00:00:00 Tim Gasper
Hello everyone. Welcome once again to Catalog and Cocktails presented by data. world. We're coming to live from Austin, Texas, and also from CDOIQ in Cambridge, the Boston area. It's an honest no- BS, non- salesy conversation about enterprise data management with tasty beverages in hand. I'm Tim Gasper, longtime data nerd, product guy and customer guy at data. world, joined by Juan Sequeda.
00:00:29 Juan Sequeda
Hey Tim, I'm Juan Sequeda, principal scientist at data. world and as always it's a pleasure. I'm so excited that we're now getting back to our flow and season six, starting year number four of our podcast. And, I'm just so excited that we get to do a podcast with such amazing people and we get to do this live. And today, we are live coming from CDOIQ and we're with Tom Redmond, the data doc.
00:00:52 Tom Redmond
Yeah, thank you for having me. I've been looking forward to this since you invited me.
00:00:56 Juan Sequeda
Which was a while ago. We've been prepping this for six months or something like that.
00:01:01 Tom Redmond
It does seem like that.
00:01:02 Juan Sequeda
And I think it was. But we're super excited. A lot to chat about what we've been hearing in the first two days of CDOIQ.
00:01:08 Tom Redmond
Right.
00:01:09 Juan Sequeda
You have an upcoming book that I want to talk about, and talk about what's working, what's not working. But anyway, before we get there, what are we drinking? What are we toasting for? Tim, you go first.
00:01:19 Tim Gasper
I've got a Bee's Knees here, because being able to chat with you, Tom, is going to be the Bee's Knees. So, I'm very excited about that. It's got some Neptunia Hendrick's gin, some lemon, and some honey syrup. I made some honey syrup a couple days ago, so I'm trying to use that up. And so, yeah, excited.
00:01:37 Juan Sequeda
How about you Tom?
00:01:38 Tom Redmond
So, my tastes are a little more pedestrian. I have a gravity free beer. And, the thing we need most in the data space is courage. And so, I'm toasting the people who've shown the most courage for the last 18 months. In my view, the people in Ukraine.
00:02:01 Juan Sequeda
Cheers to that. I'm having a paper plane, which is, whiskey in a bourbon, and aperol, and very refreshing and sweet for today. Oh, cheers to that, Tom.
00:02:15 Tim Gasper
Cheers.
00:02:16 Juan Sequeda
All right. We got really good cocktails today. So, warmup question, if you could be a doctor or a therapist, but not the data doctor, what kind of doctor would you be?
00:02:29 Tom Redmond
So, I mean it's really interesting that you pose that question that way because I actually think mostly I am a therapist. Right? Maybe it would be change management, or organizational change, or organizational psychology, something like that. And so, mostly, I work on data quality. And when you really get down to it, there's four or five concepts that you have to understand. Now, you have to understand those and figure out how they're going to work in your organization. But, that's the psychology, that's the change management. And so, I think, if I wasn't the data doc. I don't know, the organizational change doc doesn't have the same ring, but that might be where I'd have my degree.
00:03:16 Tim Gasper
Nice. Nice.
00:03:18 Juan Sequeda
Tim, what would you be, the blank doc?
00:03:22 Tim Gasper
I've always been fascinated with the brain. Right? So, I'd be a brain doctor, whether that's a neurosurgeon, or a cognitive scientist, something like that. So, I'd be the brain doc.
00:03:34 Juan Sequeda
And I think I'm taking inspiration from my wife who works a lot in human behavior. She's a psychologist and in special education working with children with autism. So, I like the whole part of just understanding human behavior. So I think it's also the type of therapist, and we're talking before that, maybe it's a bartender too, right? To go talk to people, understand the problems.
00:03:54 Tom Redmond
So, I mean, right, having that set of skills is not about mixing the drinks, but talking to people and engaging them is really, really important.
00:04:03 Juan Sequeda
Yeah. All right. So I think, we got different career options here if you want to go change. All right, well let's kick this off. All right, we're coming from CDOIQ. Tom, you have an incredible career going, seeing so many things out decades and decades. Honest, no- BS, what's working in the data world? What's not working in the data world?
00:04:21 Tom Redmond
So it's a really good question. And I think that there's two parts to this and it's a little bit paradoxical, because if you look over the last, I don't know, 20, 25 years at the success stories, broadly, I see the world in the data science, the analytics, and the data quality space. Right? So, I think both those broadly. But there's enormous successes in both those spaces. So, we designed the global telecommunications network and the thing worked, right? We have manufacturing facilities that produce incredible amounts of stuff at lower and lower cost, and analytics and data sciences has played a role in that. So today, AI, I mean, there's some great stories. One of my favorite is something that's going on at Morgan Stanley called Next Best Action, right? And similarly, in data quality. If you attack data quality properly, right, and focus on what are the root causes of the problem, and how are we going to build the organizational capabilities to get at those and make them go away permanently? It's a miracle. So much cost goes out of the company today, and a lot of companies... Maybe a third of people's time over the entire organization is spent dealing with mundane data issues. Right? And so, you can make an incredible amount of that go away. And when the people do the work to make that happen, it is the most empowering thing ever, right? And this stuff, I mean, the methods are written up, the approaches are written up, the case studies are written up. Some of my clients include Chevron, and AT& T, and Morningstar, and so forth. So, there's not as many data quality case studies as there are in the data science realm. But this stuff works, and we know it works because it has been proven in tough environments. But, beyond the points of light, the situation is really, really a lot different, and progress has been expensive, slow, it has been painful, and uncertain. And if you just take a typical organization today, you find, data quality is essentially unmanaged and pretty darn bad, right? The failure rate of data science projects is embarrassingly high. So, there's various statistics out there, and it's exactly what counts, and so forth. And, where their failures actually make it into the statistics. I don't know. It is north of 90%, almost. Certainly, in most organizations. A little bit more subtly, technical debt is out of control. And all this, costs are high. We have seen some great technologies in the last, I don't know, dozen, 15 years, right? Generally speaking from an economic point of view, we expect technology to drive productivity. But productivity is flat, right? And the effects of that across the economy in terms of the inability to grow. And so, they're really stifling, right? Inside the company costs are high. We talked about that a little bit. But, in the global economy, the productivity is flat. And I really want people to wrap their heads around that. There have been some great technologies, blockchain, data lakes, data warehouses, that productivity should be soaring, and it is not. Okay? And so, overall, we have this paradox, we have this paradox of what is demonstrably possible, right? And then, we have the, I don't know, maybe mediocre performance that most are experiencing. And, the impact on all of us is pretty darn bad.
00:08:50 Juan Sequeda
So, we know the art of the possible because it's not just an art, it has happened. So, we have these concrete examples, but we're also living through a world where we're just seeing all this pain, and where is that productivity? We're not seeing many more of those success cases. So, it's working, but at the same time, it's not working. So I would argue that at the end it's not working. And, you brought up, we're accepting mediocre results. The status quo is like, " Yeah, it takes so much time, that's just the way it is. And, we have to go deal with all this stuff."
00:09:31 Tom Redmond
So, look, I mean, I do want to have a viewpoint here. Not all organizations are this way, but I think that there is a point of view that says, " Okay, we're going to take a business process, we're going to open it up, we're going to insert a new technology, and then we're going to deal with the wetware." Right? The wetware are the human beings. And the fact of the matter is it's just way more complex than that. If you look at the technological successes, the technological successes were accompanied by changes in management, changes in the way the organization was set up, changes in responsibility, and then sorting out the accommodations between people and the technologies. I mean, a simple example is, a great technology was electrification. Right? Well, when that came in, some people had all these bear wires, right? And so, it was dangerous. Touch a bear wire and people could lose their life. And so, we had to figure out how people were going to work with electrification, and use it. How we were going to change our factories and so forth, right? And so, in my view, the things around the technologies that we're seeing now, and I'm going to put AI, and especially gen AI in this bucket, is there has been an incredible focus on the technology, and not the things that go with it. Right? And in the case of analytics, and a lot of these technologies, the things that go with it are the data, and the data, and the people, and the organization. Right? And so, we have this, think of this as a three or four legs or whatever it is, right? But one leg is yay long. And then, the other two are like this, right? If you talk to people when you get down, " What really made you succeed?" It was, " Oh, we did this organizationally." Or, " I connected with this person." Or, " We got together as a team." Right? And those things, for those that are not succeeding, it is some... So, we've talked about Tolstoy, right? It's like, all good families are the same, all dysfunctional ones are dysfunctional and they're the same way. But, each organization has some combination of not dealing with the data, right? Not bringing people front and center, right? Blaming people for not knowing what they're supposed to do when nobody told them, right? So, making the organizational changes. And so, I don't think that we're going to see these growths in productivity, or these big success stories, or the promise of technologies and AI until these other things catch up.
00:12:45 Tim Gasper
I think that that is a very strong statement. And, I'm not sure that I disagree with it. But, I also want to actually backtrack a little bit, because I think that you made a very strong statement about five minutes ago around, " We expect technology to drive productivity, but productivity is flat." And I found that to be a very interesting statement, because even with AI, I think there's a lot of folks that are very excited about the productivity impact about AI. I know Juan and I talk a lot about that as well. That, " Oh my gosh, you can write things much faster and it can help explain the data and you didn't even have to teach it anything. You can just throw it on structured information and all of a sudden it's telling you insightful things, and answering your questions, and things like that." Right? So it certainly feels like there's a productivity improvement that's happening, but it seems like you're saying, something is weighing it down, it's related to governance, and to quality, and to people, and to organization design. Is that a correct way of thinking of it? And, what really goes into you saying the productivity is flat versus maybe just increasing, but not as much as we wish?
00:13:59 Tom Redmond
Well, let me answer the first question... The last question first. So, I read a magazine called The Economist, and they follow such things, and they've had a couple of good features on this particular topic in the last year, 18 months, or whatever it is. So, this is not Tom Redmond opining that productivity is flat, this is U. S. government statistics or whatever it is that The Economist is sourcing things on. And so, yes, I mean, that is a strong statement. People want to challenge that, well go, look, I mean, there's improvements that don't make it into the productivity stats. And maybe, there's things there. But then, your first question was, " Hey Tom, I think you said that there's a whole bunch of other stuff that has to happen for this technology, or any technology for that matter, to really flower and it hasn't happened." Okay? And that is 100% what I'm saying, right? Now, further, by the way, though, Tim, take new technology out of it. I mean, just take day in and day out work, and day in and day out work, we are still left with the fact that across an organization, a third, 40%, a half of people's time is spent dealing with mundane data issues. I mean, it is a tremendous productivity drag, having nothing to do with any new technology.
00:15:45 Juan Sequeda
One of the things, we focus so much on productivity, and I want to tie this to one of the takeaways I've been having from the conference right now is, when it comes to data and what CDO should be focusing on is value. And I think that last year, the theme was value. " How are we driving business value, business value?" And right now, the theme that I'm hearing throughout yesterday and today, it's profit center. " How are we making sure that the data that the business value they're driving is profit?" Which is different from productivity. I would argue it's different because productivity, efficiency, that's like, " Oh, well, you spent less time here, therefore your time has worked so much. So we saved." Whatever. And I was like, " That's the fluffy type of savings I would argue."
00:16:36 Tom Redmond
So now, wait a minute. Cost reduction is not fluffy. Right? Profit is still revenue minus cost. And so, if you take cost out, it is impacting profit. And, productivity is what did you get per unit of labor? Right? And so, you reduce the unit of labor. I mean, there's nothing fluffy about that.
00:16:58 Juan Sequeda
Yeah, very fair point, it's very factual, you just said. I agree. Let me go rephrase. I think, there's all these claims that people are making about how things can be, we save so much time and stuff. And I feel that these claims about how we're saving time when it comes to data... I don't know, I see this a lot of the vendors going on and that's the fluffiness that I do. But, when we zoom out, I think, what we really need to be able to go make sure that we're aligning is to what are the top three pain points that the companies are doing? And it's not like, " Oh, we're wasting so much time cleaning the data." Whatever, stuff like that.
00:17:38 Tom Redmond
Well, so let's build on that, right? I mean, at a high level, look at profit, and revenue, and revenue and cost, right? Well, so a dollar in either one of them, right, has the same net effect. But organizations think differently about those dollars, right? So, I spent the early part of my career in telephony. And, there was this concept of a cost, how much it costs to provide a minute of phone service, kind of thing. I mean, and billions of minutes were out there, right? And so, if you could drive that down by just a fraction, it was an incredible, right, an incredible multiplier. And so, cost went down. And in telecommunications at the time I worked there, those dollars were valued, right? Now, my first client when I hung up my shingle was an investment bank. Right? And frankly, they spent water like a drunken sailor in port kind of thing, and they didn't care about cost. And, when you really looked at, I mean, they got their bonuses on making deals, right? They cared about revenue. And so, to your point, the data efforts ought to be aligned to what the business cares about, right? And aligned to hard numbers that the business cares about. The most incredible thing in the statement you just made is, why in the world in 2022, are we even having a discussion about the notion that you got to add value? Right? Think about how ridiculous that is. I mean, think about how ridiculous that is for any function, any operation, any process in any company, everywhere. Right? No. Say, " Oh yeah, we just exist so that you can play games with the data." It is absurd, right? Now, I do agree with you. So I see the shift more to it is a political thing. And we want dollars on the revenue side, as opposed to the cost side. The harsh reality there though is you can't sell bad data. You can't use it to make a model. You can't use it to make better decisions or anything like that. So data quality, I mean, it's easy to see the cost reduction, but it is also on the critical path to frankly anything on the revenue side using data. Okay? And so, it's almost like the gift that keeps giving in that respect. Yes, we're going to get the immediate cost reduction, and we're going to set ourselves up so that we can do more clever and fun things with the data.
00:20:49 Tim Gasper
So, Tom, what needs to change? You talked a little bit about some wetware problems, and some of our data management problems, and we haven't been turning this latest data revolution into productivity. So what needs to change?
00:21:05 Tom Redmond
So look, I'm going to narrow my focus right now for a minute on data quality. Okay? And, I want you to imagine, Tim, that you have a bucket. And in that bucket is every data quality issue that the company has today. Okay? Now you'll probably say, " Well Tom, that's not a bucket. That's a big oil drum." Right? Or maybe it's a tanker, or maybe it's a super tanker, or whatever it is. Okay. And now, do the thought experiment that says all those went away. All those went away. And, what would you have in a year? Right? Well, the bottom line is, you have the same problem you have now today, right? Because organizations, the things that are causing these problems to be in your way today, the root causes of those things continue to exist. Right? And so, it's like, " Okay, well, we cleaned up all the bad data. Not to worry, the company will make more." Right? We have technical debt, we've eliminated it, " Not to worry, we'll make more." Right? We don't understand lineage. " Well, okay, we've solved the problem. Not to worry, we'll make more." All right?
00:22:23 Tim Gasper
It's a bandaid every time.
00:22:24 Tom Redmond
It is a bandaid every time. And organizations are focused on getting through the day. Right? And so, if you talk to somebody in sales, and they're trying to follow- up on the data they get from their marketing counterparts and they have a quota, they've got to make so many calls, and the data's not right, well, you can't blame them for cleaning up that data, so they can meet their quota. But, on the other hand, they're just laying the foundation for that problem never going away.
00:22:57 Tim Gasper
And so, data issue whack- a- mole is not the answer.
00:23:03 Tom Redmond
So, it's not the complete answer. I mean, you must get through the day. But the secret is to figure out how we're going to stop making so many errors, right, stop creating so many of these problems. And, from a quality perspective, here's what works is, you Tim, are a customer of data coming in for marketing. I'm in marketing, I'm the creator of the stuff you need, right? Rather than, you being in the bad habit of just accepting my junk, and probably swearing at me, right, under your breath, is you need to actually give me a call and say, " Tom, I'm having some problems with the lead state. Can we sit down? Can we work out what's going on?" Right? And almost always, when you approach me in a professional manner, I said, almost always, not always, but almost always, there is a healthy interaction. All right? And a high fraction of the time, you'll get a response like, " Tim, I never knew you needed that stuff. I can do better." Okay? And so, the three hours a day you're spending, well we may not be able to reduce that to zero. But, that three hours a day practically, and this is what all those success stories I've talked about is, that three hours a day goes to an hour, and a half an hour is spent helping me get you better data. And a half an hour is spent cleaning up things that we haven't sorted out yet. Now probably then we're also going to say, " Hey Tim, the person you're sending your data to..." You're creating his or their data, you got to be conscious of that too. All right? And so, this is why this is an organizational issue, or a cultural issue, or whatever you want to talk about. But there are these fundamental concepts called a data customer and a data creator. Okay? And it is observably true that everybody in every organization has those roles to do. But they are not doing those roles. They're not conscious of those roles. They're not trained in those roles. They're not supported in those roles. Right? And, everybody, 95% of the people in organizations are doing this data management untrained, without support. And, it's really no surprise that two thirds, or a third of the time, I'm sorry I misspoke, a third of the time is spent dealing with these issues. All right? Now, I mean, let me just say one more thing. So, everybody who has taken this up, I mean, this is really empowering stuff. But, at the organizational level it is a change, right? And, the reason the organizational change doc is sorting out how do you get these things into an organization at scale and painlessly.
00:26:10 Juan Sequeda
So, Tim and I hear back channeling and we're like, so is this the idea of bad data incorrect? It's really data that because people are creating this data, it's the lack of collaboration, lack of understanding. So, it's not bad data, it's just, well, misunderstood data.
00:26:30 Tom Redmond
Well, so I mean, look, the data literally is bad. So, if I put in and my address is 10 Main Street, Anytown U. S. A., and you're trying to use it to send me a package, well, it's bad data, because that's not where I live. Okay? But it is getting together. Right? And so, I mean, you may look at this and go, "Well, there is no Anytown, U. S. A. I can't send a package there." And so now, you do the work to find out where I really live, right? Now, you are correct though, in the extent that, I mean, it's not like I'm creating this bad data to screw you up or anything like that. We don't have an understanding of what you need.
00:27:12 Juan Sequeda
Well, so I think we can consider almost a spectrum of the types of data issues. One where we would argue, " Yes, this is bad, and it's wrong, and so forth." All the way to another side of the spectrum where it's misunderstood. We're like, " You agree, you say it's this. I say it's no." Depending on the context, we're both right, we're both wrong.
00:27:32 Tom Redmond
Yeah. So I mean, you're absolutely right about that. I think of the spectrum of data issues in terms of how easy they are to solve. Okay? And so, I want to take 100 in a company, and then we're not talking about the specific errors, we're talking about the root causes of the errors. And, I want to take 100 of those and 80 of them are pretty easy when you get people together. Okay? And then they get increasingly complex, right? And there's probably two of them that are deep ontological. What do we mean by customer? Right? What do we mean by address? Right? That are too hard for most people to solve, right? And those can be pretty darn costly. So they can impact a lot of things. But by sheer number, by sheer number, 80% are pretty darn easy. And, organizations should start on those. People should start on those. Sometimes I feel like, we're drawn like moss to a flame on, " Let's tackle the really hard ones." Right? " Let's get a common definition of customer across this 300,000 person company." Kind of thing. So, what you're saying is absolutely correct.
00:28:57 Juan Sequeda
Okay.
00:28:57 Tom Redmond
I want to add one other thing, and that is, data quality, people think about it as it's accuracy. I mean, they have a narrow conception of it. But, it's actually very broad. And it depends on context, what you're trying to do with it. And let me illustrate this way. I mean, have any of you guys had a teenager yet?
00:29:21 Juan Sequeda
Not yet.
00:29:22 Tim Gasper
A 14- year- old.
00:29:25 Tom Redmond
So, Tim, you may relate to this story. So, you're driving home one day, and you get a call, and you don't recognize who it is, but you recognize the number is from your kid's school, right?So you pick up the call and say, " Well, I'm sorry, Tim, but your kid was caught fighting." And, they may even been trying to break it up. We don't know about that. "But, we have a zero tolerance policy." And so, seven- day suspension, five- day suspension, well week- suspension, whatever it is. Well, and you get home, and what do you do? You call the kid down, be like, " Well, how was school today?" Right? And the kid says to you, " Dad, it was great. I got A minus on my Spanish test." Now, I made you smile, you're not questioning that the kid got A minus on the Spanish test, but that's not what you were asking. All right? And, they know that's not what you were asking. All right? And so, there is always this other dimension, which is a lot slippier called relevance. Is this relevant to the task at hand? Is it relevant to the decision, kind of thing? Or, even simply the operation. And quality, people think about it too narrowly. If you're trying to train a model, there are certain times when bias is your biggest worry. There is bias in the training set and you do not want it to be reflected in the model. Well, that's a quality concern too. There's times to properly train a model, you just need lots of data, kind of thing. And say, " Okay. Well, we only did this 30, 000 times. It's just not enough."
00:31:27 Tim Gasper
Is relevance in the 80 that are easier to solve or is it in the 20 that's harder to solve, or it depends?
00:31:36 Tom Redmond
Yeah, I mean it depends, right?
00:31:37 Tim Gasper
Okay.
00:31:37 Tom Redmond
Well, so the ones that are easier, usually as Juan was saying, is it right or is it wrong? Is the address right? Is the know your customer code right on things?
00:31:50 Tim Gasper
Yeah. Is it an integer in the integer field? Et cetera, et cetera. Right?
00:31:54 Tom Redmond
Well it's in a correct integer.
00:31:56 Tim Gasper
Yeah.
00:31:56 Tom Redmond
Yeah, right.
00:31:58 Juan Sequeda
So, that's a good point though. It's a good test. If it's right or wrong, so if I can have a right or wrong and we have an agreement on that, then that falls into the easier part. The moment where people started doubting, they're like, " Okay, let's put this into the bucket that needs to go focus on relevancy and context." And, for that one, it's where we need to start talking to other humans, people.
00:32:24 Tom Redmond
Exactly right. Look, let's face it, most people in organizations are not data scientists.
00:32:30 Juan Sequeda
No.
00:32:30 Tom Redmond
Most people do things that I like to call run the store. Right? So they try to get people interested in your stuff, they try to sell your stuff, they try to deliver your stuff, they count the money associated with that delivery, they make hiring decisions. And so, in this case, almost all the time, the context is pretty darn clear. We need contact information, such that... We need to know somebody's title, such that, when we talk to them, we don't say, " Well, hi Ms. Sequeda. How are you doing?" Kind of thing. In a lot of operational stuff, context isn't that big a deal. But, as you begin to work up the value chain of more complicated decisions, and so forth, then other dimensions, like the relevancy, or do we have enough data, right, become more and more important?
00:33:32 Juan Sequeda
So, let's dive into the topic of people. And, we are a non- salesy podcast. But I am all for saling of books and knowledge. So, tell us more about what your upcoming book is about.
00:33:47 Tom Redmond
Yeah. So my book, which is already out in the UK and most of the world and is out next week in the United States, is called People in Data. And it stems from two very simple observations. The first observation is that, the vast majority of data management is being done by people without data in their titles, by the marketing person, the salesperson, the operation person, the finance person who are simply doing this work of correcting the data, right? Looking for, so maybe, confirmatory stuff, but they are doing management task. They're spending a significant fraction of their day doing it. And, as we noted before, I mean, they're untrained, they're unsupported, they're basically doing these tasks on their own. And the second observation is, is there is nothing significant that we can do in the data space without regular people. So, a data scientist cannot properly understand the business problem, he, she, or they are trying to work on unless they understand what's going on with the regular people, at the coal face or in the decision making realm or whatever it is. And by and large, we have excluded regular people. And some people even blame them. Right? So then, the problem is, " Well, these people just don't get it." Except, they've never been trained, they've never been informed, or anything.
00:35:23 Juan Sequeda
"It's their fault."
00:35:24 Tom Redmond
"It's their fault." Right? They didn't do it. Senior management don't have a system where they were asked to do it, right? But anyway, so people and data, right, starts out, and it simply says that we have to bring regular people in. Right? And we have to bring them in a meaningful way. We have to provide training and support for them. And, frankly, it argues that since that's where the data management is being done, most data teams, a significant fraction of their time ought to be spent supporting these people.
00:36:05 Juan Sequeda
So is this just data literacy? Or is this more than that we're talking-
00:36:08 Tom Redmond
So, I hear this term literacy. And I want to first of all make the comment that I don't like that term at all.
00:36:16 Juan Sequeda
...Yes. Me too. I don't like it. I'll give you my rant in a second, but go.
00:36:20 Tom Redmond
Well, so look, I mean, where I grew up, where you called somebody illiterate, you were calling them stupid.
00:36:24 Juan Sequeda
This is exactly my point. And shout out to Malcolm inaudible, we had that podcast where we shared this sentiment. Data literacy is-
00:36:34 Tom Redmond
The term's got to go.
00:36:35 Juan Sequeda
...Amen.
00:36:36 Tom Redmond
Right.
00:36:36 Juan Sequeda
Yes, because you're implying that you are illiterate. And if you're implying that they're stupid. I'm like, " No, it's disrespectful."
00:36:44 Tom Redmond
It is disrespectful. Right? There is a difference between being uninformed and untrained, and illiterate. So, but still the answer is no. The first thing that this starts with is you have to think through what it is you want regular people to do. All right? And so, the training is not on literacy. No, literacy, you go to college to be literate, and become a great thinker, right? The way I'm thinking about this is, " We want you to become a better data customer and data creator. Here's what you need to do your job." Right? " We want you to learn to make better decisions." Right? " Here's some training on decision making." Right? " Here's some support we can give you. Here's some metrics that will help you evaluate whether you're a good decision maker or not. We want you to follow privacy and security guidelines. Here's what that means. Here's how you do it." Right? " We want you to contribute to big data problems, larger analysis things. Here's what you need to do that." Right? " We want you to make improvements to your business process, independent of anything else going on. Here's what you need to do that." Right? And so, this is a big difference. This isn't literacy for literacy stake. It starts with, " Here's five things..." And no organization could do all of them at once. Right? I like starting with quality, I mean, you know where I'm coming from on that. "But these are the five things we want you to do. And now, we're setting up our organization to help you do those things. And one of the helps we're giving is we're teaching you how to do those things." Okay? " We're teaching you what it means to be a good data customer, what it means to be a good data creator. Here's the steps you go through to do that work." Right? And so, ignoring the literacy thing, I don't think of that as literacy at all. I think of that as the same as if you're a barista, " Here's how you make a cup of coffee." Right? When you're dealing with data, " Here's what we want you to do and here's how to do it." And so, I think, that is a subtle shift. A lot of literacy people are going to build on what I say here and say, " It's all about literacy." And, it's directionally correct, but not quite. Is that fair?
00:39:25 Juan Sequeda
So, actually, I'm going to take a comment here. We have, " What title the workshop instead of data literacy?"
00:39:35 Tom Redmond
What are you talking about?
00:39:35 Juan Sequeda
Well, I mean, we replace data literacy with what?
00:39:38 Tim Gasper
Instead of saying, " Come to my data literacy training, it's X."
00:39:42 Tom Redmond
Yeah. Well, the first class is called, " Come to data quality training. We're going to teach you how to become a good data customer and good data creator." Right? " Come to quality improvement training. We're going to teach you how to use small amounts of data to improve your process. Come to decision making class. We're going to teach you how to evaluate whether you're a good decision maker or not. And how to learn to become a better one." Right?
00:40:06 Juan Sequeda
So, it's really not about the data first. It's really about the decisions you're trying to make... The process you're trying to go improve, and then how data's going to help for that.
00:40:16 Tom Redmond
Yeah. I mean, look, we started this with business results. Right? So, this is driven by business results.
00:40:21 Juan Sequeda
Exactly.
00:40:22 Tom Redmond
Right. Right.
00:40:23 Juan Sequeda
So this is a great point that I think, one could argue that data literacy is shedding the light or putting the focus on what we should not be doing. It shouldn't be the first thing or how we frame it. If our goal is to try business value, and increase profit, and so forth, the workshops and the training should be about the business process going first, and then how data supports that, versus... I think we're doing it the other way around. Like, " Here's what you need to go do with data." The data tech conversation comes first. " Hey, here's how you use Power BI to go do these things." You're training on technology instead of the business problem.
00:41:05 Tom Redmond
So look, I've just been working with a client in this Gulf Bank. We put this in the public domain. There's a great article in Harvard Business Review a couple of months ago. Right? But, the training there, we first set up in inside that company a network of people called ambassadors, or the point people for all things data, down to the team level. The company has about 2000 people in it, and 140 ambassadors got named. All right? And so, it's a part- time role. Right? So the point people. But then, the promise to those people was you're going to get world- class training. Right? And then, once that was done, that took about six months, everybody in the bank got training on, we called it, Data 101, it's this customer and creator stuff, kind of thing. But there were 20 versions of it. " Juan, you work in a branch, well, here's the stuff for the branch. Tim, you work in risk management, right, here it is in risk management." And, the underlying principles were the same, but the examples were tailored to the work that people were doing.
00:42:19 Tim Gasper
Who was developing that training? Was that the governance office? Or was there a specialized group focused around data, and quality training, and things like that?
00:42:30 Tom Redmond
So look, yeah, I'm really glad you asked that question. So, the chief data office put that training together, I helped them put that training together. We went through how it was going to be delivered. By the way, when nothing was delivered online, everything was face- to- face, right? And it was delivered face- to- face, because this is the importance that we place on it, kind of thing. But a really, really key thing is the chief data office worked with HR. And HR is where the training center was, and knew how to deliver courses, and could do things at scale. And it's a plus, they had a bigger budget, than the data office. So the training was a joint effort, involving me, I mean, as there advisor. And then, the data office, and then HR.
00:43:32 Juan Sequeda
Okay.
00:43:32 Tom Redmond
I mean, now by the way, they call it literacy training. They decided not to go through this little tantrum you had, which I agree with, kind of thing. But again, I mean, it was tethered to exactly what we want you to do. Right? It was very, very specifically aimed at empowering people to make it so you don't have to come in every day and put up with this junk. Right?
00:44:01 Juan Sequeda
So, here's another interesting question we're getting here from the audience is, I think you missed one root cause of data quality, the SQL query writer.
00:44:13 Tom Redmond
Yeah.
00:44:14 Juan Sequeda
What are your thoughts about this?
00:44:17 Tom Redmond
So that that's beyond my level of expertise. I can't comment on that.
00:44:23 Juan Sequeda
Well, so let me chime in. I think, this is more about now getting into more of the semantics, the understanding is, I got a question, I got the data to be able to do. Is the person who's writing the question, writing the query is actually truly understanding that.
00:44:37 Tom Redmond
Oh, yeah.
00:44:37 Juan Sequeda
And I think, this goes back to the conversation we were having before we started the podcast, is that, we have this big gap between the people who understand the business, and where the data, and the people who actually manage the data. And then, we're talking over each other and we don't even know if we understood this correctly.
00:44:54 Tom Redmond
So, look, I mean, I'm really glad you raised that question. And, I mean, our initial focus, and almost always I advise customers, the analytics, the AI, the data science that comes second. Right? The first thing is getting the store working. Right? This is the place where you make money. This is the place where you acquire customers. This is where you incur costs. I mean, think about a typical organization. I mean, data science, maybe 1% of the organization. Right? Why are you focusing there as opposed to the other 98% or whatever it is. Now, by the way, the answer is that, is because that's where the new revenue's going to come. But you still need that data out of the store to be of high quality before you're really going to be able to do good data science.
00:45:49 Juan Sequeda
And I think another thing that... Part of my rant when I say, I dislike the data literacy, is that, what we do need is business literacy training for the data folks or data teams, because I would argue, in that case, that they are illiterate when it comes to how the business works.
00:46:09 Tom Redmond
Sometimes I even think that a lot of data scientists are entitled. Right? I mean, it's almost-
00:46:15 Juan Sequeda
I'm not the one saying it, but yes.
00:46:17 Tom Redmond
...I meet some of them-
00:46:18 Juan Sequeda
No, I agree. I agree with you.
00:46:20 Tom Redmond
... But a lot ofdata scientists don't realize how hard it is to come into work every day and run an operation, right? Run a branch. Right? So, people may not show up, the electricity may fail, the computers may not boot up. So, we got to do this regulatory reporting and all kinds of things can go on. The work of running the store is really, really hard. Right? And I feel that too many data scientists are not respectful of that. And I go, " Well Tim, you're doing this hard stuff. I got this clever model that'll make your life a whole lot easier." And you're smiling and you're thinking, and there's two words you're thinking, and hopefully they are, " No thanks." Kind of thing. But, just looking at the businesses as a whole, as best as I understand them, the most efficient place to start, the easiest place to start, the place that makes it so that you can do the clever stuff later on is in the stuff I call running the store.
00:47:37 Juan Sequeda
I like this. Running the store.
00:47:39 Tom Redmond
Running the store. Right? The stuff we do to make money. Okay. It's not a deep concept.
00:47:48 Juan Sequeda
No. All right. So, with that, let's start wrapping up, because time flies. We can keep-
00:47:54 Tom Redmond
It does. It does fly. I mean, can I just say, look, People in Data is out next week. And I have done my level best to diagnose these problems. Half of this book is aimed at diagnosing the problems. And I think that a lot of problems, when they're properly diagnosed, the answers are not that hard. And one thing you cannot unhear if you're on this podcast is that most data management work is being done by regular people without training, right, and support. All right? And so, if you're in data that cannot be okay with you. Right? And so, it's a lot of what people have done it it's like, "Well, okay, now what do you do?" Kind of thing.
00:48:38 Juan Sequeda
... I likethat. Most data management is done by regular people who don't have the training.
00:48:43 Tom Redmond
They have no training and no support.
00:48:45 Juan Sequeda
And support. All right? So, let's do the AI minute. You got one minute to rant, talk, whatever you want to say about AI. Ready, set, go.
00:48:56 Tom Redmond
So look, I think that I'm really excited about AI. But, I think that those who don't recognize that there are data, and people, and organizational issues that need to be worked on, and these are hard issues, they demand equal attention as the technology. I think that those who don't spend that energy are going to be sorely disappointed. Right? And any promoters of AI who are not transparent about that are on my rant list.
00:49:32 Juan Sequeda
All right.
00:49:34 Tim Gasper
Fair criticism.
00:49:35 Tom Redmond
Yeah.
00:49:36 Juan Sequeda
All right, Tim, we got some lightning round questions.
00:49:38 Tim Gasper
We do. Yeah. And I think, Juan, why don't we start with you?
00:49:38 Juan Sequeda
All right. Let's kick us off right here. So, our lightning round questions are presented by data. world. So, question number one, the assembly line electrification, personal computing, internet, these technologies have really made a gigantic productivity impact. Will AI break our current productivity plateau that you've been talking about?
00:50:06 Tom Redmond
So I don't know the answer to that. I mean, I think that AI, data quality, and people are entwined, right? And you're going to have to make progress on all three of those along the lines that we talked about. And, if enough companies do, then certainly, they have the potential to break the productivity trap to accelerate productivity. But if you fail on any of them, I don't think so. Right? AI depends on high quality data and high quality data depends on people.
00:50:40 Juan Sequeda
I like that. AI depends on high quality data, and that depends on people, right?
00:50:46 Tom Redmond
Okay?
00:50:47 Juan Sequeda
You go, Tim.
00:50:48 Tim Gasper
All right. Can the majority of the data quality problems be addressed by training and supporting the regular people in the company?
00:50:57 Tom Redmond
So far the evidence is yes. And it's not just that they can be addressed, but it is a big winner. And nothing empowers regular people than not having to come in and deal with other people's junk every day.
00:51:14 Juan Sequeda
I love that.
00:51:14 Tim Gasper
Nice.
00:51:15 Tom Redmond
By the way, I want to say one other thing about this. I've been searching for some time for what's the right analogy for people who've completed data quality improvement project. And the best one I come up with is when a five, six, or seven year old learns to ride a bike, right? And they can't ride the bike, they can't ride the bike, they can't ride the bike, and then all of a sudden they can. And they are the coolest ever. And they're walking around just, " Look dad. One hand." Right? And then, two days later, no hands, and stuff like that. It is freedom, right? And so, you're smiling, Tim, so you know how insufferable people, kids are when they've done this for a while. And, it's been the coolest, most unanticipated part of my job, is this connection between data quality improvement and human empowerment.
00:52:09 Tim Gasper
There's an unlock moment that happens that is pretty revelatory.
00:52:14 Tom Redmond
It really is, right? So, I mean, look, when I graduated, I was as techie as anybody. And now, here, at this point, late in my career, Tom Redmond, human empowerment. I'm pretty darn psyched about that.
00:52:32 Tim Gasper
Nice.
00:52:32 Juan Sequeda
All right, next question. So, instead of data literacy, how about we call it data enablement?
00:52:38 Tom Redmond
Okay. Data enablement. So, I mean, data enablement, a lot of people will interpret that is we need to make the data available for everybody. And so, it could be confused, but I mean like where you're going with the enablement, right? I mean, I also think it's more just the mundane, " Well, here's what you need to do your job." Kind of thing.
00:53:02 Juan Sequeda
Yeah, it's, " Here's how you get your job done."
00:53:05 Tim Gasper
So, it's job training, essentially.
00:53:11 Juan Sequeda
It's job training.
00:53:11 Tom Redmond
I mean, just think about it, if you went in a Starbucks and you ordered a coffee and the barista said, " Well, I've been working here for three years and I still, nobody's ever given me any training on how to make a cup of coffee. So this is going to be an adventure. And you get what you get." Right? Kind of thing. I mean, it's ridiculous when you see it in that light. Okay. So, I like enablement. Maybe a better phrase just, " Here's what you need to do your job."
00:53:41 Juan Sequeda
I love that. All right.
00:53:43 Tim Gasper
All right. Fourth and final lightning round question. This one has a little bit of an open- endedness to it, but I'm very curious to the answer. So we're going to cheat a little bit. So you emphasized data quality quite a bit today. Would data privacy and security be the next thing or two? Or, what's the second thing? If you had to pick your second thing that was coming after data quality, what would that thing be?
00:54:06 Tom Redmond
I think the thing I'd pick is process improvement using small amounts of data. Okay? And the reason I think that is I think there's progression from everybody just getting the quality issue, to everybody being able to use data to improve their process, their team's performance or whatever, to be assisting in big data things. And I think the way you build organizational muscle, individual capability is in a sequence like that.
00:54:39 Juan Sequeda
I really love this answer, and I think it's something that I've been thinking a lot is, we need to... I always talk about catalogs are more than data. It's about data and knowledge. And part of that knowledge is bringing together is how the business operates, the business processes, and the decisions that are being done, and the people who are part of that stuff. And then, at the end, you talk about data lineage, but I want to talk about business lineage, and how things work, so I can improve those processes, or learn from the processes that are doing great and why they're doing great. So, I love that. All right, Tim, take away times. Take us away with your takeaways.
00:55:13 Tim Gasper
All right. Well, we started off with the question of what's not working in the data world. And, you actually started off on a very positive note, which we appreciate. And you said, " Hey, if you look at the last 20 plus years of success stories, there is a lot of positive to see." You said that if you look at the telecommunication space, all the data- driven ability to make these networks work at scale. And manufacturing, what we've accomplished there. There's also these really compelling AI stories. You mentioned Morgan Stanley for example, with Next Best Action. And, these data quality success stories at places like Chevron, et cetera. Although, the data quality success stories aren't quite as plentiful as you'd might hope. But you did mention that they're out there. However, given that success progress has been expensive, a lot of money has been flowing out the door. It's been slow, it's been painful, and it's been uncertain. We haven't notably affected the failure rate of data science and data projects in general, which continues to be in the 80 to 90% range, depending on what studies you look like and look at. And that hasn't notably changed, despite the fact we have all this exciting new technology, right? We have modern data warehouses, we have streaming technology, we have blockchain, we have AI advances. So there's this paradox, as you noted, of all this technological advancement and yet very little, if any, productivity improvement. And, I even pushed on that productivity a little. And you noted, if you look at the Economist and these macroeconomic studies, you can see that even though maybe on a day- to- day basis we feel like we have these magic moments. On the whole, in the aggregate, productivity has not notably changed. And that's a problem. And it really ties back to the fact that, as you mentioned, multiple times a day, people, and process, and data quality all need to come together and need to work well if you want to then be able to take advantage of a lot of these more advanced technologies. And I know we'll have a few more takeaways on that in a moment. You mentioned that it is absurd that today people have to talk about business value. It's the 2020s for God's sake. And somehow, we have to remind people of this. And, either it's a problem that we have to remind them of it, or it's a problem that we're here in the first place. And, when you really zoomed into what needs to change, you really, especially zoomed into data quality. I think that was one of the biggest things that you emphasized today. And, I think you had a really good analogy or example when you said, if you put all your issues into that bucket related to data quality, now imagine all those issues went away, come back a year later and all those issues are going to be back again because you didn't solve the root problem." And so, if you really want to address data quality in a real way, you have to solve the root cause. And you really have to talk to people. You got to work with people, understand them, understand the business, and really think about data customers and data creators. Those are the two personas or those two roles in the organization. And a ton of people are actually in the business. They are the ones who are creating the data. They are not supported, they're not trained. 95% of the people are doing data management are in those roles that are untrained. And that's a huge problem. And Juan, I'll pass it over to you.
00:58:31 Juan Sequeda
Well, we were talking about A, bad data, is that actually a thing or not? You're like, " Yes, there is. If I keep you to the wrong address and I can't ship anything to it, because it doesn't even exist, that's bad data." But we are discussing this spectrum of data issues. You can have 100 issues, 80% can probably be the easy things to go solve. Right? And where easy means there's a clear right or wrong. And, maybe that 20% will have those deep, logical, philosophical issues. But those are the ones that are costly, right? So, another thing you said, folks, when we talk about data quality, they're so narrow routed. So it's not just about accuracy, but also think about the relevance around things. And, it goes back to if it's right or wrong, that's a test to solve if it's an easy thing. And if you don't know if it's right or wrong, then it's complicated, then that means you need the context and you need the relevance, you go talk to people around that. So people, people, people, people is a big theme here. Most people aren't data scientists, right? They're the people who just run the store. So I think that first observation you had, part of your book, is that, the vast majority of data management is being done by people without data in their titles. They work in marketing, the ops and finance, right? And they're doing the work of confirming the data. They're untrained, they're unsupported, they're just trying to get their job done. And the second observation that the data scientists of the whole data teams, they'll properly understand the business, get the business problems they're working on, unless they understand what is going on with the regular people involving in those decisions. This is the miscommunication that we have. So we need to bring in the regular people. And I think we both share this sentiment that data literacy is a term that needs to go, because they're not illiterate, right? They're uninformed. So, we want you to become a better data customer and a data creator, because everybody is a data customer and everybody's a data creator. And, I think, we're talking, if it's not data literacy training workshops, then it's about how to improve the quality, how to understand your decisions, what are your business processes? Those are the types of workshops that we should be having. And so, who does this work? You're talking about, in your examples, a customer at a bank, they had over 100 ambassadors. It's a part- time of their role. You get world crisis training around this. It's part of the CDO office to put those training, where you have all this face- to- face. You end up working with the HR to figure out how to go scale this, because they have a bigger budget. So, running the business is harder than the data scientist and the analysts give credit, right? Running the store is hard, and it's critical. And this is why you need to go learn around that, be it that business literacy we're arguing. And I think the closing sentence here that summarizes everything is, most data management is done by regular people without training and without support.
01:01:14 Tom Redmond
Right. And just put a capstone on it. And, it may not stand. It is time for that to go away.
01:01:22 Juan Sequeda
How did we do? Anything we missed on takeaways?
01:01:25 Tom Redmond
No, I think we did really, really great. I mean, I will add, one other thing we didn't really talk much about leadership, and we didn't talk about courage. Right? But, these changes, the people who've done these things before, their leadership was involved. And they were leaders themselves, and they were working with leadership across the organization that they were dealing with. And the last thing is, is any change, I don't care how small it is, requires courage. So, breaking out from doing this to doing that, it is an act of courage.
01:02:03 Tim Gasper
I think that's really important to note that, because I think it's easy sometimes to, especially when we talk about data quality, get really obsessed with some of the minutia, some of the detail. Sometimes you got to remember, leadership, courage, change requires leadership, and that's a necessary ingredient.
01:02:19 Juan Sequeda
So to wrap us up quickly here, advice? Who should we invite next? And what resources do you follow?
01:02:27 Tom Redmond
Well, so I'll answer those in reverse order. Look, I read everything. Right? I mean, I cast a wide net. Right? So, things from, In the Business World from Michael Porter and Peter Drucker. And it's like, " Why does a data guy know about productivity?" Right? So some economics in there. I study but not view myself as a expert in change management. And, by the way, I mean, I am a voracious reader, and I try to learn things and integrate them into the way I'm thinking about things. I do my best to give credit to others who have contributed to my thinking.
01:03:12 Juan Sequeda
So, read everything.
01:03:13 Tom Redmond
Read.
01:03:14 Juan Sequeda
All right, so who should we invite next?
01:03:16 Tom Redmond
So, look, I think that there's a great list of people who've written with me in the past couple of years. But, if you really want to focus on catalogs, then I had a little study group consisting of Dave Hay, John Zackman, and Lua inaudible. Okay? And, as I mentioned John and John's framework, and you looked it up and said, " Gee, this would be a great thing to learn." Another guy I work a lot with is Tom Davenport, the chief data Officer at Gulf Bank would be a great person to have, right? Some people in the data management community like John Ladley are struggling, " How do we make this stuff more relevant and better?" So, there's more candidates.
01:04:08 Juan Sequeda
Yeah. I mean, I love that. I'm excited to chatting with Tom Davenport. So, having him soon.
01:04:13 Tom Redmond
Yeah.
01:04:13 Juan Sequeda
So finally, to wrap us up, take us away with your advice.
01:04:16 Tom Redmond
Yeah. My advice is just have courage. I mean, go after this, right? We're at the equivalent of day one in the data space, and it's easy to think you're behind, because you don't know technology. You don't know this. You don't know that. But I don't care who you are, you can make a mark within your team, within your division, within your company, and you can do it now. Right? And, it's a knowledge about data. Well, that's in short supply, but what's in even shorter supply is courage. And so, have the courage to get yourself out there.
01:04:52 Juan Sequeda
And with that, I love this courage, and a last question that came up here was, " I need business literacy class. Where can I learn or unlearn?" I'm like, you know what? I think, Anna, this is the opportunity to be courageous and just go meet the people in your business and the people who are running the store and just ask them, " Hey, how do you run your business? How do you run that store?" I think that's the way to start. Just all these people are around you, just have the courage and go talk.
01:05:18 Tom Redmond
Yeah. I mean, let me build on that, go read your annual report. Okay? There's a lot of stuff in there.
01:05:22 Juan Sequeda
Yeah. If they're a public company, go read the annual report and everything.
01:05:25 Tom Redmond
Right. Right. I mean, even private companies have some report like that. So, you don't have to bother anybody to do that.
01:05:32 Juan Sequeda
And with that, Tom, this is a great way. Cheers. Thank you so much. Tim.
01:05:36 Tom Redmond
Thank you.
01:05:37 Juan Sequeda
We're off.
01:05:37 Tim Gasper
Cheers, Tom.
01:05:38 Juan Sequeda
We have our honest no- BS data dinner. Cheers, everybody. Thank you.
Special guests
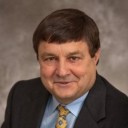