00:00:04 Tim Gasper
Juan, welcome. It's Wednesday once again, and it's time for Catalog& Cocktails. Honest, no- bs, non- salesy conversation about enterprise data management presented by data. world. I'm Tim Gasper, longtime data nerd, product I over at data. world, joined by Juan Sequeda.
00:00:19 Juan Sequeda
Hey everybody, I'm Juan Sequeda, principal scientist at data. world and as always, it's Wednesday, middle of the week towards end of the day. I mean, today we're doing an hour earlier, but it doesn't matter. It's Catalog& Cocktails, it's time to take that break and chat about data. And today is such an awesome, awesome day because we're going to have the one and only Ari Kaplan, who's the head evangelist at Databricks, was a former global evangelist at DataRobot, but he's the real Moneyball guy. Remember that movie, The Moneyball? That was based on all his experiences at MLB. Ari, how are you doing? It's great.
00:00:56 Ari Kaplan
Yeah, great to see you again, Juan. You and I had met at the CDOIQ event in Boston, hit it off, had lunch together, so really great to have the invite. And Tim, we had some great conversations leading into this, but doing well. You two live in Austin. Tim, you're in San Fran, I'm in Chicago. But yeah, great to connect. I love listening to your show. You've had so many seasons of great guests and success.
00:01:25 Juan Sequeda
Yeah.
00:01:26 Tim Gasper
inaudible.
00:01:25 Juan Sequeda
And I want to call out that we met because of Cindi Howson and from ThoughtSpot. Cindi has been a guest over here, so just really great that the whole data community gets connected. So anyways, cool, let's kick it off. What are we drinking and what are we toasting for?
00:01:41 Ari Kaplan
Sure, I'll start off. Well, I'm drinking honeybee latte from my local... It's called Bourgeois Pig Cafe, if you're in Chicago. It's a great place, great sandwiches, and I have in my McLaren Formula One mug. Spent the last couple years traveling the world, having an amazing life adventure, working with the Ray Strategy team. And who am I toasting? So anytime I get an open toast, I always dedicate it to a gentleman named Raoul Wallenberg, who's a Swedish hero during the Holocaust, rescued tens of thousands of people. And I still am honor of helping with the data- driven investigation into his faith. And when you do a toast, he used to toast people saying, " To life and to the future." So that's my toast.
00:02:33 Tim Gasper
Nice.
00:02:34 Ari Kaplan
I said that many times-
00:02:36 Tim Gasper
inaudible also a great toast.
00:02:36 Ari Kaplan
Yeah. How about you two?
00:02:38 Tim Gasper
I'm actually on the 45th floor in downtown San Francisco right now, so I'm going to toast to not being afraid of heights here and also second your toast. And actually I don't have a cocktail unfortunately because of how early it is, but I have a nice Sanpellegrino here, that I'll be drinking.
00:02:59 Juan Sequeda
Well, I went into my bar and as always, I'm at home and I'm like, what random thing is in there? And I just saw some cucumber vodka. I'm like, " Oh, what the heck?" So I put some... Actually had some cucumbers, I sliced up some jalapenos and I got some vodka cucumber soda, spicy and just cheers to that, Ari. Really, really enjoy that. So cheers everybody.
00:03:22 Tim Gasper
Cheers.
00:03:23 Ari Kaplan
That's an amazing concoction. It might go viral.
00:03:26 Juan Sequeda
It's actually really refreshing right now, and it kind of gives that spice of... Sleepy, will kick you, wake you up.
00:03:33 Tim Gasper
Cucumbers are great in cocktails and so are jalapenos, so you're getting the best of both worlds there.
00:03:38 Juan Sequeda
Yeah. All right, well hey, we've got a warmup question here today. So given your role as evangelist, we asked what is something that is not popular that should be evangelized more? Anything.
00:03:50 Ari Kaplan
Yeah, good question. And I expect more of these spontaneous questions, I welcome them. Maybe I'll give two answers. One is just general and then an actual thing. So general, I think more people need to evangelize and promote being vulnerable. A lot of people just on social media, when you talk at events, it's like everyone's perfect. And that vulnerability adds some... You don't want to hear too much information, but just admitting everyone's learning, no one's perfect, we're all pointing in the same vector direction. So vulnerability is something that needs to be incorporated more. And then I don't know if offhand, I love Opensource, but that is popular. Hundreds of millions of downloads. If you haven't heard of LangChain, that's one of the cool things, I met their CEO, Harrison and people... Probably heard of GitHub as a resource. You might've heard of Hugging Face, which is kind of LLM. And LangChain has had a huge uptick in popularity. So if you're into LLMs and you're beyond, which is a bit jaded by now, wow, it can write essays and stuff. If you're already beyond there and you want to actually do something and make something happen, LangChain is a website to help piece all different puzzles together. Kind of like GitHub in a way, to share code and stuff. So I like evangelizing that.
00:05:26 Tim Gasper
Nice. I think those are great ones. And I love that you mentioned vulnerability as well, I think that's a great thing that folks need to be more willing to do. It kind of reminds me of Radical Candor as well, in terms of being a little more exposed and being direct, right? Putting yourself out there.
00:05:44 Juan Sequeda
Yeah, I appreciate you bringing up that vulnerability. I mean, especially now you see everybody on social media just always talking about all the great things I've done, but nobody's talking about the things that have not worked. And one thing that also reminds me is something that I would like to evangelize more on this main is, I'm reading this book called Fierce Conversations. We need to have more fierce conversations, which falls into the whole attitude of just need to be honest and no- bs, please. That's one. And actually this is going to be a good segue to our conversation today, but I think at least I know we live in our bubble where we think about LangChain. That's what I hear almost every day. So I'm like, " I cannot believe that you haven't heard about it." But we've got to get ourselves outside of our bubble and realize there's a lot of that and people need to go learn. Which is a good segue, let's kick this off. So Ari, honest, no- bs. In our world, I hear lakehouse all the time, but honest, no- bs, what is a lakehouse and how is this different from databases, data warehouses, data lakes and so forth?
00:06:44 Ari Kaplan
Yeah, and it's also remarkable that not everyone understands or have heard of a lakehouse, but it's basically the modern data stack. Historically, you have data warehousing databases, which is structured data, numbers, categories, looking at what happened in the past. I used to work for Oracle at one point, as president of the worldwide Oracle user group. So that's the original paradigm. And then there's a whole separate paradigm like data lakes, which is unstructured data, videos, PDFs, word docs, machine learning, making, predictive algorithms, things like that. And there are two very separate ways to figure problems, do use cases. One structured, one unstructured. I would say the best way to do predictions is when you have multimodal data, you have data that's both structured and unstructured. And that's where this concept of the lakehouse or the data lakehouse comes into play, where it's one environment where you have all types of data in one environment. Streaming data too, with social media and the IOT. So you have all different data types, and that way you can make most likely, the best insights. So that's one thing, is its one platform and there are many vendors doing this. No sales, no- bs, but historically my company, Databricks was the creator of it, but now it's a whole big marketplace with a lot of companies doing it. We've been founded proudly so on Opensource, which maybe we'll talk about now, but yeah, that's what the lakehouse solves, is you have one umbrella, one governance instead of having some password control for the data warehouse and the data lake. It's just one environment. You understand the lineage from the open to the end, how it all flows. So there's a lot of governance play. And the other nice thing is based on this parquet delta lake it's called, the performance is astronomical. When I worked at Oracle, we're talking about millions or maybe billions of records, and now you're talking about hundreds of billions if not trillions of records, which is needed when you have somebody doing some generative AI or doing some query, you want the response to be in real time. You're playing a video game and you need to be matched with your opponent in under a second, the speed of which it's done is incredible. So that's kind of what the modern data lakehouse is.
00:09:39 Tim Gasper
Okay. I think that's actually a very helpful explanation around it. And you're right, I remember the first time I ran into the lakehouse concept, I believe it was Databricks, maybe this was back in 2017 or something like that along those lines. It was maybe even earlier than that, where folks were talking a lot about data lakes, but then I think folks were starting to hit some of the struggles around Hadoop and things like that, some of the big data excitement was starting to fade. And my interpretation of lakehouse, and I'm curious if you think that this is a good way to think of it, is you kind of talked about those two modes. There's sort of the structured data warehouse, and then there's more of the data lake, unstructured, maybe a little bit more large scale kind of thing, that the lakehouse is kind of, hey, can I have the best of both worlds? Can I bring that together in a smart way where I get the benefits of each? But as you mentioned, in a single governed kind of platform.
00:10:38 Ari Kaplan
Exactly. And a unified platform, you get all those benefits and then there's so many things we could talk for hours, but you could do SQL now. I should also say I am technical, so I've stood up Hadoop clusters. It's a pain, it's complex, it took me quite some time to get it right and working, but now it's a bit democratized too, it's easy enough. You could do SQL against any end data. You could do Python or Scala against where the target is. Structured, unstructured, all unified, which something to be said about democratization and simplification too. Exactly.
00:11:21 Tim Gasper
Yeah. It's funny how all of this, going back to the beginning of Hadoop and things like that started without it really being about SQL, but now it's all kind of come back to things like Parquet and SQL and as you've mentioned in some cases, really, really good performance. Can you go a little bit more? You mentioned about AI, right? And how the lakehouse can be pretty helpful with that. Obviously AI is a big focus for a lot of organizations now and how they can take advantage of it. How does a lakehouse support a company's AI strategy and what they need to do there from a technology standpoint?
00:11:59 Ari Kaplan
Great. Well, I'll start by saying how do you define AI? At least generally speaking, you have your modern LLMs, which is kind of all the rage, so that's generative AI. How can you take a lot of information and give some insights, whether it's text or artwork or things like that? Which could have incredible use cases, especially when it's based on your own company data like, " Hey, what were my sales the last couple of quarters? What do I predict it to be?" " My mother- in- law wants to go into surgery. What's the best doctor my zip code?" So that's generative AI, and people shouldn't lose sight or should also be aware of traditional AI, which is how do you make predictions based on something in the past? What's a pattern that could be in the future? Transparency of that, of what features or variables are helpful for predicting? And then classifications. Is something likely to occur or not? What type of artwork is this? What type of customer is this? What type of lifetime value? So all of those use cases are all perfect for the lakehouse. So when I said multimodal is generally the best to do AI... So if you just have a data warehouse and you just have numerical type of information, you will get predictions, they may very well be very good. But when you start adding in text and other information, it won't hurt the model, but it's very likely that the model will get more and more accurate. And vice versa, if you only have video, you want to have some tags in the video, which are numerical tags. So the more variety of data, kind of going back to the Vs and big data, the more volume and variety of information you have, the more likely you could do better predictive analytics, which is part of AI. So those are high level. And then the lakehouse itself lends itself very, very well since the data... The other thing we didn't mention is not just the time, but the cost. If you have two separate systems of copying data all over the place and securing it, if you have to keep copying data, that doubles, triples, exponentially grows. But if you could do AI on data of where it exists already without having to move that data, much cheaper, much less storage costs and potentially lower compute costs dramatically.
00:14:41 Tim Gasper
Yeah, you don't want to have to be duplicating the data a lot, especially when you're talking about really large data sets, which may be leveraged for some of these either AI models or machine learning models. And I know obviously you can use SQL and things like that with lakehouses, but I know a lot of lakehouses such as Databricks for example, have a lot of tools for data scientists as well, right? Is that a key aspect around lakehouse as well?
00:15:08 Ari Kaplan
Exactly. And that's been a large part of my career even before Databricks, is how can you make tools for the data scientists? You want to make people both more efficient at least, in the way that the boring and repetitive and time- consuming parts of their job gets automated, so everyone gets elevated to do more of the complex work. So yeah, the whole simplification. There's another market called auto machine learning or auto ml. So that's one part, is how do you automate whether it's the workflow, ingesting raw data, making, it's called the medallion. Silver, bronze, gold, and so on. Building features upon features or variables based on other variables. All of that is perfect for tools for the data scientists. And then above and beyond that, the whole unity catalog, the whole unification. When I started with baseball, I'm sure we'll get into that, it was a one person show. I had to do everything, which means I wasn't collaborating on the programming. But now you have teams of 10, 20, 100. You have teams with thousands of data scientists and data engineers, so it lends itself to more of a collaboration platform. So if it was the three of us, we'd all be working together. Juan writes some Python code and shares it with me, et cetera, we all can share. So one part of it is the collaboration. And then the other cool thing that's just now happening, just really starting out in the industry the last couple of months, are using gen AI to help with the coding experience. So before, you would type and it would autofill, select star from... It would autofill the table name and stuff like that. Then the next version is as you type, it brings up ideas of what other people at your company have done. And now using gen AI, it thinks of other questions other coders at your company have asked. So it's like using AI as an intelligent assistant for coding, for writing whole programs. And most of the world when you watch television just thinks, hey, it's writing essays at my kid's school, but there's a big chunk of that that's doing code generation. Write a Python code that does this and add comments, stuff like that.
00:17:49 Tim Gasper
It's not just for your book report.
00:17:50 Ari Kaplan
Exactly.
00:17:50 Tim Gasper
And I know we've been experimenting a lot with code generation at data. world as well, both internally as well as customer facing stuff, and it's pretty incredible. There's a huge productivity gain.
00:18:04 Ari Kaplan
Yeah, absolutely. And Juan and I were talking at our lunch with Cindi Howson and others about you have this incredible AI lab, I'd love to see more of it someday, but what's real, what's not, what's at the leading edge?
00:18:17 Tim Gasper
Yeah. Well, I know we want to go a little bit more into talking about gen AI and code generation, and also I know we definitely want to come and talk a little bit more about sport as well, because a really fun topic and you have a lot of history there. But before we do that, just to cap off on some of the lakehouse topic, I think we talked a lot about some of the benefits around lakehouse. Honest, no- bs, that's part of our conversation here. I want to ask, can't lakehouses also be more complicated? Because I know that even though databases are... A more of a structured database, can be a little bit limiting, can be more sort of single mode in some cases. Obviously lakehouses, they might have clusters and zones and it can get a little bit more complicated. Talk about that. Are there some downsides there, and how do you mitigate against that?
00:19:10 Juan Sequeda
One thing I want to add to this is that if we look at history, it's always pendulum swings. So it's themes that we've gone to. We're going on this pendulum swing to one side, now I'll add everything and where does it end? And is it going to keep going somewhere or is it going to swing back here? So I'm curious to get your perspective about this because the follow- up on this is, so what's next? What is the lake house then missing, that you can argue that says, oh, it needs to also have this other thing? Or is it now" complete"?
00:19:40 Tim Gasper
Right. So what are the pitfalls of lakehouse? And then have we reached the destination or is this a stop on the way?
00:19:47 Juan Sequeda
That's a good way of putting it, Tim.
00:19:49 Ari Kaplan
Yeah, great way to put it. Again, it's like a dichotomy of what we call personas. You have technical people where they don't care if it's simple or not, they want to write their own code, Python, whatever, RSQL, pick your language of choice, Scala, et cetera. So they just want to dig in and don't worry about gooeys or anything like that. Then you have the whole democratization. And this is kind of the question or the challenge that I've had with every software company that I've been at, and was at Oracle, DataRobot, Databricks now, is what personas do you gear towards?
00:20:36 Tim Gasper
More technical, more business. And even those are too broad, right? Really, there's much more specific personas.
00:20:42 Ari Kaplan
Yeah, yeah. Like persona data engineer, data workflows, data scientist, end user, business user. Put in a link from Microsoft Excel, so you could do stuff. So yeah, the best technology is if you have a little for each. So if you want to roll up your sleeves and really drill down, then it makes things as easy as possible. If you're in a notebook, which is a programming environment, how can you make it as extensible as possible? How can you seamlessly plug it in to third party solutions too? Opensource is another thing we should bring up. Opensource is something that opened my eyes. When I joined Databricks, our founders created... We're the creators of Apache Spark, Delta Lake, MLflow, which I thought would have hundreds of thousands of downloads, but they have over a billion downloads per year, is the rate, which is insane. And Opensource, I'm a big fan of even more so now, since you have the whole community contributing to it. So you have really smart people and hopefully the best ideas bounce up to the top. So that's one thing that's the technical side. How can you make them more efficient so it's easier for them to code quicker, debug quicker, see the workflows quicker? When they're doing an AI model, they know what the source of the data was like. It's called lineage, all the way up to what models are being used, how is the data drifting? Should I recalibrate my models? But then you have on the other end, non- technical people that you'd want to enable to do data science like things. And one reason you want that is for every data scientist... I conducted the study in my last company, we looked at LinkedIn job titles. For everyone with a data scientist or very similar job title, there was 30 people who had business analyst titles. So if you enable them to do at least beginning intermediate level data science type questions, you just increased your value 30 fold and even more so for Excel users. So the democratization is great, but that case you still need people who know math and probability, to make sure it's guided correctly. Then there's that whole semantic layer which helps with that. When you have a non- technical person saying, what were my sales last quarter? You want it to know what is a sale? Does it include returns to Nordstroms or not? What is last quarter mean? Stuff like that. So I would say, yeah, it's a stop along the journey. If you were to see all the great things coming out and all the innovation from Opensource, from all the companies in our space, innovation is happening faster than ever, and I don't see it slowing down for years to come. Definitely where we are now, you can add incredible, tremendous value. So every company where the lakehouse is now, will be incorporating a lakehouse technology in the coming years, but it'll get even better over time. There's inaudible now and then even more I foresee in the future.
00:24:20 Juan Sequeda
The way I'm interpreting this and kind of thinking about it is, the core principles behind what's being called a lakehouse is just being able to go integrate all types of data, the multimodal part, right? And then from there you have all these types of... There's all these workflows and stuff around that stuff and all these types of applications you want go do that. So there's the foundation of being able to go bring in all these different types of sources, and then there's the management of that and then doing things with that. And then eventually the vendors will say, " I'm going to have this one- stop solution for everything." Or the other vendor will say, " No, I'm more at the lower layer." And then there's other vendors will do more the workflow processing part or application. So I think that's how we're going to be starting to see how this ecosystem moves on. I mean, that's my interpretation. Would you agree with that or disagree?
00:25:08 Ari Kaplan
Yeah, it's an ecosystem. So I think-
00:25:12 Juan Sequeda
Ecosystem is a great way to put it.
00:25:13 Ari Kaplan
Yeah, I think companies are going to want to standardize on one primary lakehouse environment since you want to have the same governance, auditing, controls, traceability. You don't want to move data back and forth. So the primary core of the lakehouse, companies will want to standardize. But then there's a whole bunch of things around that. The visualization, semantic layer, applications built on it, domain expertise. I'm missing a lot, but to build upon that whole ecosystem.
00:25:53 Juan Sequeda
And by the way, to bring up a statistic, because I did this also, I just found it here. Last February 2022, I looked on LinkedIn for US- based employees for large companies like General Motors, 3M, Coca- Cola, General Mills. And what we saw was that 3% had some sort of a data title. 3% only. 97%, those are the non- data people, don't have a title of data. I'll argue that everybody's a data person, right? Or should be in a way. But anyway, that's to put into perspective. So talking about we're in our bubble, I think we live in this bubble of the 3% sometimes, we got to really get out of it. And then hence our original conversations, can't believe that people don't know what a lakehouse... Well yeah, because we're inside that 3% bubble right now. But anyways, let's get out of that 3% bubble right now, and I would love... I mean you're the Moneyball guy. Share us your experiences, this would be fascinating, about kind of everything that you had gone through before everybody talked about to do all those sports analytics, you need data, which is just inaudible but also streaming and visual, all these things. Just share us your experiences. Love to hear this.
00:27:12 Ari Kaplan
Yeah. Well, I've been very honored, fortunate to have worked in sports analytics for many decades. I started back in the 80s as an undergraduate at Caltech, which is the Big Bang Theory school, if you like that. They own and run Jet Propulsion Lab, so kind of brainiacs. And they have the summer undergraduate research, otherwise known as SURF program. And just being a fan ever since a kid and being kind of mathematically minded, just would observe some players that I thought were great, would have bad statistics. And some players that I knew would be blowing games, they would have great statistics. And a lot of people in sports made complaints about it. I was one that complained, but then came up with the actual metrics that improved upon it and tried to do it in simple terms so that I could go on CNN, the Today Show and stuff like that and explain it to people. So one of the things that lasted true through today was that anytime you see the letter X in the statistic, like expected goals, expected wins, in any sport, was the paradigm that I started way back when. That was that research. Still remember giving the keynote, it was such an incredible single point in time, a bunch of Nobel Prize winners in the audience, industry titans, Gordon Moore from Moore's Law, I could go on and on. And just hearing me talk about sports analytics. And the owner of the Baltimore Orioles was in the audience, he helped me out. I ended up also getting a call from Fred Claire, who's the GM of the Dodgers, happened to see me in the LA Times and just invited me out to Dodger Stadium saying, I don't know yet... Speaking of vulnerability and humbleness and leadership, he said, " I don't know if what you are doing are helpful or not, but I want to learn more. Anything that can help me do my job, I want to explore." So he was humbled to say he may be willing to learn, but he was intentional enough to not just say, " Hey, what you're doing is great." He just wanted further evidence. And then the rest is kind of history. Maybe one giant lesson learned is every four years roughly, I pretty much had to reinvent myself since all the good ideas that would come out, other teams would hear about it or people would switch jobs and they would bring those ideas with them or try to do it on their own. But when I did start out with the Dodgers, there was only four people that I had heard of, that were employed doing anything data analytics with sports organizations. And now fast forward many, many decades, it's a whole industry. There's college degrees, there's multiple tens of thousands of people doing that industry, but still have to keep growing and iterating every couple of years. And so we could talk about where the data and AI is now, but yeah, the journey at the beginning was, there was almost no data. I had to go to a library, get microfilm, hand enter play- by- play data into my own database and try to by hand, come up with stats. So it would take me a summer of coming up with... Probably could be done in a day these days.
00:30:44 Tim Gasper
Yeah, that actually was going to be my follow- up question here, is that you didn't have the benefit of massive online libraries of data exposed by API and things like that and just things that you can tap into today much more easily. Also didn't have modern data tools, a modern data stack. What was that process like when you really got into doing the econometrics and stuff like that around and then Sabremetrics, et cetera? What kind of tools were you using? How did you approach that process?
00:31:14 Ari Kaplan
Yeah, have you heard of Paradox? Borland paradox, it's an old, old-
00:31:20 Tim Gasper
No, I haven't.
00:31:21 Ari Kaplan
... old,old database. It was before Microsoft Access came out, but that was a big deal on the computer. So when I say we, first we just did that to create play- by- play data and then we could study things like what relief pitcher would be best to be brought in at a certain time or what batter would be used. So super fun, when I was still a teenager, I was working with the Baltimore Orioles. They had heard of my stuff with the Dodgers and hired me there, and Earl Weaver was kind of... People think of him as an old school manager, the managers that kicked dirt into the umpire's face and stuff and argue. But he was one of the innovators in data analytics in sports. He was the first person to know what's called a split. If you're a left- handed batter or right- handed batter, left- handed pitcher or right- handed pitcher, he saw that there was a difference, there was an advantage by having certain match ups. So he had these famous Earl Weaver index cards that he would prepare before the game, bring into the dugout, so late in the game, who do you want to pinch hit with? And it pretty much worked. The media roasted him by the way, kind of what Moneyball, was you get kind of made... And maybe people listening are doing the same thing. Sometimes when you make innovations that help, you get ridiculed or people don't want to change. But Earl was the opposite. He's like, " I don't care what the media says, if we make the playoffs they're going to love me." So one of the awe- inspiring things I got to do is automate the index card of the Earl Weaver paradigm with the Orioles, coming out with math models to help them with the lineup. Frank Robinson became the manager, he is a hall of fame player. Roland Hemond was the GM, hall of fame general manager. And then we started winning. And even if we weren't winning, the method was still valid. And then people would hear of me and I'd get hired to different organizations. So that was early on, and then we kind of ahead of the time, multiple decades ahead of the time, wrote the first database for scouting reports. And the reason that that's relevant today is scouting reports are text with some numeric information where the scout is just saying, what do I think of Tim Gaspar's performance? And like, " Oh, Tim's great. I know his father. He has great abilities, but Ari is inconsistent and lacks the ability. He has a good heart, but he lacks athleticism." And these are all on paper, so making a database in that paradox program made it so scouts can enter the reports, put it into a centralized database. It was really before Oracle came out, believe it or not. And that way the general manager could just general AI ask a question, who are some good third basemen in the American League that we might want to sign? And then evaluating how good the scouting reports were. And even now actually working with the Texas Rangers, there's a great article that came out a week ago, a blog that they wrote. They now use for example, gen AI. The tactics are more modern, but the idea is the same. Scout writes information, there's injury reports. Just tell me the summary. What do we think? Is he capable of playing in the majors? What's the likelihood? Using gen AI now. So that's kind of been the journey and along the way... Really, the last eight years has been a huge revolution in measuring biomechanics. Everything that goes on in the field, every limb of a player in live, hundreds of times a second. So it's like moving dots, and you get the signature of a player, ability to make improvements, velocity of pitch, spin rate of the pitch, command of the pitch, how you approach your swing. So when I started out, you could just say, did the batter swing and miss or put the ball in play? It was bullying. Yes or no? But now you could say he swung over the ball by six inches or by 2. 5 inches. He was a little bit late. So you get way more precise insights.
00:36:05 Tim Gasper
There's way more variables now and much more data inputting into things.
00:36:10 Juan Sequeda
So listening to you, two things come to mind. One is history, right? It is so important to really understand our history and what we've been doing. Because a lot of the things that you're describing and either what people wanted and what you were able to accomplish is what people are talking today. And sometimes a lot of folks, especially a younger generation, thinks it's the latest, greatest stuff. I'm like, "No, no, no, no. Hold on, hold on. People have been working on this stuff. It's actually working." Things have been advancing. So just know your history, because otherwise if you don't, you're just going to be repeating bunch of stuff and then we're not making progress. We're making progress, but wasting our time in a bunch of stuff. So that's one thing which I really appreciate listening to you. The second is to go through everything that you've been discussing, you had to have a lot of knowledge, context about the sport, baseball. And I think just putting in my personal interest and bias here is about knowledge. It's not just about give me a bunch of data and then just feed it into this model, whatever. Just give me more data, give me more spreadsheets, give me more data, and just hope it's going to work. No, no, you actually had to understand the meaning, the semantics of knowledge around this stuff. And I feel that we live in this world, going back from pendulums. We swung to a pendulum of no, I just needed more quantity, more volume and variety, but we're missing the quality, the knowledge, semantics. And the issue is that that kind of contradicts, I just want more because you got to invest in these semantics. You got to invest in this knowledge. So I see this kind of... I don't know if it's a contradiction, but it's like you need to find this balance around it. So anyways, I'm sorry to rant. I'm going to shut up here, but does this make sense? I'm inaudible
00:38:00 Ari Kaplan
Yeah, 100%. It is not a rant, it's awesome. Yeah, it's where you still need the collaboration of people who could actually do data science or AI modeling, and people who understand both the domain, how's the business run, and people who understand the human aspect. And I don't mean that as cliche. So for example, I did a lot of work with Nielsen and IRI, and sometimes the model would recommend, your agreement with Walmart is inefficient, therefore don't sell your product in Walmart anymore. And then the salesperson or the business rep would say, we have a five- year deal with Walmart. Even if we want to, we cannot cut it. So your models can recommend something that the reality of your business just won't work. To do a sports analogy, if you have a player that's injury prone and the model says to reduce the injury risk, have them become less effective by throwing the pitch easier, it's going to make the pitcher less vulnerable to injury, but it's also at the same time going to make them less effective. It's like how do you optimize the best performance and injury and health of the athlete to the most effective? So you're spot on, Juan, of having people collaborate, who know the business and can ask the right questions and know how to paint the picture. When you do have an insight, how do you get real life people to take action? And that's all part of communication and being able to relate to the people that can actually affect the real world.
00:39:50 Juan Sequeda
This is what we need to talk more about, because I think we live in this world, especially going back to that 3%, right? It's like, " Oh yeah, just give me data. I know how to go do this. Give me your data." I'm like, " Wait, there's the 97% rest of your organization. How much are you talking to them? Because they probably know more than you do."
00:40:12 Ari Kaplan
And you have to be vulnerable and humble enough.
00:40:14 Juan Sequeda
There we go.
00:40:14 Ari Kaplan
Yeah.
00:40:15 Juan Sequeda
Hey, I love this. We're tying all these topics together.
00:40:20 Ari Kaplan
Here's what I propose, does this make sense? How can I make it more relevant to you? Stuff like that.
00:40:25 Juan Sequeda
And I think it goes back to what we were talking earlier this week, you brought it up in the sports is oh, the end users, the general manager wants to be able to ask this question. And they're like, now we're talking about this in the AI, now generative AI world, is like, " I want to go chat with my data on these things." But I'm like, " Well, one thing is that you want to be able to go chat, but you also want to understand where those answers come from, you're able to go explain these answers and the explanations would be different." So I think there's a lot to be learned here about what we've been doing in the past, but also it's like there's all this context, all this people around that we need to figure out how to go tight. And it's not just throw it to the machine, to the AI and we'll do it all correct, you can trust it. So I think that's one of the big kind of just flags on waving out here.
00:41:13 Ari Kaplan
Yeah, nice.
00:41:16 Juan Sequeda
But any final thoughts? Because I know we want to go into our AI minute, other questions and stuff like that. But just to kind of wrap it up on here, just your final message you want to give to everybody. I'll tie the lakehouse, the sports, the AI altogether.
00:41:34 Ari Kaplan
No, it's great, just always constantly be learning. The technology just keeps... There's new ways of doing things. Educate yourself. Always strive to learn. I know myself, I keep trying to get certifications and learn the latest technology and feel free to jettison stuff you've done in the past if there's a better way to do things. And also join organizations, join affinity groups, go to conferences, learn from people. If you haven't spoken, share your experiences. So that's kind of one of my messages out there.
00:42:13 Juan Sequeda
I love this. And I think one of the key takeaways I'm having out of this is that vulnerability. I think it's important.
00:42:21 Ari Kaplan
Nice.
00:42:22 Juan Sequeda
All right. Let's go to our next segment here, the AI minute. We've already talked a lot about AI, but let me just put in here stopwatch. One minute to go rant about anything you want about AI. Ready, set, go.
00:42:35 Ari Kaplan
All right, rant of AI, oh my god. A lot of people just focus on what you shouldn't be doing, which is a great concern. But I like to start with what's the potential for humanity? What's the potential for an enterprise business? How can this help personalized health medicine? How can it help humanity? And those are the things I actively want people to think of the strengths and the positivity. And then once you see where do we think it can help, then we're in parallel figure out the limitations and how we can do things safely, securely, and so on. But yeah, the genie is out of the bottle. Let's help humanity as best as we can.
00:43:20 Juan Sequeda
Love this. Thank you so much. All right, lightning round questions. Here we go. I'm going to start. First one, do all companies and use cases need a lakehouse or can you do things without it?
00:43:37 Ari Kaplan
I would say you if you have small data, maybe not. If you have large data, variety of data, which is basically any Fortune 500, S& P 500, Fortune 2000 type of company. If you have data and you want to get insights from that data, then yeah, you will want a lakehouse. But the larger the data, the more value it becomes.
00:44:09 Tim Gasper
That makes sense.
00:44:10 Ari Kaplan
The more people you have on your team, the more valuable that governance becomes.
00:44:14 Tim Gasper
Yeah, I think that's good guidance. Second lightning round question. So back when you were focused on sports analytics, I feel like it was a little more controversial, whereas... Even though the media will still sometimes poke fun at the analytics guys and stuff like that, right? Do you feel that's flipped around? AKA, is sports analytics and data in sports a given now?
00:44:41 Ari Kaplan
For baseball and the NBA, I would say absolutely. You have teams that are pushing 50 people in the data engineering through data science, 50 people. When I started with the Cubs, I think there was a dozen people in the whole front office including the GM and scouts, and it was like we went from zero to one of the analytics. The owners have seen the success of being data- driven, managers and so on. So yeah, I think it has flipped around in some of the sports. In some of the others, you still... Maybe the NFL, some teams in international racing, maybe some soccer teams where it hasn't been proven out yet. There's still room to grow. But yeah, overall, yeah, tens of thousands of people in the industry collecting data, vendors, huge ecosystem. So that's pretty wild to see since there's a lot of resistance early on. People wouldn't even want to sit down to even know what they're disagreeing with.
00:45:50 Tim Gasper
It's like, no, no, no, no, I don't want to hear it.
00:45:54 Ari Kaplan
Yeah.
00:45:54 Tim Gasper
It's interesting how different it's by sport too, that's very interesting.
00:46:00 Juan Sequeda
I'm going to take a quick little parentheses on the lightning round question. When it comes to sports, I am curious, how does this whole approach change from sport to sport?
00:46:10 Ari Kaplan
Yeah, so baseball started out more analytically driven. It's more discrete events. You can measure a pitch. It's really about how deceptive the pitcher is and how the batter can overcome that deception, and then you have some fielding and base running in between. But things like football, like the quarterback, you could do metrics, how the mechanics are of throwing and passing, but it's really more of an interrelated team sport or international football/ what we call soccer where you get zero to one, maybe more goals. So defining in economics a path to purchase, there the path to a goal is few and far between so it's more noisy, how do people interrelate? Which is definitely more doable but harder to do.
00:47:03 Tim Gasper
That is super fascinating. Yeah, because American football, for example, is a much messier system and success is a lot harder to analyze there. Whereas between a pitcher and a batter, it's much clearer. Either you hit it or you didn't and where did it go? And so success is a lot clearer, right?
00:47:23 Ari Kaplan
Yeah, exactly. And who do you attribute success to? So if you are a quarterback and I hand it to Juan and he's a foot from me and he runs 90 yards, I'm the quarterback, I get a 90 yard touchdown pass to my credit, even though he did all the work. Or if I throw it... I don't want to use Tim. If I throw it to someone named Ari who's wide open in the end zone, it bounces out of my hands, no one's even close to me, as a quarterback, it's an incomplete pass and I get charged. So that's where the team work and how do you ascribe skill versus luck is a challenge. That also drives back to AI. You want to see which variables are luck and which are actual causal or value. And that's the challenge in every AI problem, and that's even more so a challenge in sports, which athletes are... If you look next year, what will they be like? Don't look at the results as much.
00:48:33 Tim Gasper
Side note to our listeners, I feel like we need to have a second episode at some point where all we do is we talk about this topic, because now my brain is thinking about attribution models and stuff like that and oh, there's so much we could do there.
00:48:43 Juan Sequeda
The thing is that not everybody's going to agree too, right? So then this goes back into the holding knowledge and the people, what do you think? So there's like, if there's not even an agreement, then yeah, how do we figure out how to go forward here because we still need to go forward somehow.
00:48:58 Ari Kaplan
Yeah, MTA, multi- touch attribution. Marketing people still struggle with that. What tactics work to make a sale?
00:49:08 Tim Gasper
Yeah, you saw an ad, you went to the conference, you talked to a salesperson, how do I attribute?
00:49:14 Ari Kaplan
Yeah.
00:49:14 Juan Sequeda
That's why one thing I've learned and I always tell people is if you want to go start, figure out that in the data within organization, go to marketing. Their hands are all over the place. All right next question, getting back into the AI stuff. So will generative AI take over most of the code generation for engineers in the near future? Note that I'm saying code generation for the engineers, not taking away engineers jobs.
00:49:41 Ari Kaplan
Yeah, great question. I would say overall, yes, from what I've seen, and it's still not even a year into it, that co- gen is pretty cool. A lot of times it's working. But what I kind of foresee, there's a great speech at our Databricks conference with Eric Schmidt, one of the innovators where basically the co- gen of code will elevate people to the next level. So instead of having entry level people writing simple python code, you have a scrum master or agile development, a human directing other humans of what the daily sprints are like. It'll be a human directing, co- gen AI bots. But you'll still need people to make sure, is it solving the problem we want? Or maybe the co- gen can only go up to a second year professional and you still need humans to do the more complex parts. But yeah, I think the vast majority of what people are coding on today will be automated. The coding, the documentation, everything around that. And it'll be fascinating. We're talking about years. I have kids that are going to college next year and I don't know what to tell them what will be good in four years from now. So I just tell them problem solving, relating with people and problem solving.
00:51:17 Juan Sequeda
Critical thinking.
00:51:18 Tim Gasper
Things are changing fast.
00:51:20 Juan Sequeda
All right, Tim, you got the last one?
00:51:21 Tim Gasper
All right, last lightning round question. So around AI, there's a lot of proprietary advances going on, but there's also a lot of open source advances going on around AI and a lot of times it's the interplay between the two that gets super interesting. Do you see that the biggest innovation in AI right now is actually happening in Opensource?
00:51:50 Ari Kaplan
Well ChatGPT is taking all the headlines, but I do see in the non 3% one of companies that want to do generative AI on their own data sets using their own lingo. So if you have a dataset in healthcare, you don't want to use ChatGPT since that's just general, it has, I don't know, Seinfeld episodes built in. You want it based on the lingo of your industry and also oftentimes you have proprietary data that you don't want leaked out in the world or you want it trained on your own data. So a lot of that is in the Opensource world, a lot of that is proprietary... Databricks did acquire Mosaic ML for over a billion dollars, so that helped out, but I just see the whole industry... I think at first it was proprietary, but now it's getting easier and easier, faster, faster, cheaper, cheaper to make large language models. Like Mosaic, you could build it pretty much right away with almost no coding experience. Point to the dataset and you could generate it. And still we're within a year of all of this just happening. So I do think eventually Opensource is going to win out since if you have free fast easy works, that's going to be paying million dollars to a company to build it for you.
00:53:31 Tim Gasper
Interesting.
00:53:32 Juan Sequeda
Very interesting point, we'll see what happens in the next... We'll do a time check one year to see what we're doing.
00:53:38 Tim Gasper
I know. It's changing so fast that every year we got to check in and everything could be different. So it's exciting.
00:53:44 Juan Sequeda
All right, well let's start wrapping it up. Tim, takeaways inaudible.
00:53:48 Tim Gasper
All right, so takeaways.
00:53:50 Juan Sequeda
There's so many takeaways, I don't think we're even doing a service for all the stuff we discussed. Let's do our question.
00:53:54 Tim Gasper
I know. There's so much here, so I'll just make a few quick points on the takeaways, which is we started off with what is the lakehouse? And some people are like, " I've been hearing all about lakehouse for the last 10 years." And some people are like, "Lakehouse? What are you talking about?" And it's interesting to see that spread here. And Ari, you had mentioned that the lakehouse is really pretty tightly woven into this idea of the modern data stack and that there are these two paradigms, structured data warehouse and more of the data lake around unstructured or larger data sets. But the best predictions and the best data work is really when you can bring these two modes together, and the lakehouse does that. It's bringing those two different modes together in one place with a single platform, a single approach to governance where you can get the best of both those worlds and also achieve things like great performance as well. And we also talked about AI as well and how that can be an important workload for lakehouses, but also that with the generative AI movement and how much has been advancing there, that it's becoming more possible as well as more important to do things like get insights from text, from images, from unstructured data. And the best way that you're going to do that is when you have this sort of multimodal environment and that's where the lakehouse is going to really come in. And you also had mentioned that it's something that can work well for lots of different personas. So you might have more of your business personas and therefore you're just going to be using BI tools maybe directly with SQL, et cetera, et cetera. But then you've got your data engineers, your data scientists, maybe your AI developers and things like that. And this is going to be an environment that's going to work well for them as well. Where's the lakehouse kind of going? Is it complete? Is it complicated? Well, you have to know the personas, the technical folks want to get more into the technical side of it and the business folks want to keep it a little bit more high level, that's fine. And companies are going to want to standardize on one priority environment, one mandate environment. So you're kind of thinking that folks are going to pick that one center of the universe and then they're going to build around that and there's an ecosystem that's going to continue to develop and expand around that. So I thought that was a nice way to think at a high level about lakehouses. Juan, what about you? Your takeaways?
00:56:10 Juan Sequeda
Well, we'll continue. We got into the sports and the Moneyball section, right? All your experience. I mean it's fascinating you started working right since the 80s, right? Going into Caltech around this and how you just started looking, some players had bad stats but were clutch of games at key moments and somebody great stats, but bad in games. So what gives around this stuff? So that's kind of really what got you into this, seeing that disconnect and want to go ask yourselves why is that happening? So when you see the letter X in stats, that's a paradigm that you helped to start there. And so you showed up in the LA Times, right? The Dodgers reached out and it was just great that they don't even know if this is going to help, but they wanted to go learn and hey, the rest is history. So one thing is always reinvent yourself every four years. I really like that, I think we see this a lot. And talk about tools and approaches before there wasn't even that data. You have to go get that from microfilms, get the stats by hand and use the database paradox. There's a lot of history there I'm learning right now. So you wrote your first databases around this for scouting reports, even before Oracle. And eventually there's all these questions people want to ask. Now we're talking about chatting with the data people we've been wanting and having do this before. The general manager wants to ask who are the good third basement that we might put or promote or sign here? So scouts can input all this information and now we take it, what we're doing now is we have so much more physical data that we're analyzing, just approaches to swings and so forth. So AI is here all over the place on how we can not just generate things but also analyze, categorize, and predict. And one of the things that kind of wrapped up, it was a theme around a lot of stuff, was knowledge. The knowledge is critical. Your model may recommend you to do things, but it doesn't know the context. So hey, you should not be selling to X anymore, but you're like, " I have to because we have a contract, so that's not a valid recommendation." Or hey, this player is prone to injury, so tell them to throw the ball softer. It's like, " Yeah, I mean that makes sense, but at the same time it doesn't make sense." So for this, you really need to talk to people. You really need to understand the context of business and talk to people to collaborate, to paint the picture once you get those insights. And I think this wraps it all around, is vulnerability. Be able to understand, be explicit when you don't know things and just reach out and say you need help.
00:58:25 Ari Kaplan
Beautiful wrap up. You were paying attention, but you summed it all up beautifully.
00:58:31 Juan Sequeda
This was all you. So quickly to wrap up, what's your advice? Who should we invite next and what resources do you follow?
00:58:40 Ari Kaplan
Yeah. The advice again, innovate yourself, keep learning and networking. Gee, who should be the next guest? Just thinking close to home. So my prior company, I co- hosted podcast with Ben Taylor, who is now the chief strategist at Dataiku. He is one of the most creative, innovative people. Modern day Forest Gump, as he calls himself, would be good. And then recently did this show for our conference called Live from the Lake House, which was wild. We had 75,000 registrants for the online streaming. And I had some co- hosts that all have such vastly different backgrounds from sales to marketing in the AI and data space. So Pearl Ubaru, Holly Smith, Jimmy inaudible. All super energetic, but great perspectives would be great.
00:59:42 Juan Sequeda
Fascinating. I'm just looking that up. We're definitely going to ping you to help us reach out to them. Any resources that you follow? People, blogs, conferences or magazine, books or whatever?
00:59:57 Ari Kaplan
Yeah, so much. But recently like Advancing Analytics, they have a YouTube channel. They're everything, not just lakehouse, but they're vendor neutral, so you get the real deal from them. And I've learned a lot myself. When I was interviewing at Databricks, I was watching them so I know what's up. And then Kate Strachnyi with a DATAcated. You mentioned Cindi Howson. There are a lot of great folks. And then I also do follow analyst firms, especially like Gartner and Forrester who talk directly with customers and have their own methodology to quantify what's the value and what are people really doing?
01:00:45 Juan Sequeda
All right. Well with that, thank you so much. Just quick next week we have Alexa Westlake from Okta, to discuss data that does not drive results as useless. After that, Tim and I are going to be in Europe, we're going to be in Amsterdam, and then London. So more coming. All right, thank you so much. We really, really appreciate it. This has been awesome.
01:01:03 Ari Kaplan
You too, thank you. Cheers.
Special guests
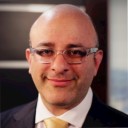