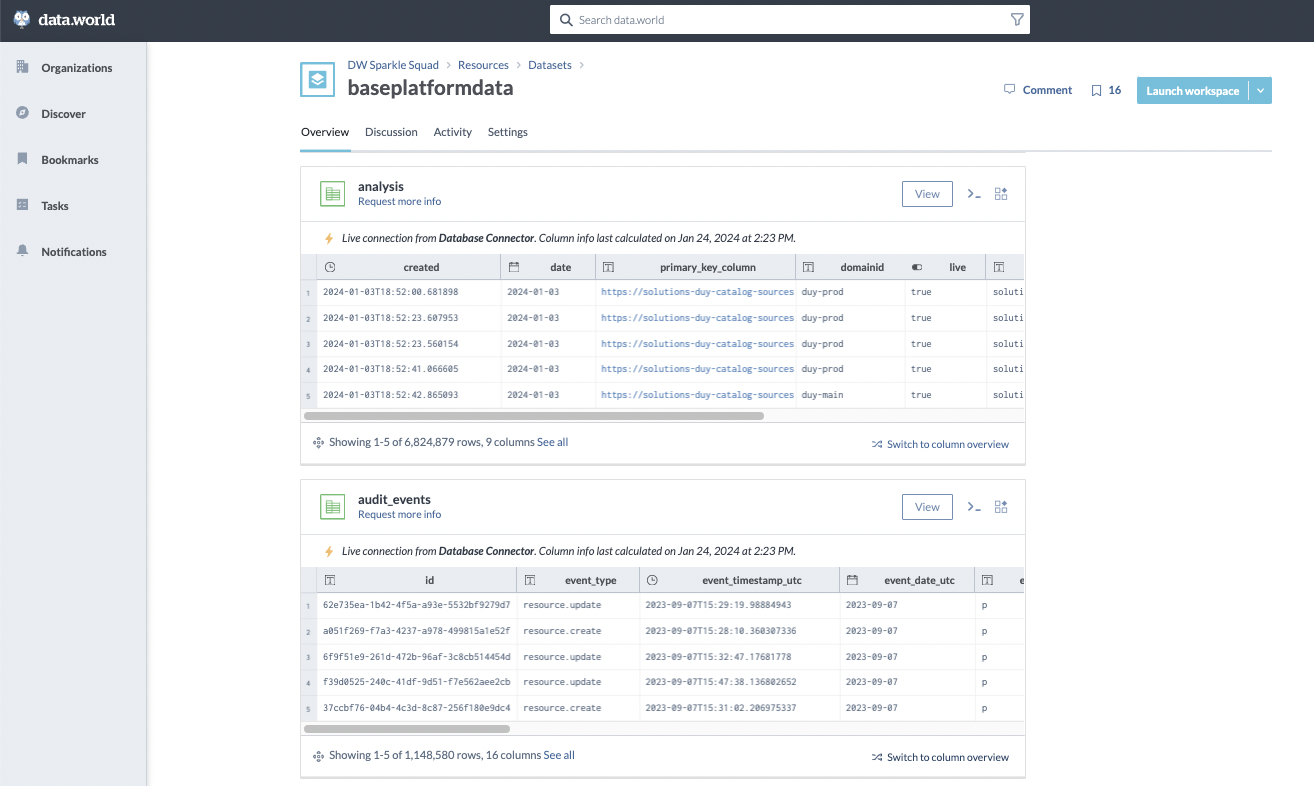
The integration of data.world and Snowflake provides a gateway to a more streamlined, insightful, and efficient data governance experience. As a result, our customers' data teams are gaining overall productivity in a closely collaborative environment.
Looking Ahead: The Future of Enterprise Governance with the power of AI
As technology increasingly incorporates Large Language Models (LLMs) into their data initiatives, data.world has been collaborating closely with our friends from Snowflake to accelerate AI adoption in a way enterprises can trust. Stay tuned for a series of in-depth articles that will delve into how data.world will continue to evolve its integration with Snowflake and AI. Meanwhile, if you are curious on how our platform is able to help increase generative AI accuracy by 3X, I recommend you to check out the Generative AI Benchmark research paper written by our own Dr. Juan Sequeda at data.world.